Efficient descriptors and active learning for grain boundary segregation
PHYSICAL REVIEW MATERIALS(2023)
摘要
Segregation of solutes to grain boundaries (GBs) is an important process having a large impact on mechanical properties of metallic alloys. In this work, we show how accurate density functional theory (DFT) calculations can be combined with machine learning methods to obtain reliable GB segregation energies with significantly lower computational efforts compared to a full ab initio approach. First we compare various descriptor sets with respect to their efficiency in predicting segregation energies for arbitrary GB types. Second, we demonstrate that active learning can be employed to optimize the initial training set obtained by DFT calculations. The methodology is applied to the GB segregation of Re in a WRe alloy, which is a well-studied system of technological relevance.
更多查看译文
AI 理解论文
溯源树
样例
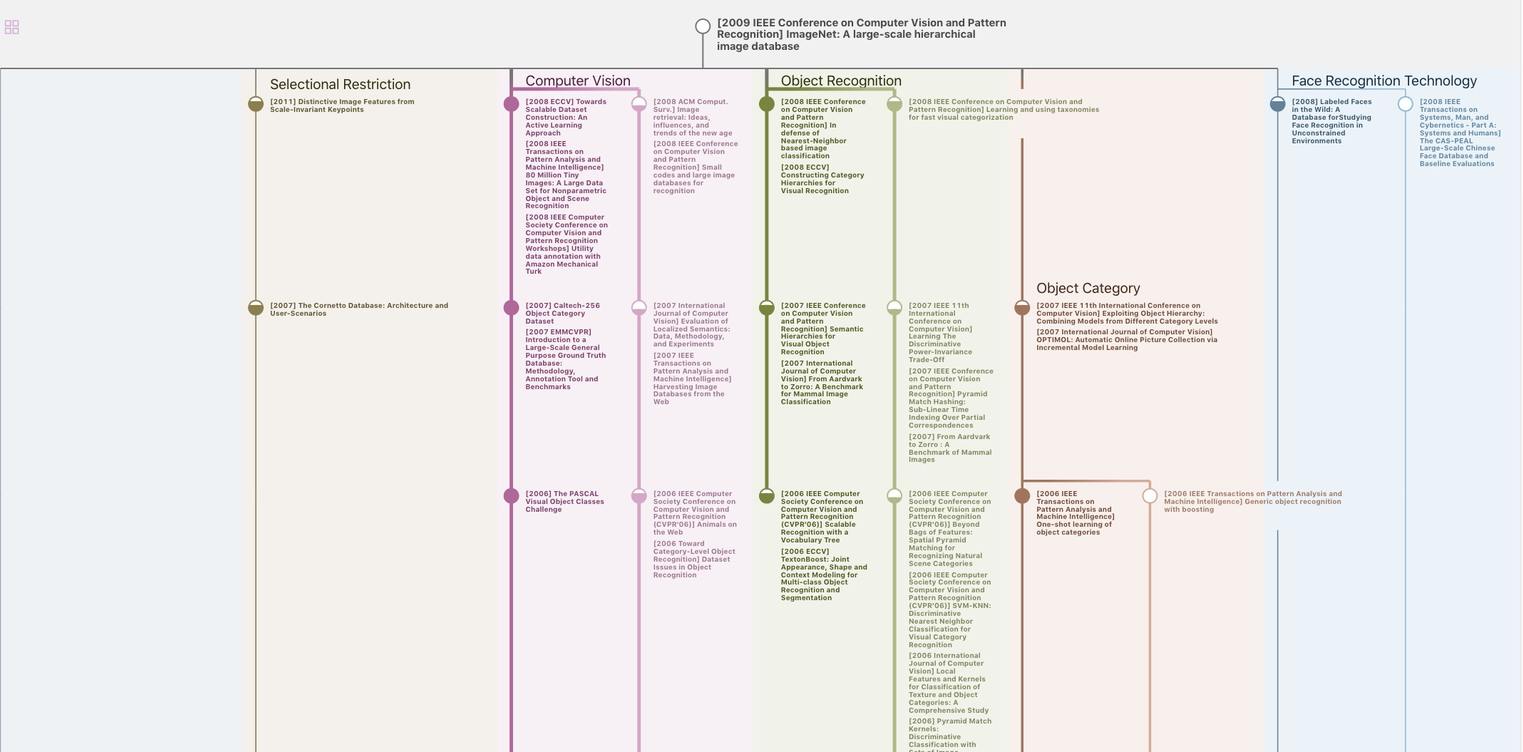
生成溯源树,研究论文发展脉络
Chat Paper
正在生成论文摘要