Privacy-Aware Energy Consumption Modeling of Connected Battery Electric Vehicles using Federated Learning
IEEE Transactions on Transportation Electrification(2023)
摘要
Battery Electric Vehicles (BEVs) are increasingly significant in modern
cities due to their potential to reduce air pollution. Precise and real-time
estimation of energy consumption for them is imperative for effective itinerary
planning and optimizing vehicle systems, which can reduce driving range anxiety
and decrease energy costs. As public awareness of data privacy increases,
adopting approaches that safeguard data privacy in the context of BEV energy
consumption modeling is crucial. Federated Learning (FL) is a promising
solution mitigating the risk of exposing sensitive information to third parties
by allowing local data to remain on devices and only sharing model updates with
a central server. Our work investigates the potential of using FL methods, such
as FedAvg, and FedPer, to improve BEV energy consumption prediction while
maintaining user privacy. We conducted experiments using data from 10 BEVs
under simulated real-world driving conditions. Our results demonstrate that the
FedAvg-LSTM model achieved a reduction of up to 67.84\% in the MAE value of the
prediction results. Furthermore, we explored various real-world scenarios and
discussed how FL methods can be employed in those cases. Our findings show that
FL methods can effectively improve the performance of BEV energy consumption
prediction while maintaining user privacy.
更多查看译文
关键词
Federated Learning,Electric Vehicles,Energy Consumption Modelling,Edge-Cloud Computing,Digital Twin,Privacy Awareness
AI 理解论文
溯源树
样例
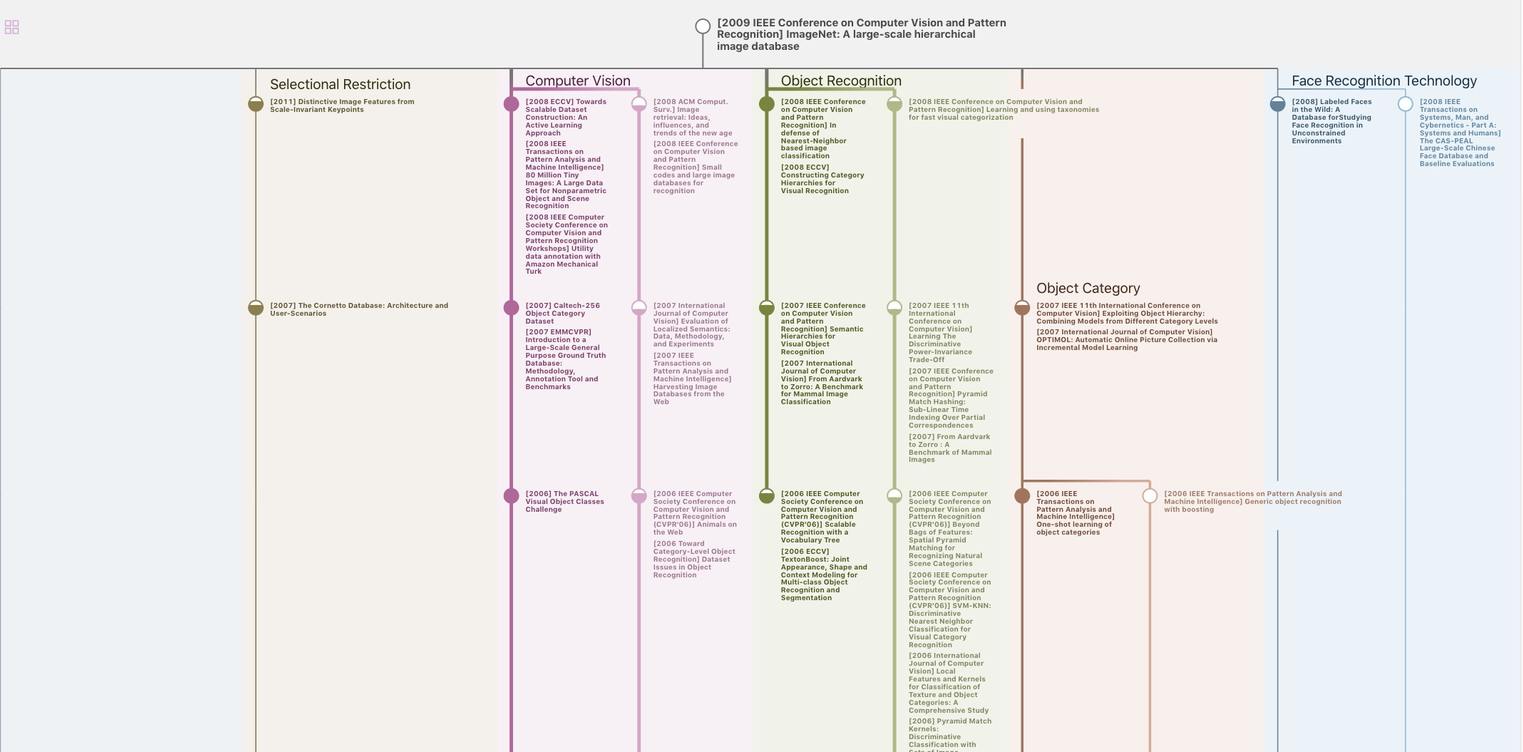
生成溯源树,研究论文发展脉络
Chat Paper
正在生成论文摘要