Resources Scheduling for Ambient Backscatter Communication-Based Intelligent IIoT: A Collective Deep Reinforcement Learning Method.
IEEE Trans. Cogn. Commun. Netw.(2024)
摘要
The rise of edge intelligence is driving a shift in the focus of complexity computing to the edge. Due to network and communication constraints, traditional edge computing resource scheduling solutions for industrial Internet of thing (IIoT) usually face many challenges. For example, delayed decision release, unreasonable policy scheduling and under-utilization of resources. These problems hinder the further construction and advancement of intelligent IIoT. In order to solve these problems, this paper proposes an edge computing resource scheduling scheme based on collective learning. The process of model training is formulated as a Markovian decision process (MDP). The scheme enables edge nodes to exchange learning experiences of resource scheduling schemes, through a shared ledger on the blockchain, including parameters for initial model training. The updated policy scheduling scheme is then obtained through a collective deep reinforcement learning (CDRL) algorithm. Also, to reduce the transmission burden of the underlying industrial devices, we benefit ambient backscatter communication (AmBC) to improve the power utilization of battery. Simulation results display our proposed scheme can reduce energy consumption significantly, while decreased approximately 12.6% compare to A3C algorithm.
更多查看译文
关键词
Mobile edge computing (MEC),ambient backscatter communication (AmBC),industrial Internet of Things (IIoT),blockchain,collective deep reinforcement learning (CDRL)
AI 理解论文
溯源树
样例
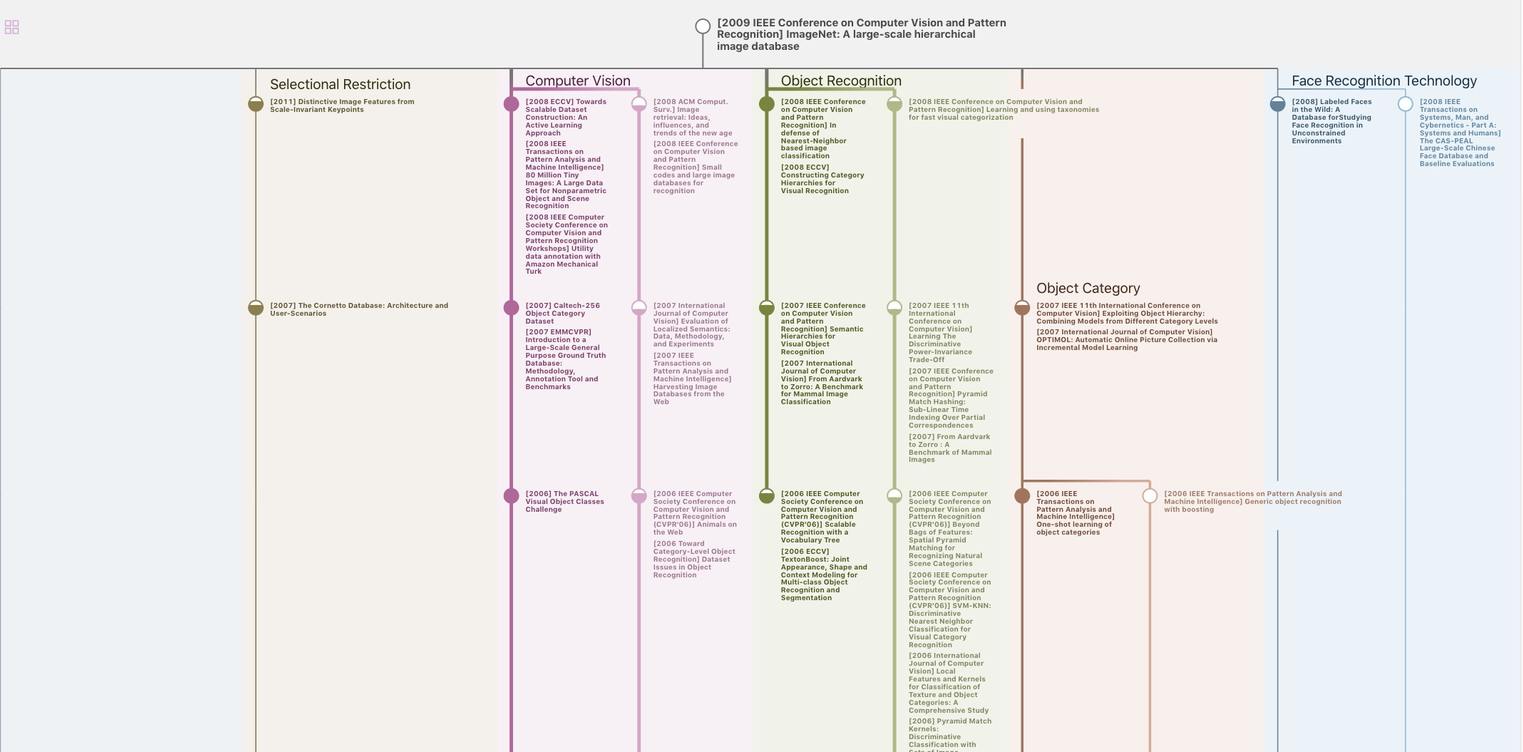
生成溯源树,研究论文发展脉络
Chat Paper
正在生成论文摘要