Improving the Accuracy and Efficiency of Compression-based Dissimilarity Measure using Information Quantity in Data Classification Problems
Transactions of The Japanese Society for Artificial Intelligence(2023)
摘要
Compression-based Dissimilarity Measure (CDM) is reported to work well in classifying strings without clues. However, CDM depends on the compression program, and its theoretical background is unclear. In this paper, we propose to replace CDM with the computation of information quantity. Since CDM only uses compressed size, our approach uses the value of information quantity of maximum probability partitioning of string instead of file size. We find this approach is more effective. Then, CDM and the proposed method were applied to publicly available time series data. In addition to the careful implementation of computation using suffix arrays, we also find this approach more efficient.
更多查看译文
关键词
dissimilarity measure,information quantity,classification,data,compression-based
AI 理解论文
溯源树
样例
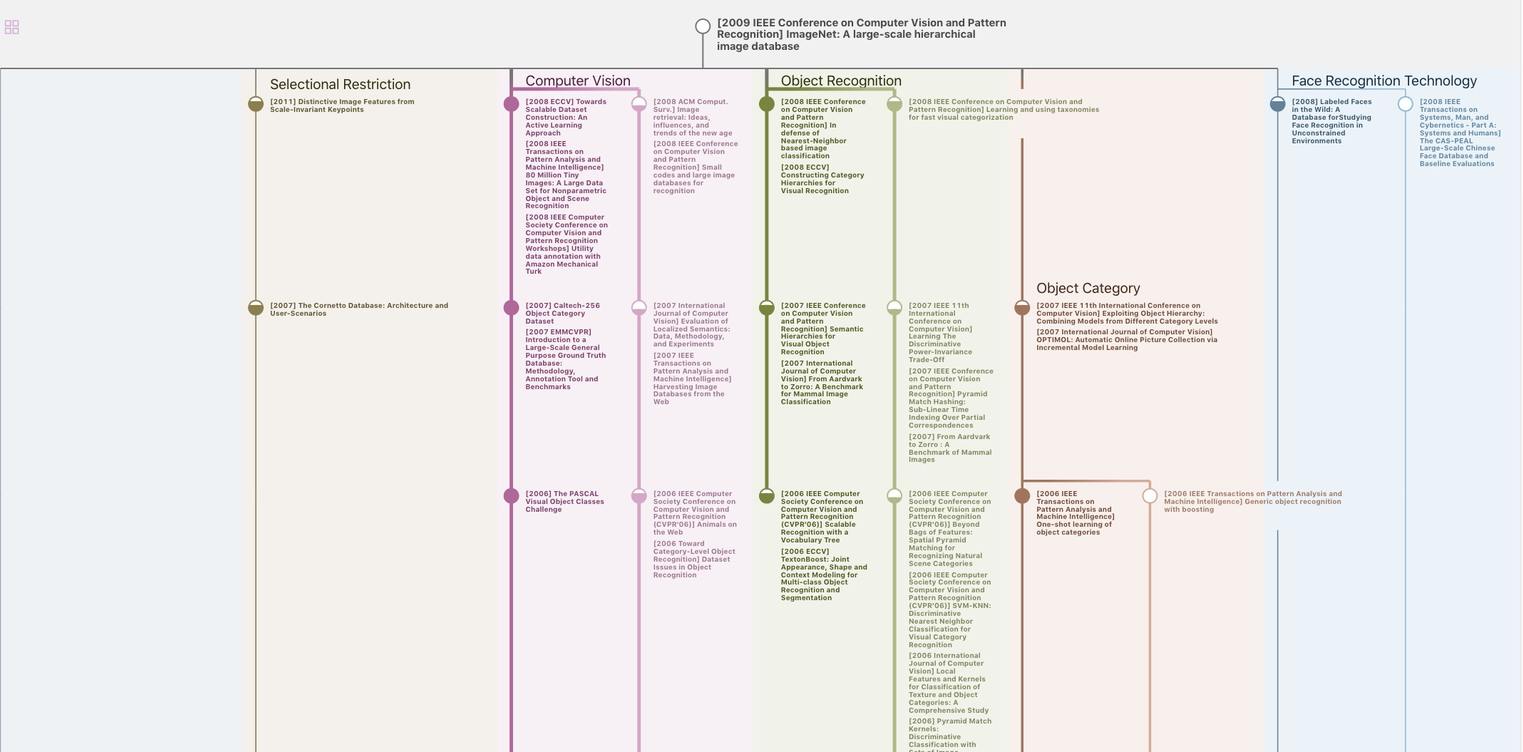
生成溯源树,研究论文发展脉络
Chat Paper
正在生成论文摘要