Anchor-Enhanced Geographical Entity Representation Learning
IEEE TRANSACTIONS ON NEURAL NETWORKS AND LEARNING SYSTEMS(2023)
摘要
Geographical entity representation learning (GERL) aims to embed geographical entities into a low-dimensional vector space, which provides a generalized approach for utilizing geographical entities to serve various geographical intelligence applications. In practice, the spatial distribution of geographical entities is highly unbalanced; thus, it is challenging to embed them accurately. Previous GERL models treated all geographical entities uniformly, resulting in insufficient entity representations. To address this issue, this article proposes an anchor-enhanced GERL (AE-GERL) model, which utilizes the key informative entities as anchors to improve the representations of geographical entities. Specifically, AE-GERL develops an anchor selection algorithm to identify anchors from large-scale geographical entities based on their spatial distribution and entity types. To utilize anchors to guide geographical entities, AE-GERL constructs an anchor-enhanced graph to establish explicit connections between anchors and nonanchor entities. Finally, a graph neural network (GNN) based anchor to nonanchor node learning model is designed to impute missing information of nonanchor entities. Extensive experiments are conducted on four datasets, and the experimental results demonstrate that AE-GERL outperforms the baseline models in both sparse and dense scenarios. This study provides a methodological reference for embedding geographical entities in various geographical applications and also provides an effective approach to improve the performance of message-passing-based GNN models.
更多查看译文
关键词
Representation learning,Semantics,Roads,Trajectory,Graph neural networks,Geology,Time complexity,Geographical entity,graph neural network (GNN),representation learning
AI 理解论文
溯源树
样例
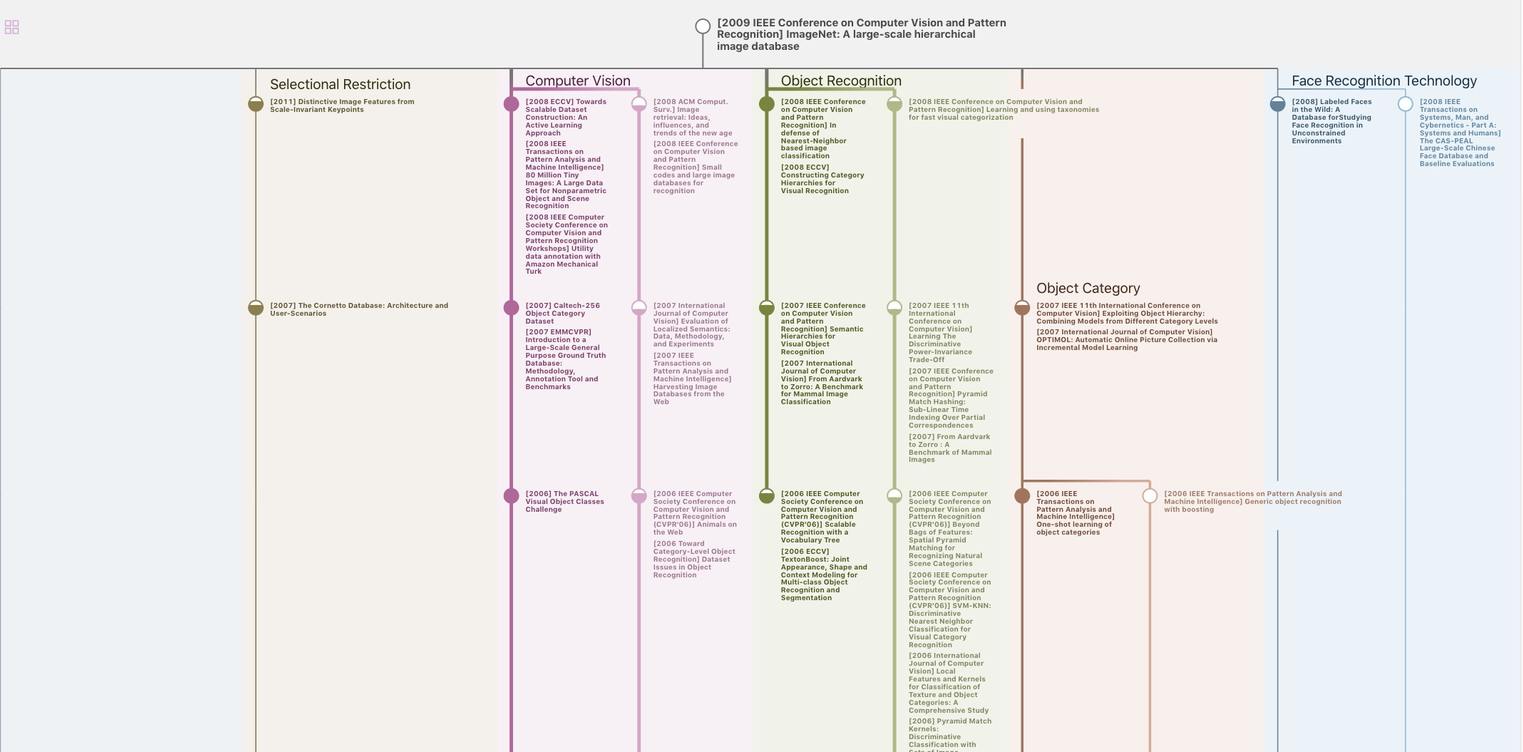
生成溯源树,研究论文发展脉络
Chat Paper
正在生成论文摘要