Semi and Self-supervised Learning for Multi-label Classification for an Underwater Inspection Imagery Application
Research Square (Research Square)(2023)
摘要
Abstract Underwater inspections are crucial for the preventive maintenance of offshore equipment from the oil and gas industry. However, the entire inspection process is costly, subjective and time-consuming. Traditionally, specialists assess equipment conditions through image and sensor data collected by underwater vehicles that travel to the seabed. Since this data can present a wide range of events, an inspection can yield a considerable amount of data. Despite being a tedious task, this data is undoubtedly domain-specific, requiring a group of highly specialized professionals to correctly scrutinize it. In this scenario, we propose using image classification models to help specialists find event(s) of interest. However, there are challenges inherent to the underwater inspection image classification problem, such as: high cost and scarcity of balanced labeled data, presence of label noise, high intra-class variance and characteristics of underwater images such as turbidity and uneven lightning conditions. Therefore, traditional supervised models might not fulfill the task. We undertake this problem with the methods DINO (Self-DIstillation with NO labels, self-supervised) and a new proposed method mPAWS, a multi-label version of PAWS (Predicting View Assignments With Support Samples, semi-supervised). The results obtained show the benefits of using such models for the application, achieving an improvement of $2.7\%$ when compared to current state-of-the-art supervised models. Such models can perform real-time inference, which can help accelerate the tasks of the underwater vehicle, making the work of the specialists more time-efficient.
更多查看译文
关键词
classification,learning,self-supervised,multi-label
AI 理解论文
溯源树
样例
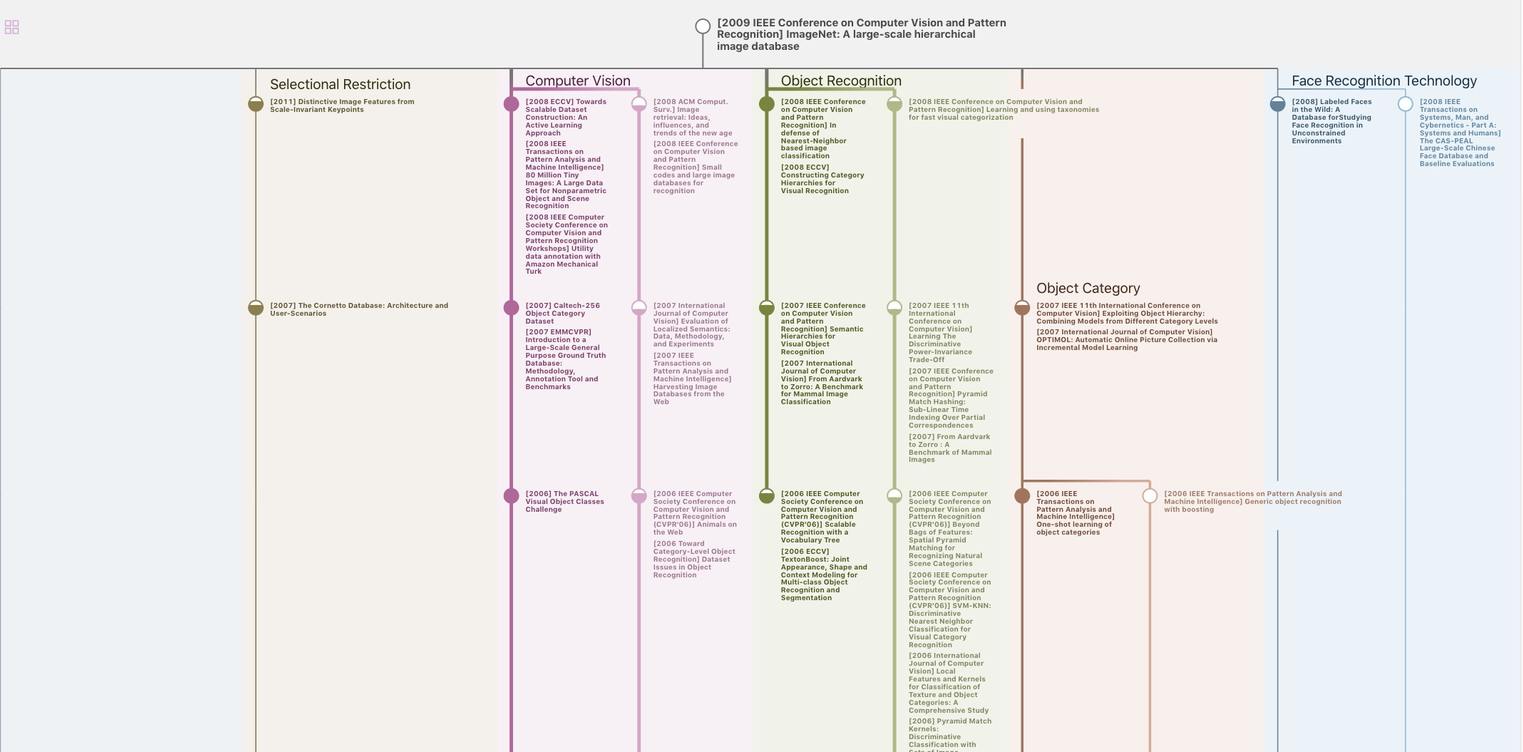
生成溯源树,研究论文发展脉络
Chat Paper
正在生成论文摘要