State of Charge Estimation of Lithium-ion Batteries using Physics-informed Transformer for Limited Data Scenarios
ASME letters in dynamic systems and control(2023)
摘要
Abstract Accurate estimation of the state of charge (SOC) is crucial for ensuring the safe and efficient operation of lithium-ion batteries. Machine learning (ML) models may achieve high SOC estimation accuracy, but typically require large training datasets that may not always be accessible in practical applications. To address this issue, this work proposes a hybrid model consisting of a Transformer neural network and a single particle model with electrolyte dynamics (SPMe) for SOC estimation in limited data scenarios. The Transformer can leverage the internal battery states estimated by the SPMe when necessary and learn to use information from multiple sources (i.e., experimental data and SPMe). Two limited data scenarios, partially available cycles and varying temperatures, are evaluated with experimental battery discharge cycles to identify the conditions under which the proposed model outperforms traditional ML models. Despite being highly dependent on the SPMe's performance, the hybrid model demonstrated improved SOC estimation over the baseline models, with less than 2% error for most scenarios.
更多查看译文
关键词
charge estimation,transformer,lithium-ion,physics-informed
AI 理解论文
溯源树
样例
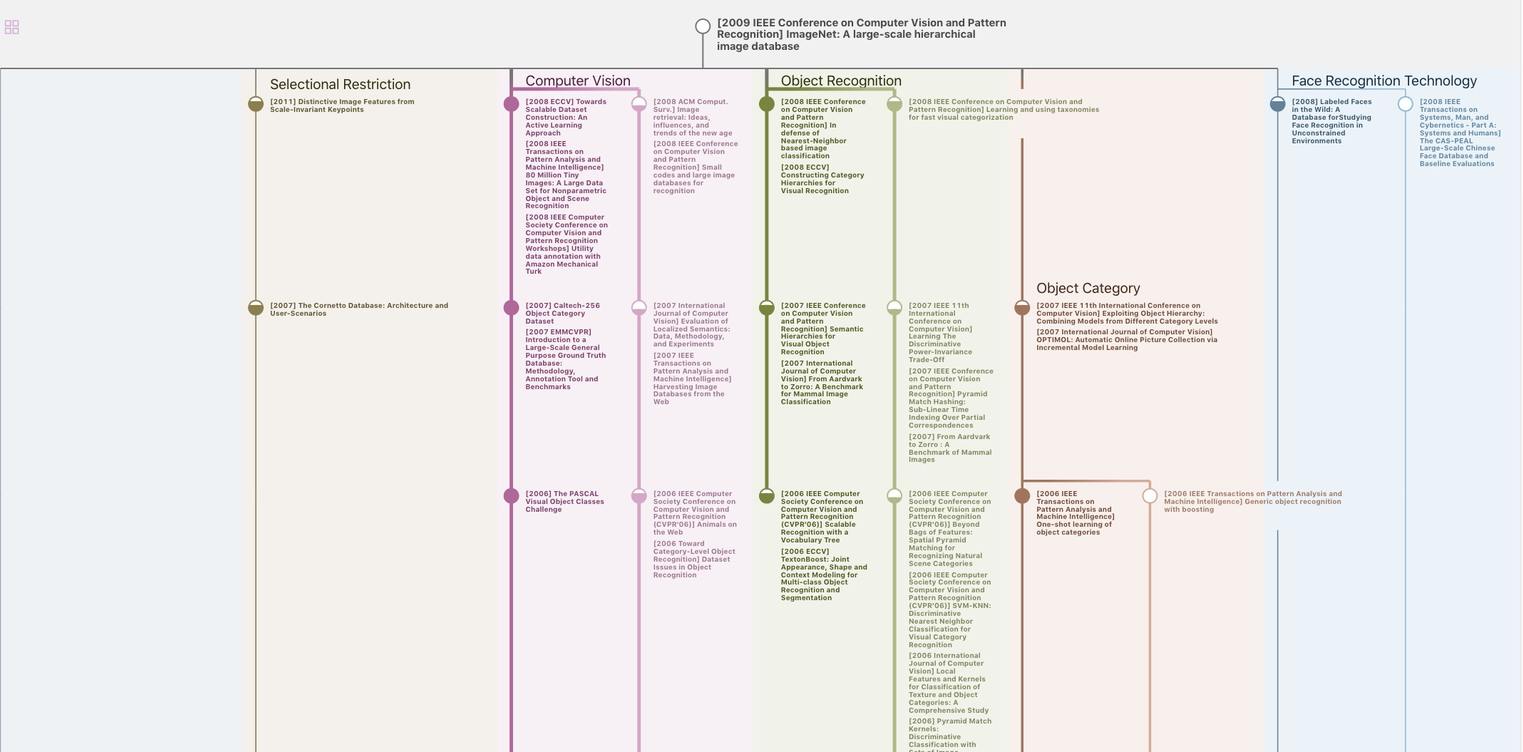
生成溯源树,研究论文发展脉络
Chat Paper
正在生成论文摘要