Evaluation of computer vision for detecting agonistic behavior of pigs in a single-space feeding stall through blocked cross-validation strategies
Computers and Electronics in Agriculture(2023)
摘要
Agonistic behavior at feeding spaces is associated with both welfare and feed intake issues in swine farming. Studying interactive social behaviors of group-housed pigs provides valuable information to improve their production and welfare. The aims of this study were to (1) develop a deep learning pipeline based on convolutional neural network (CNN) and long short-term memory (LSTM) to classify videos depicting four types of interactive behavior between pigs in a single-space feeding stall and (2) validate the CNN + LSTM pipeline through various blocked validation strategies. Four categories of behaviors were classified in this study: head-to-body contact (including gentle nosing, casual contact between head/ears of a pig with a feeding pig, head knocking, tail biting, and pushing); levering where the feeding pig was lifted from behind by another pig; mounting in which the feeding pig was mounted by another pig; and no-contact when a second pig entered the feeding stall without physical contact with the feeding pig. Behavior at the feeding stall was filmed twice, three weeks apart, for two consecutive days each week using six groups of grow-finish pigs (10 per group) housed in pens equipped with FIRE® feeders. This resulted in a total of 15,679 30-frame video episodes for classification. Random validation, blocking-by-time validation, and blocking-by-feeder validation were utilized for training-validation data split. The size of training sets was held constant (N = 7,500) through all validation scenarios. The average validation accuracies were 0.968(±0.001), 0.860(±0.033), 0.766(±0.026), and 0.860(±0.010) for random validation, blocking-by-time validation, and blocking-by-feeder validation (at Feeder 1 and at Feeder 2), respectively. The results indicate that the CNN + LSTM pipeline yielded acceptable predictive performance in random validation. However, performance was substantially worse in blocking-by-time and blocking-by-feeder validations. More work is needed for algorithm generalization to improve its robustness across a variety of real-life application scenarios. We provide public access to the dataset and the code.
更多查看译文
关键词
pigs,agonistic behavior,computer vision,single-space,cross-validation
AI 理解论文
溯源树
样例
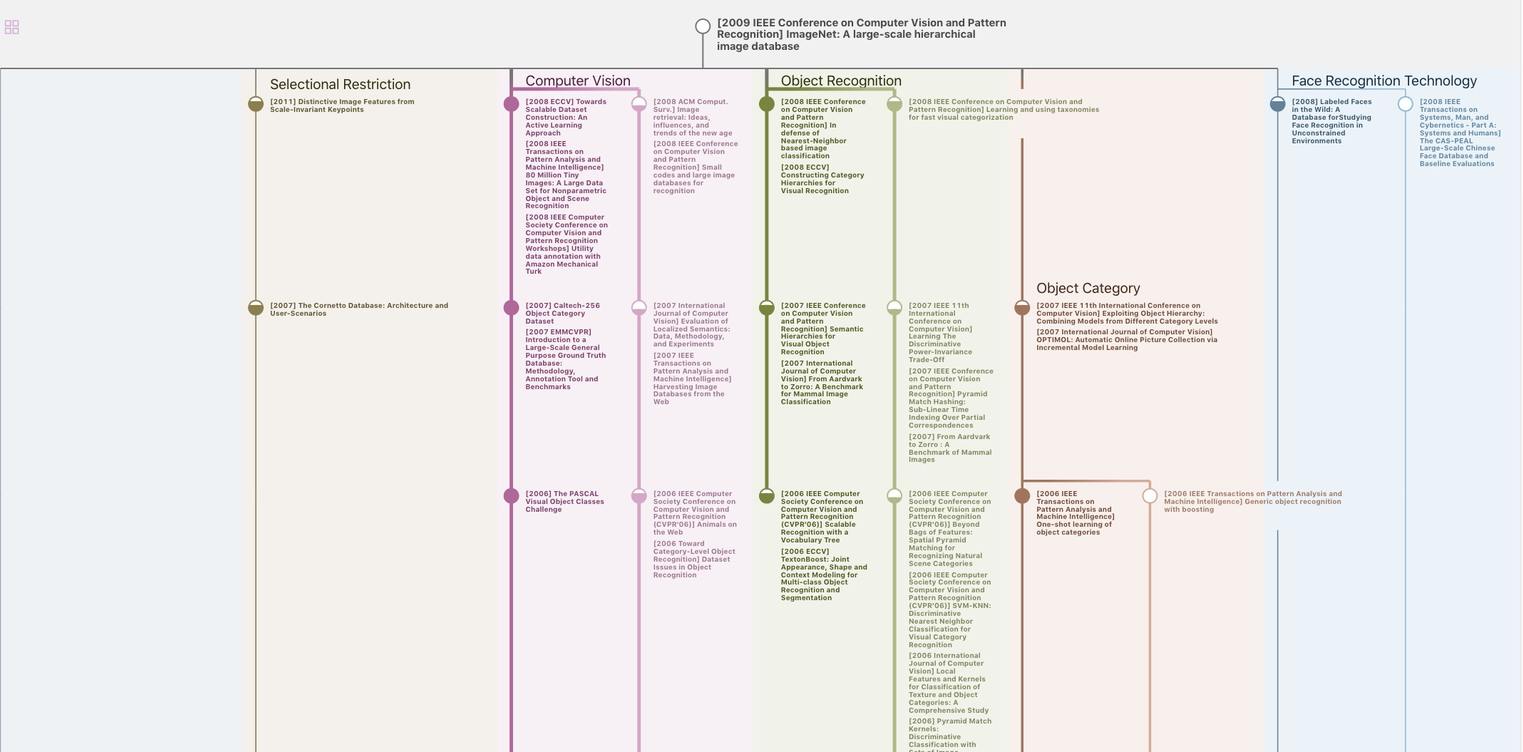
生成溯源树,研究论文发展脉络
Chat Paper
正在生成论文摘要