Distributionally Robust Infinite-horizon Control: from a pool of samples to the design of dependable controllers
CoRR(2023)
摘要
We study control of constrained linear systems when faced with only partial statistical information about the disturbance processes affecting the system dynamics and the sensor measurements. Specifically, given a finite collection of disturbance realizations, we consider the problem of designing a stabilizing control policy with provable safety and performance guarantees in face of the inevitable mismatch between the true and the empirical distributions. We capture this discrepancy using Wasserstein ambiguity sets, and we formulate a distributionally robust (DR) optimal control problem, which provides guarantees on the expected cost, safety, and stability of the system. To solve this problem, we first present new results for DR optimization of quadratic objectives using convex programming, showing that strong duality holds under mild conditions. Then, by combining our results with the system level parametrization (SLP) of linear feedback policies, we show that the design problem can be reduced to a semidefinite optimization problem (SDP).
更多查看译文
AI 理解论文
溯源树
样例
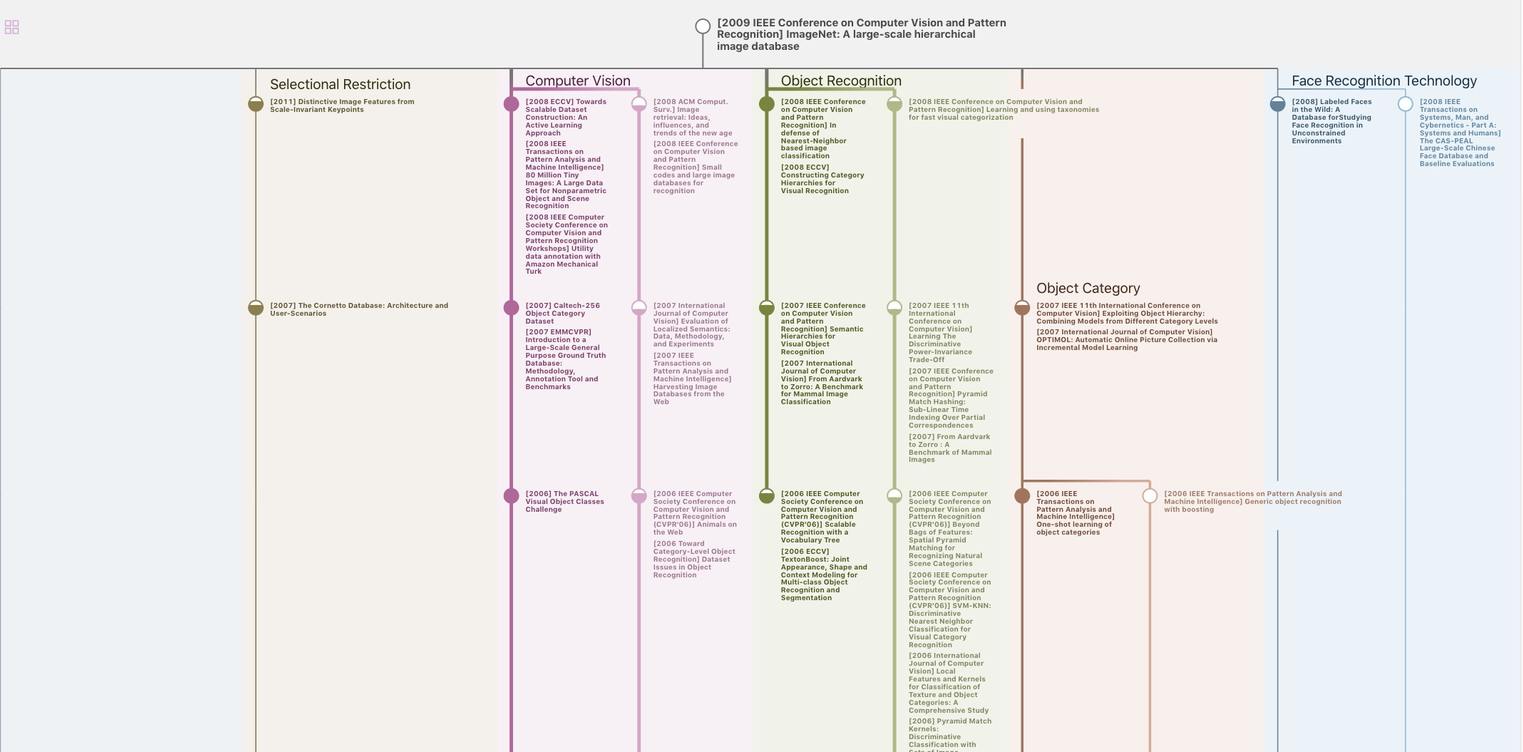
生成溯源树,研究论文发展脉络
Chat Paper
正在生成论文摘要