Understanding Physical Dynamics with Counterfactual World Modeling
arxiv(2023)
Abstract
The ability to understand physical dynamics is critical for agents to act in
the world. Here, we use Counterfactual World Modeling (CWM) to extract vision
structures for dynamics understanding. CWM uses a temporally-factored masking
policy for masked prediction of video data without annotations. This policy
enables highly effective "counterfactual prompting" of the predictor, allowing
a spectrum of visual structures to be extracted from a single pre-trained
predictor without finetuning on annotated datasets. We demonstrate that these
structures are useful for physical dynamics understanding, allowing CWM to
achieve the state-of-the-art performance on the Physion benchmark.
MoreTranslated text
AI Read Science
Must-Reading Tree
Example
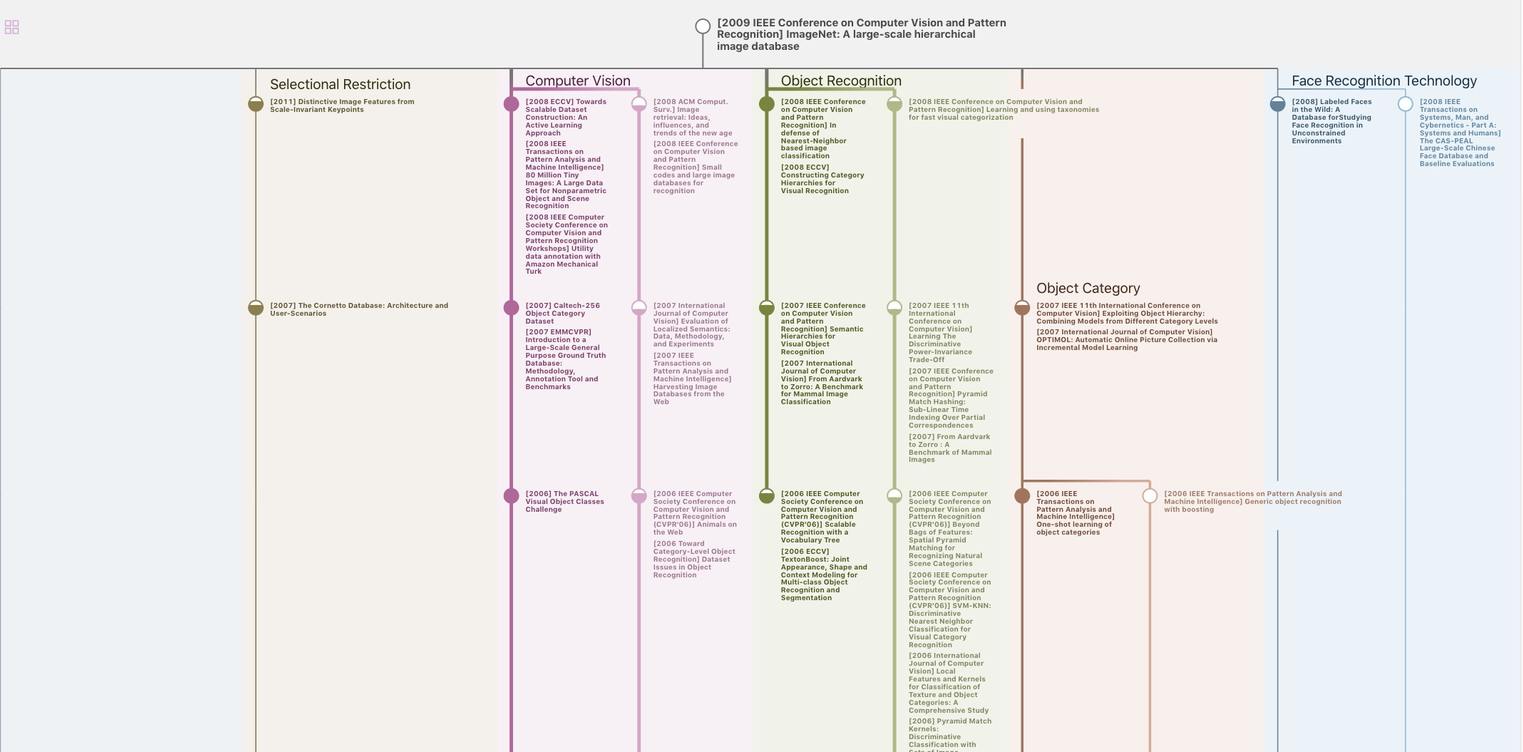
Generate MRT to find the research sequence of this paper
Chat Paper
Summary is being generated by the instructions you defined