Efficient Processing of MLPerf Mobile Workloads Using Digital Compute-In-Memory Macros
IEEE TRANSACTIONS ON COMPUTER-AIDED DESIGN OF INTEGRATED CIRCUITS AND SYSTEMS(2024)
摘要
Compute-in-memory (CIM) has recently emergedas a promising design paradigm to accelerate deep neuralnetwork (DNN) processing. Continuously better energy and areaefficiency at the macrolevel had been reported through manytestchips over the last few years. However, in those macrodesign-oriented studies, accelerator-level considerations, such asmemory accesses and processing of entire DNN workloads havenot been investigated in-depth. In this article, we aim to fill thisgap starting with the characteristics of our latest CIM macrofabricated with cutting-edge FinFET CMOS technology at 4-nmnode. We then study, through an accelerator simulator developedin-house, three key items that would determine the efficiency ofour CIM macro in the accelerator context while running MLPerfMobile suite: 1) dataflow optimization; 2) optimal selection ofCIM macro dimensions to further improve macro utilization;and 3) optimal combination of multiple CIM macros. Althoughthere is typically a stark contrast between macro-level peak andaccelerator-level average throughput and energy efficiency, theaforementioned optimizations are shown to improve the macroutilization by 3.04xand reduce the energy-delay product (EDP)to 0.34xcompared to the original macro on MLPerf Mobileinference workloads. While we exploit a digital CIM macro in thisstudy, the findings and proposed methods remain valid for othertypes of CIM (such as analog CIM and analog-digital-hybridCIM) as well.
更多查看译文
关键词
Adders,Microprocessors,Energy efficiency,Common Information Model (computing),Random access memory,Throughput,System-on-chip,Compute-in-memory (CIM),deep learning,MLPerf benchmark
AI 理解论文
溯源树
样例
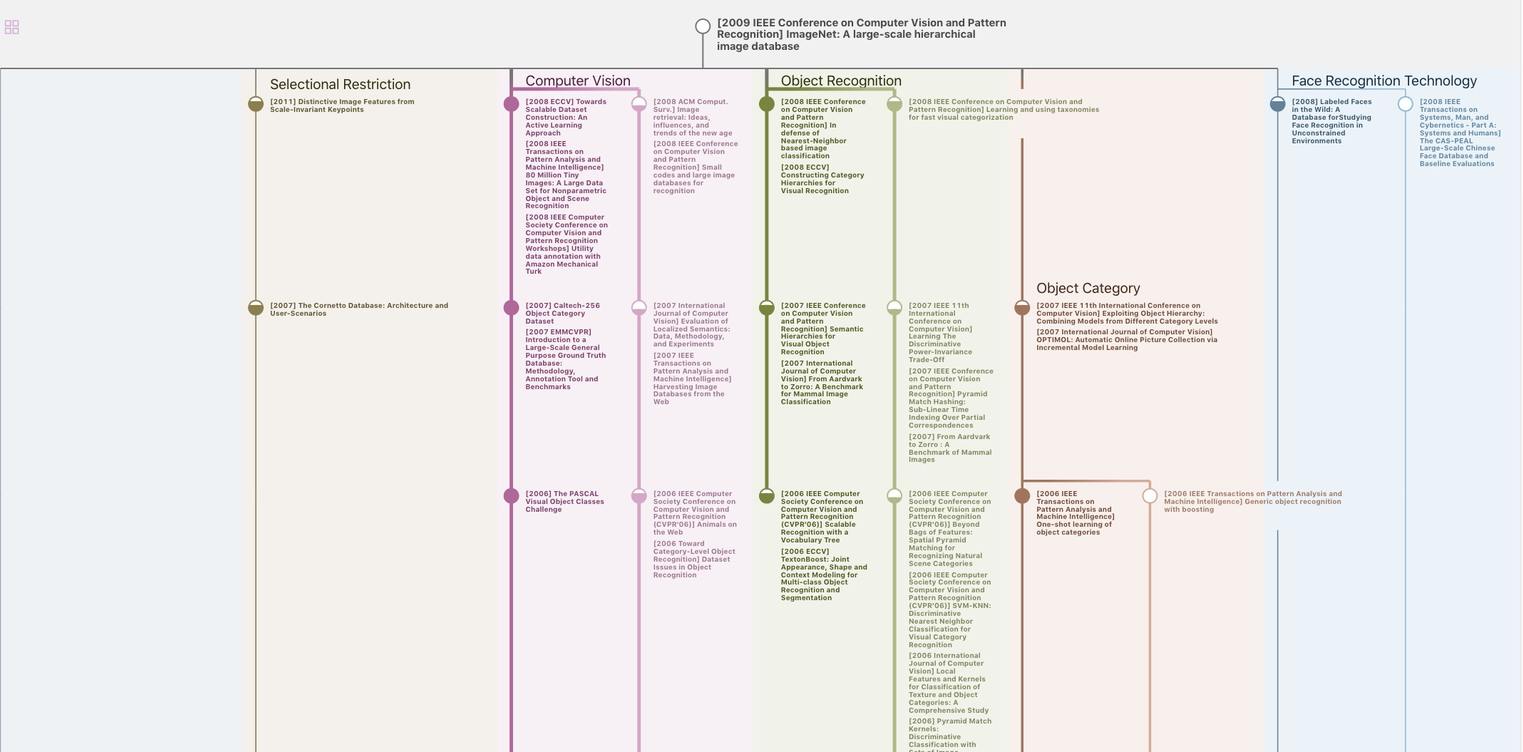
生成溯源树,研究论文发展脉络
Chat Paper
正在生成论文摘要