Convolutional Neural Network With Reinforcement Learning for Trajectories Boundedness of Fault Ride-Through Transients of Grid-Feeding Converters in Microgrids
IEEE TRANSACTIONS ON INDUSTRIAL INFORMATICS(2024)
摘要
The transient stability of the grid-feeding voltage source converter (GFD-VSC) has been studied in the context of weak-grid connections. This article investigates the vulnerability of the fault ride-through (FRT) transient of the GFD-VSC in inverter-dominated autonomous microgrids, where the GFD-VSC observes different impedance characteristics. The transient impedance model of the GFD-VSC is developed considering the current controller/saturation block and studying the impact of the phase-locked loop (PLL) synchronizing unit. The saturation of the current controller imposes a significant phase shift and the PLL's consequent action drives the GFD-VSC to a floating reference frame. The boundedness of the trajectories is evaluated through the nonlinear phase system analysis. It is shown that the system is susceptible to instability depending on its operating conditions such as power factor and the X/R ratio of feeder impendence. A state feedback control is proposed to bound the FRT trajectories of the GFD-VSC. The robust performance of the proposed method is reinforced by utilizing the intelligent deep reinforcement learning (DRL) method to adjust the feedback gain. A convolutional neural network based architecture is proposed for the DRL agent to solve the computational issue related to training and operating the DRL agent in a dynamic time scale of power converters. Numerical simulations validate the proposed method.
更多查看译文
关键词
Deep reinforcement learning (DRL),fault ride-through (FRT),grid-feeding converters,phase-locked loop (PLL),phase systems,renewable energy resources,transient stability
AI 理解论文
溯源树
样例
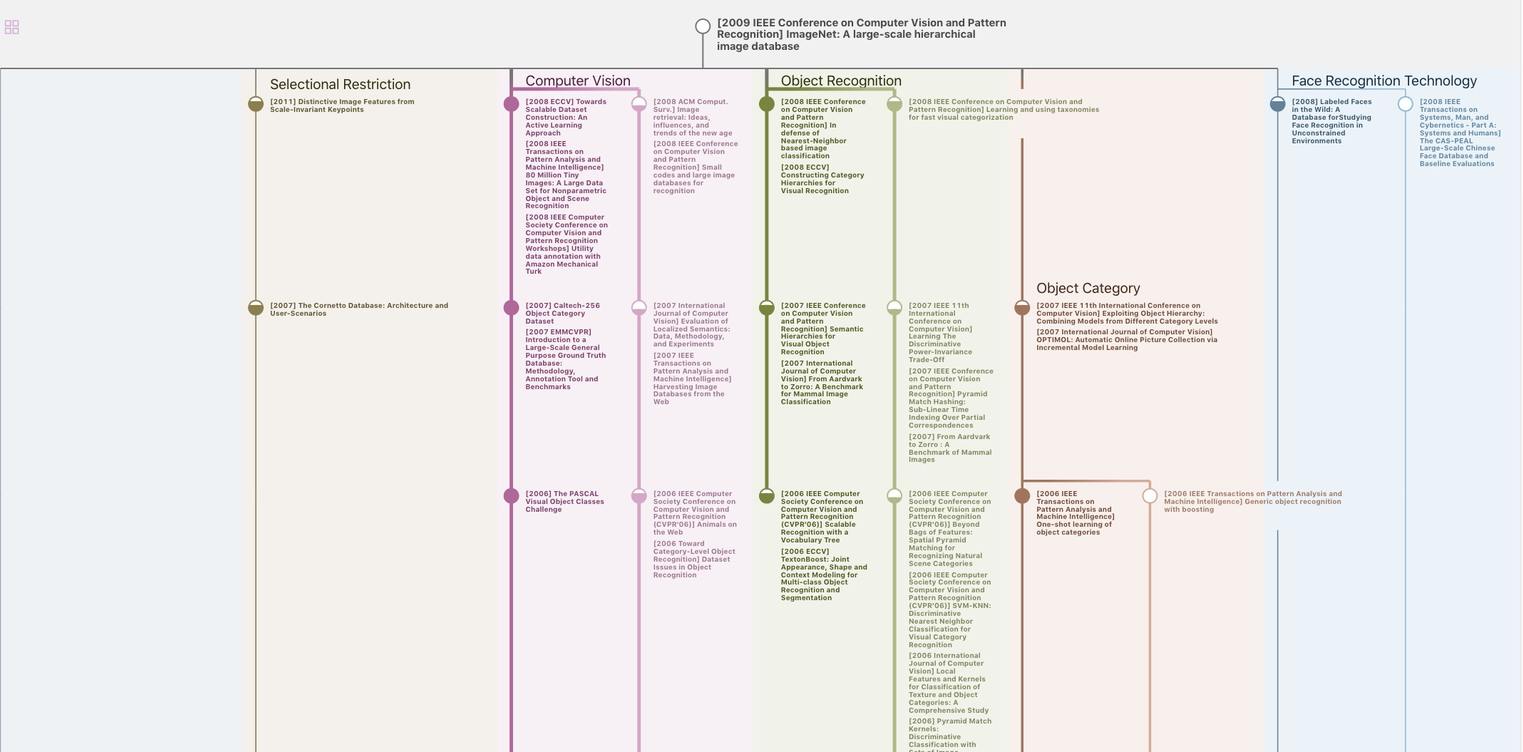
生成溯源树,研究论文发展脉络
Chat Paper
正在生成论文摘要