Retrieving Texts based on Abstract Descriptions
arXiv (Cornell University)(2023)
摘要
While instruction-tuned Large Language Models (LLMs) excel at extracting information from text, they are not suitable for locating texts conforming to a given description in a large document collection (semantic retrieval). Similarity search over embedding vectors does allow to perform retrieval by query, but the similarity reflected in the embedding is ill-defined and non-consistent, and is sub-optimal for many use cases. What, then, is a good query representation for effective retrieval? We identify the well defined and consistent task of retrieving sentences based on abstract descriptions of their content. We demonstrate the inadequacy of current text embeddings and propose an alternative model that significantly improves when used in standard nearest neighbor search. The model is trained using positive and negative pairs sourced through prompting a LLM. While it is easy to source the training material from an LLM, the retrieval task cannot be performed by the LLM directly. This demonstrates that data from LLMs can be used not only for distilling more efficient specialized models than the original LLM, but also for creating new capabilities not immediately possible using the original model.
更多查看译文
关键词
abstract descriptions,texts
AI 理解论文
溯源树
样例
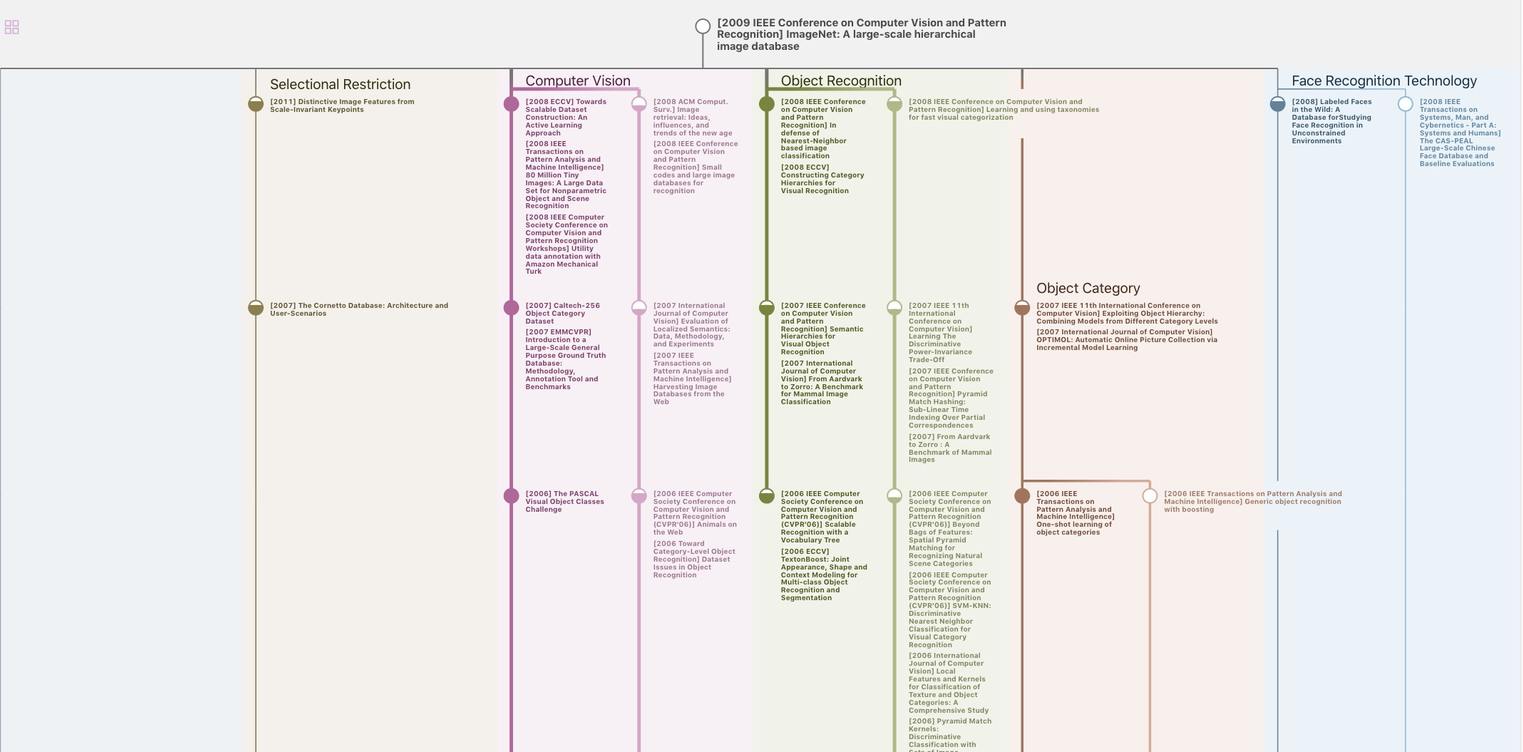
生成溯源树,研究论文发展脉络
Chat Paper
正在生成论文摘要