MyVoice: Arabic Speech Resource Collaboration Platform
INTERSPEECH 2023(2023)
Abstract
We introduce MyVoice, a crowdsourcing platform designed to collect Arabic speech to enhance dialectal speech technologies. This platform offers an opportunity to design large dialectal speech datasets; and makes them publicly available. MyVoice allows contributors to select city/country-level fine-grained dialect and record the displayed utterances. Users can switch roles between contributors and annotators. The platform incorporates a quality assurance system that filters out low-quality and spurious recordings before sending them for validation. During the validation phase, contributors can assess the quality of recordings, annotate them, and provide feedback which is then reviewed by administrators. Furthermore, the platform offers flexibility to admin roles to add new data or tasks beyond dialectal speech and word collection, which are displayed to contributors. Thus, enabling collaborative efforts in gathering diverse and large Arabic speech data.
MoreTranslated text
Key words
data collection,multi-dialect Arabic,speech recognition
PDF
View via Publisher
AI Read Science
AI Summary
AI Summary is the key point extracted automatically understanding the full text of the paper, including the background, methods, results, conclusions, icons and other key content, so that you can get the outline of the paper at a glance.
Example
Background
Key content
Introduction
Methods
Results
Related work
Fund
Key content
- Pretraining has recently greatly promoted the development of natural language processing (NLP)
- We show that M6 outperforms the baselines in multimodal downstream tasks, and the large M6 with 10 parameters can reach a better performance
- We propose a method called M6 that is able to process information of multiple modalities and perform both single-modal and cross-modal understanding and generation
- The model is scaled to large model with 10 billion parameters with sophisticated deployment, and the 10 -parameter M6-large is the largest pretrained model in Chinese
- Experimental results show that our proposed M6 outperforms the baseline in a number of downstream tasks concerning both single modality and multiple modalities We will continue the pretraining of extremely large models by increasing data to explore the limit of its performance
Try using models to generate summary,it takes about 60s
Must-Reading Tree
Example
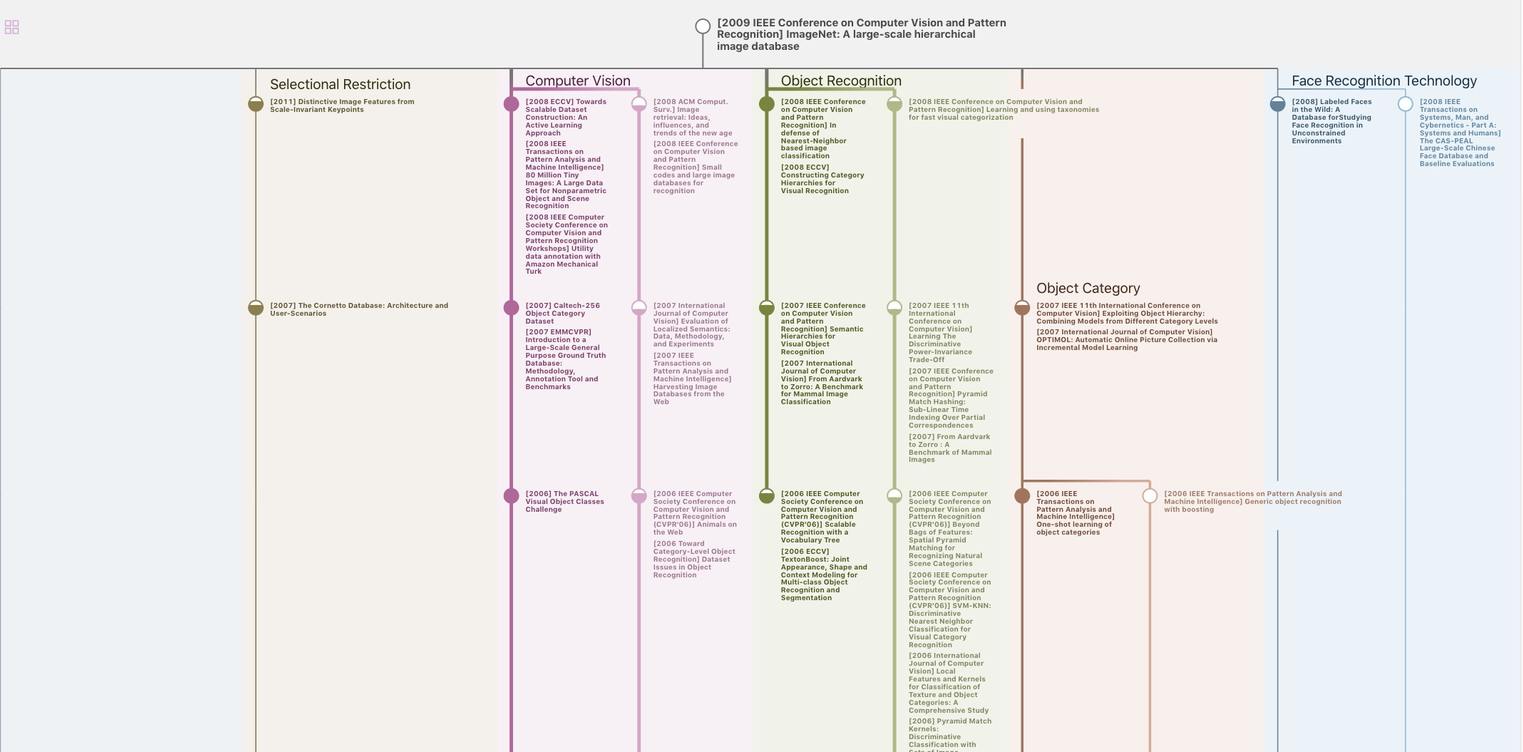
Generate MRT to find the research sequence of this paper
Related Papers
2020
被引用173 | 浏览
Data Disclaimer
The page data are from open Internet sources, cooperative publishers and automatic analysis results through AI technology. We do not make any commitments and guarantees for the validity, accuracy, correctness, reliability, completeness and timeliness of the page data. If you have any questions, please contact us by email: report@aminer.cn
Chat Paper
GPU is busy, summary generation fails
Rerequest