Machine Learning-Based Calibration Approaches for Single-Beam and Multiple-Beam Distance Sensors
IEEE SENSORS JOURNAL(2024)
摘要
Sensors are the foundation to facilitate smart cities, smart grids, and smart transportation, and distance sensors are especially important for sensing the environment and gathering information. Researchers have developed various methods to calibrate distance sensors; however, they mainly focus on using external information for calibration, which is inefficient and computationally expensive. To alleviate the above problems, we propose machine learning (ML)-based calibration approaches for distance sensors. We develop two calibration mechanisms, where the naive mechanism directly predicts the true distance, while the residual mechanism predicts the residual for calibration. The conventional ML regression algorithms are then adopted to predict the true distance or calibration residual. Experimental results show that the designed residual calibration mechanism outperforms the naive mechanism by reducing the root mean square error (RMSE) by 92.2% and mean absolute error (MAE) by 93.3% in the fitting task. In prediction, the proposed 2-D-residual mechanism achieves an improvement of 78.6% and 65.7% compared to the state-of-the-art method. The experimental results show the effectiveness and generalization of the proposed ML-based calibration method, especially for the 2-D mechanism, which achieves the highest robustness in all fitting and prediction tasks.
更多查看译文
关键词
Calibration,distance sensors,machine learning (ML)
AI 理解论文
溯源树
样例
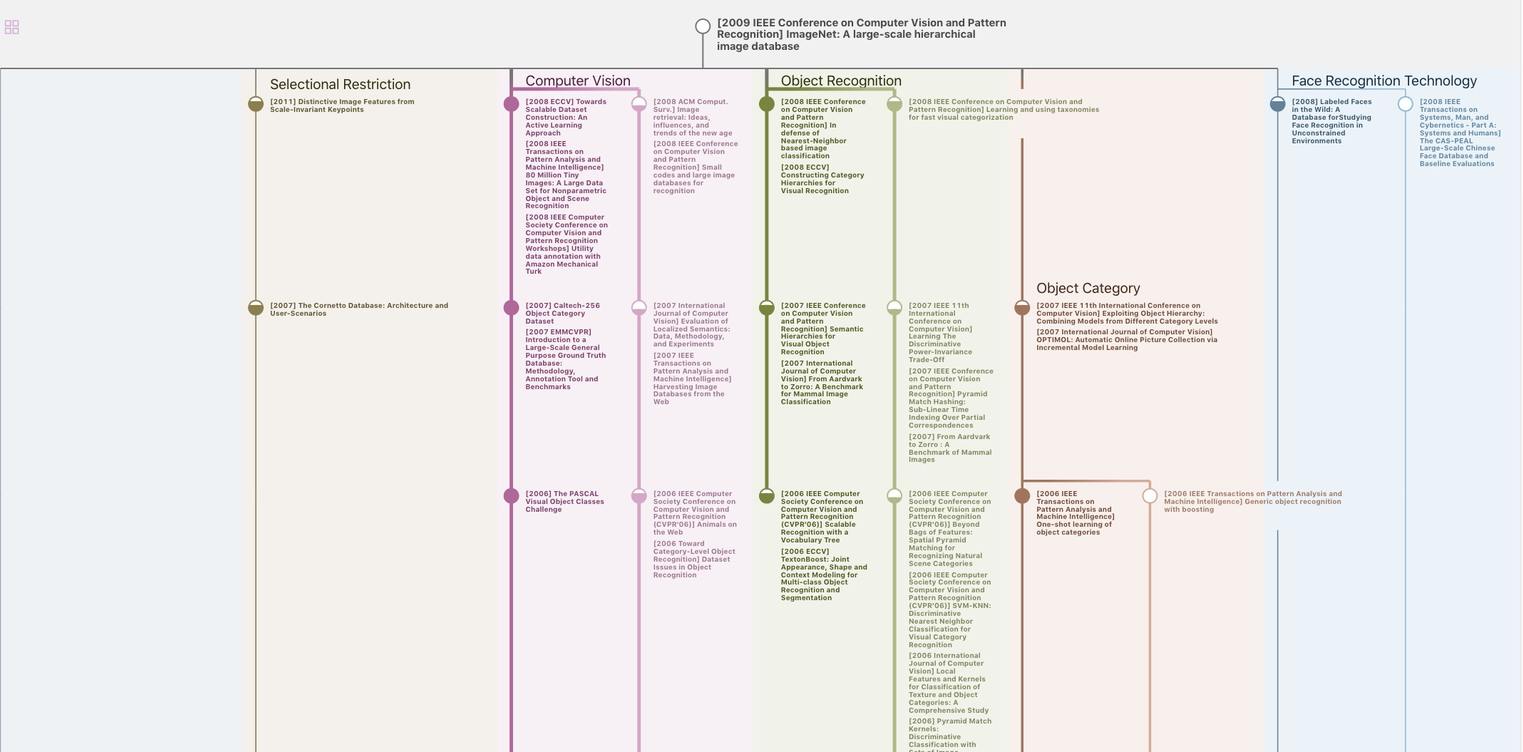
生成溯源树,研究论文发展脉络
Chat Paper
正在生成论文摘要