Physical-Layer Security Enhancement in Energy-Harvesting-Based Cognitive Internet of Things: A GAN-Powered Deep Reinforcement Learning Approach
IEEE INTERNET OF THINGS JOURNAL(2024)
摘要
Cognitive radio (CR) is regarded as the key technology of the 6th-Generation (6G) wireless network. Because 6G CR networks are anticipated to offer worldwide coverage, increase cost efficiency, enhance spectrum utilization, and improve device intelligence and network safety. This article studies the secrecy communication in an energy-harvesting (EH)-enabled Cognitive Internet of Things (EH-CIoT) network with a cooperative jammer. The secondary transmitters (STs) and the jammer first harvest the energy from the received radio frequency (RF) signals in the EH phase. Then, in the subsequent wireless information transfer (WIT) phase, the STs transmit secrecy information to their intended receivers in the presence of eavesdroppers while the jammer sends the jamming signal to confuse the eavesdroppers. To evaluate the system secrecy performance, we derive the instantaneous secrecy rate and the closed-form expression of secrecy outage probability (SOP). Furthermore, we propose a deep reinforcement learning (DRL)-based framework for the joint EH time and transmission power allocation problems. Specifically, a pair of ST and jammer over each time block is modeled as an agent which is dynamically interacting with the environment by the state, action, and reward mechanisms. To better find the optimal solutions to the proposed problems, the long short-term memory (LSTM) network and the generative adversarial networks (GANs) are combined with the classical DRL algorithm. The simulation results show that our proposed method is highly effective in maximizing the secrecy rate while minimizing the SOP compared with other existing schemes.
更多查看译文
关键词
Jamming,Internet of Things,Wireless communication,Resource management,Communication system security,6G mobile communication,Mobile handsets,Cognitive radio (CR) network,deep reinforcement learning (DRL),energy harvesting (EH),physical-layer security (PLS) enhancement
AI 理解论文
溯源树
样例
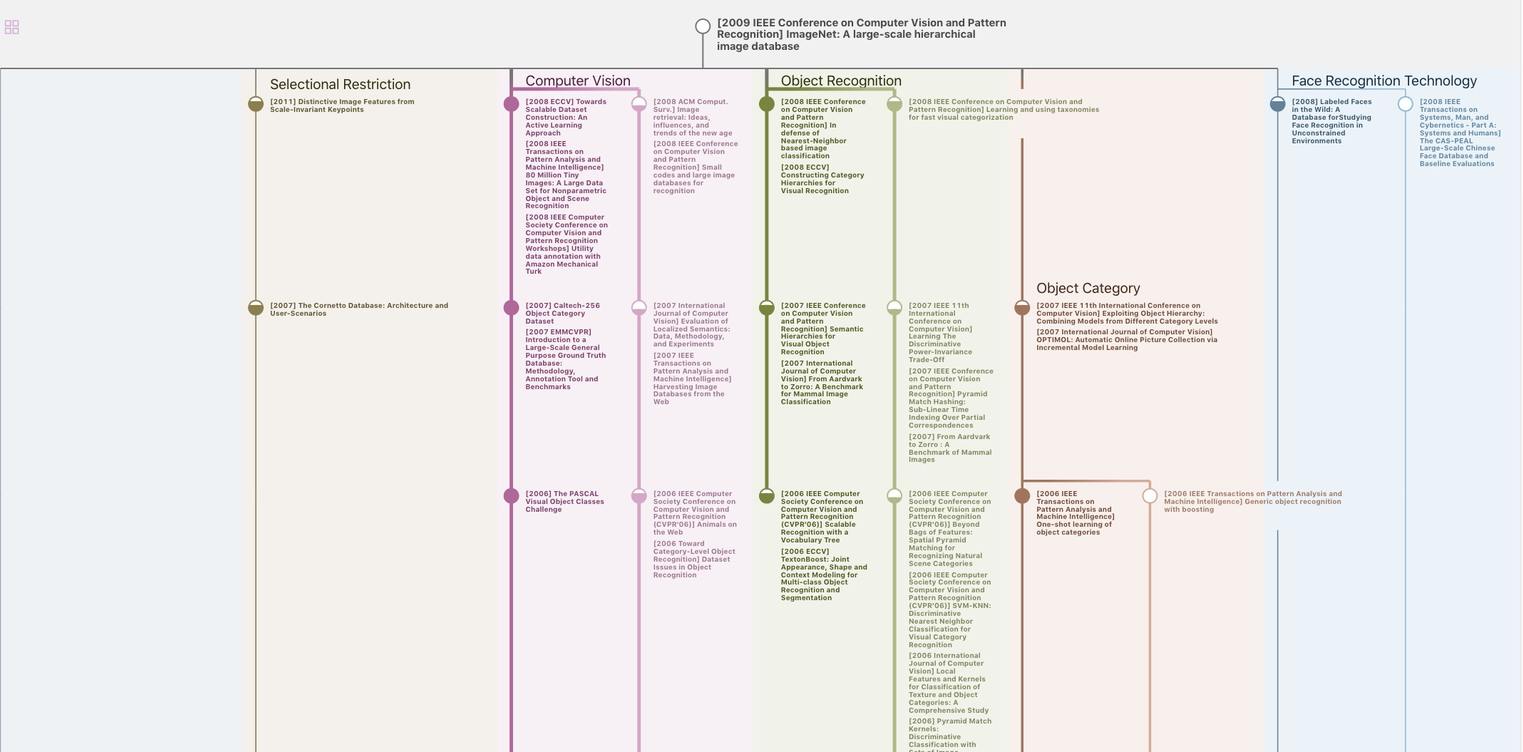
生成溯源树,研究论文发展脉络
Chat Paper
正在生成论文摘要