Transfer-Learning-Based Long Short-Term Memory Model for Machine Tool Spindle Thermal Displacement Compensation
IEEE SENSORS JOURNAL(2024)
摘要
In precision machining industries, maintaining the machining precision of machine tools is critical. Spindle thermal displacement resulting from heat impresses the machining precision of machine tools, and traditional tool machining is often regarded as an open-loop system. Because the output of machine tools depends on the operator's input, the machining precision is determined by the operator's machining experience. Therefore, operators should typically focus on the current status of machine tools and apply any required corresponding adjustments. To address the aforementioned problems, this study proposed a real-time method for measuring the temperature-sensitive points of machine tools and used transfer long short-term memory (LSTM) networks to establish and predict thermal displacement compensation models. The predicted thermal displacement values allowed for feedback compensation of thermal displacement through FANUC Open CNC API Specifications to convert the original open-loop system to a closed-loop control system. The experimental results indicated that before transfer LSTM compensation was used, the spindle thermal displacement measured by an eddy current displacement sensor exhibited a final error of approximately 20 mu m. However, after LSTM compensation was applied, the measurement errors remained within 5 mu m. This decrease in the overall measurement errors confirmed the effectiveness of the proposed system.
更多查看译文
关键词
Temperature sensors,Temperature measurement,Machine tools,Machining,Sensors,Deformation,Predictive models,Long short-term memory (LSTM),machine spindle,milling,thermal displacement compensation,transfer learning
AI 理解论文
溯源树
样例
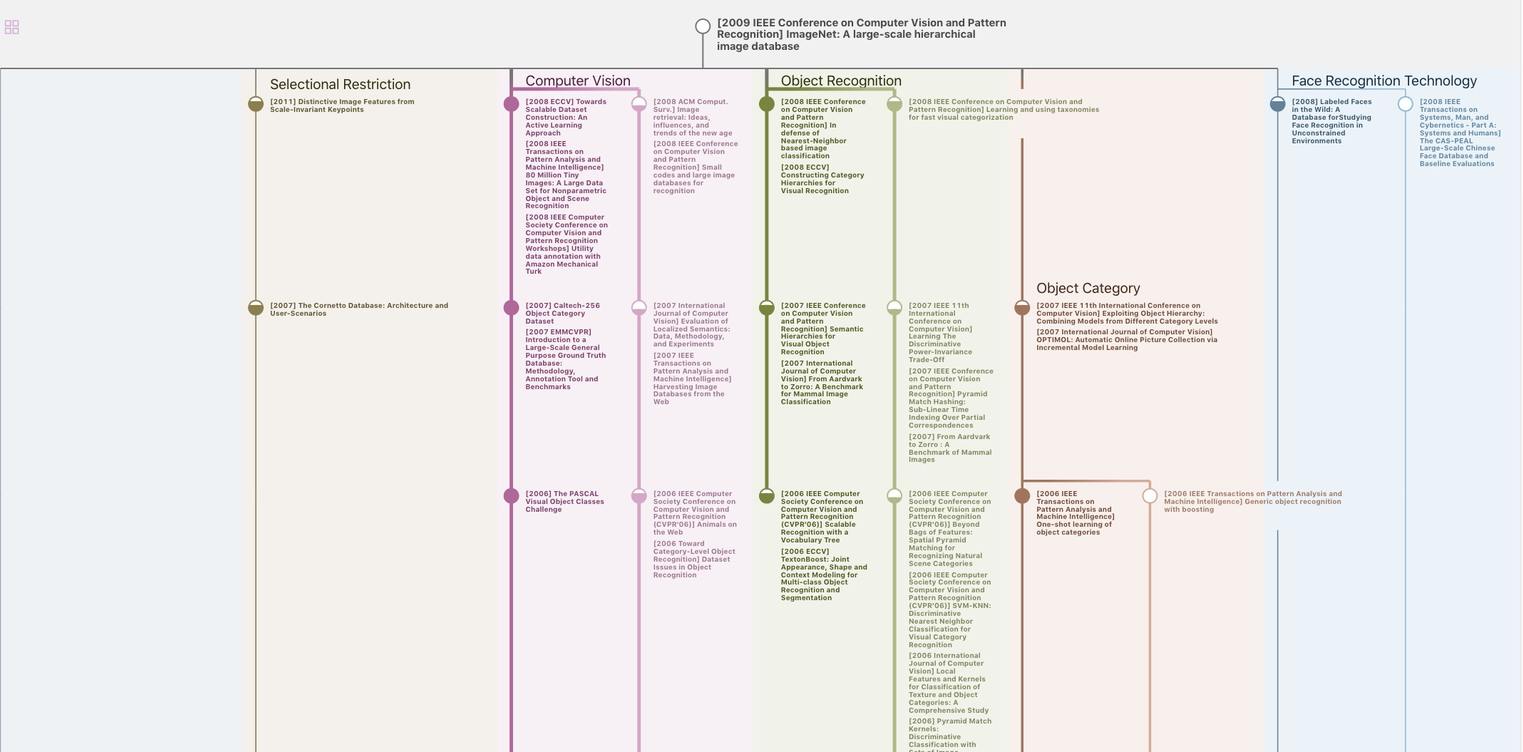
生成溯源树,研究论文发展脉络
Chat Paper
正在生成论文摘要