Knowledge Distillation-based Domain-invariant Representation Learning for Domain Generalization
IEEE Transactions on Multimedia(2023)
摘要
Domain generalization (DG) aims to generalize the knowledge learned from multiple source domains to unseen target domains. Existing DG techniques can be subsumed under two broad categories, i.e., domain-invariant representation learning and domain manipulation. Nevertheless, it is extremely difficult to explicitly augment or generate the unseen target data. And when source domain variety increases, developing a domain-invariant model by simply aligning more domain-specific information becomes more challenging. In this paper, we propose a simple yet effective method for domain generalization, named Knowledge Distillation based Domain-invariant Representation Learning (KDDRL), that learns domain-invariant representation while encouraging the model to maintain domain-specific features, which recently turned out to be effective for domain generalization. To this end, our method incorporates multiple auxiliary student models and one student leader model to perform a two-stage distillation. In the first-stage distillation, each domain-specific auxiliary student treats the ensemble of other auxiliary students' predictions as a target, which helps to excavate the domain-invariant representation. Also, we present an error removal module to prevent the transfer of faulty information by eliminating incorrect predictions compared to the true labels. In the second-stage distillation, the student leader model with domain-specific features combines the domain-invariant representation learned from the group of auxiliary students to make the final prediction. Extensive experiments and in-depth analysis on popular DG benchmark datasets demonstrate that our KDDRL significantly outperforms the current state-of-the-art methods.
更多查看译文
关键词
Domain generalization,knowledge distillation,domain invariant representation
AI 理解论文
溯源树
样例
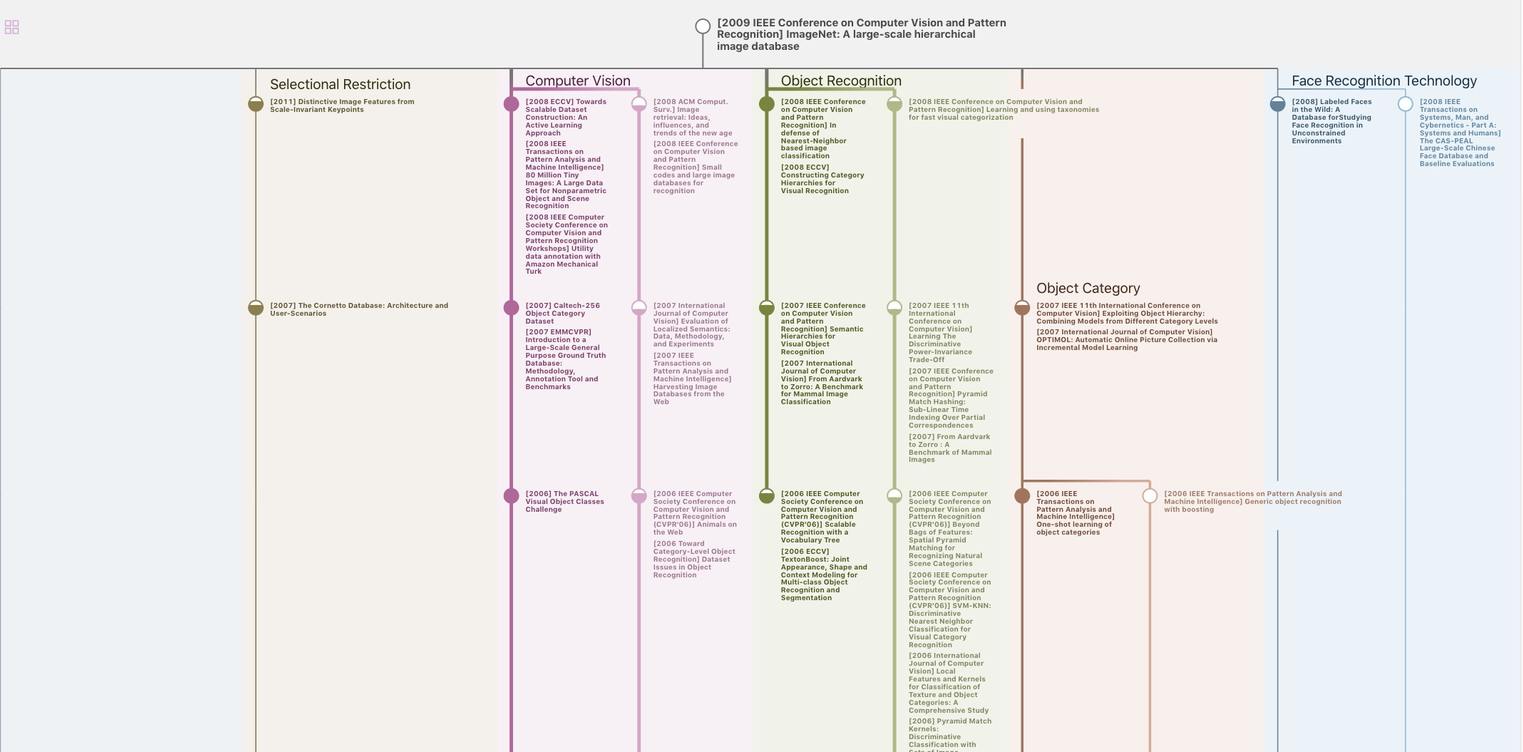
生成溯源树,研究论文发展脉络
Chat Paper
正在生成论文摘要