CFL-IDS: An Effective Clustered Federated Learning Framework for Industrial Internet of Things Intrusion Detection
IEEE INTERNET OF THINGS JOURNAL(2024)
摘要
The Industrial Internet of Things (IIoT) offers the manufacturing sector opportunities for transformation and upgrade but also carries significant security risks. Traditional federated learning (FL) as a potential security solution is challenging in complicated application environments with heterogeneous data, imbalanced data, and poisoning attacks. To address these challenges, we construct a clustered FL Framework for IIoT intrusion detection (CFL-IDS) based on local models' evaluation metrics (EMs). First, we designed an intrusion detection model with a dynamic focal loss (DFL) for all edge nodes (ENs). This model's performance is enhanced under various imbalanced data partitions by dynamically altering the focus on samples during the loss minimization training process. Second, the time series of EMs of local models to reflect the data distribution of ENs implicitly, and use clustering algorithms to facilitate knowledge sharing among those ENs with similar data distribution to co-optimize a common model for them. Finally, an intelligent cooperative model aggregation mechanism (ICMAM) adaptively adjusts each local model's weight distribution, which substantially improves the benefits of FL and alleviates subpar models' alleviates interference from subpar models to FL. Experiments demonstrate that CFL-IDS has stronger robustness and displays superior performance under data imbalance and non-independent and identically distributed (non-IID) situations while being effective against poisoning attacks.
更多查看译文
关键词
Clustered federated learning (FL),data imbalanced,evaluation metrics (EMs),Industrial Internet of Things (IIoT) Intrusion detection,non-independent and identically distributed (non-IID),poisoning attack
AI 理解论文
溯源树
样例
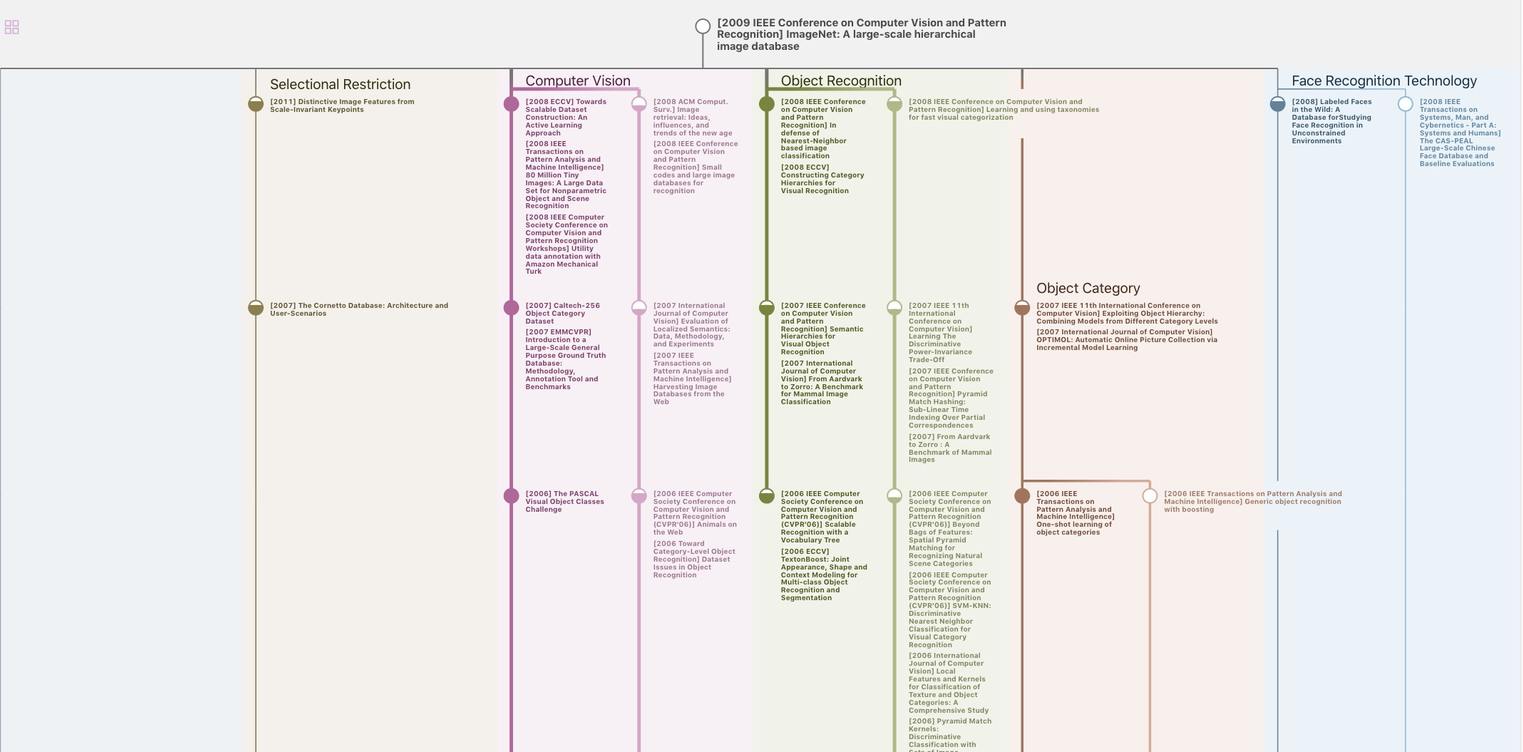
生成溯源树,研究论文发展脉络
Chat Paper
正在生成论文摘要