Image-to-Text Conversion and Aspect-Oriented Filtration for Multimodal Aspect-Based Sentiment Analysis
IEEE Transactions on Affective Computing(2023)
摘要
Multimodal aspect-based sentiment analysis (MABSA) aims to determine the sentiment polarity of each aspect mentioned in the text based on multimodal content. Various approaches have been proposed to model multimodal sentiment features for each aspect via modal interactions. However, most existing approaches have two shortcomings: (1) The representation gap between textual and visual modalities may increase the risk of misalignment in modal interactions; (2) In some examples where the image is not related to the text, the visual information may not enrich the textual modality when learning aspect-based sentiment features. In such cases, blindly leveraging information from visual modal may introduce noises in reasoning the aspect-based sentiment expressions. To tackle the shortcomings mentioned above, we propose an end-to-end MABSA framework with image conversion and noise filtration. Specifically, to bridge the representation gap in different modalities, we attempt to translate images into the input space of a pre-trained language model (PLM). To this end, we develop an image-to-text conversion module that can convert an image to an implicit sequence of token embedding. Moreover, an aspect-oriented filtration module is devised to alleviate the noise in the implicit token embeddings, which consists of two attention operations. The former aims to create an enhanced aspect embedding as a query, and the latter seeks to use this query to retrieve relevant auxiliary information from the implicit token embeddings to supplement the textual content. After filtering the noise, we leverage a PLM to encode the text, aspect, and image prompt derived from filtered implicit token embeddings as sentiment features to perform aspect-based sentiment prediction. Experimental results on two MABSA datasets show that our framework achieves state-of-the-art performance. Furthermore, extensive experimental analysis demonstrates the proposed framework has superior robustness and efficiency.
更多查看译文
关键词
Aspect-Based Sentiment Analysis,Multimodal Sentiment Analysis,Pre-trained Language Model,Natural Language Processing
AI 理解论文
溯源树
样例
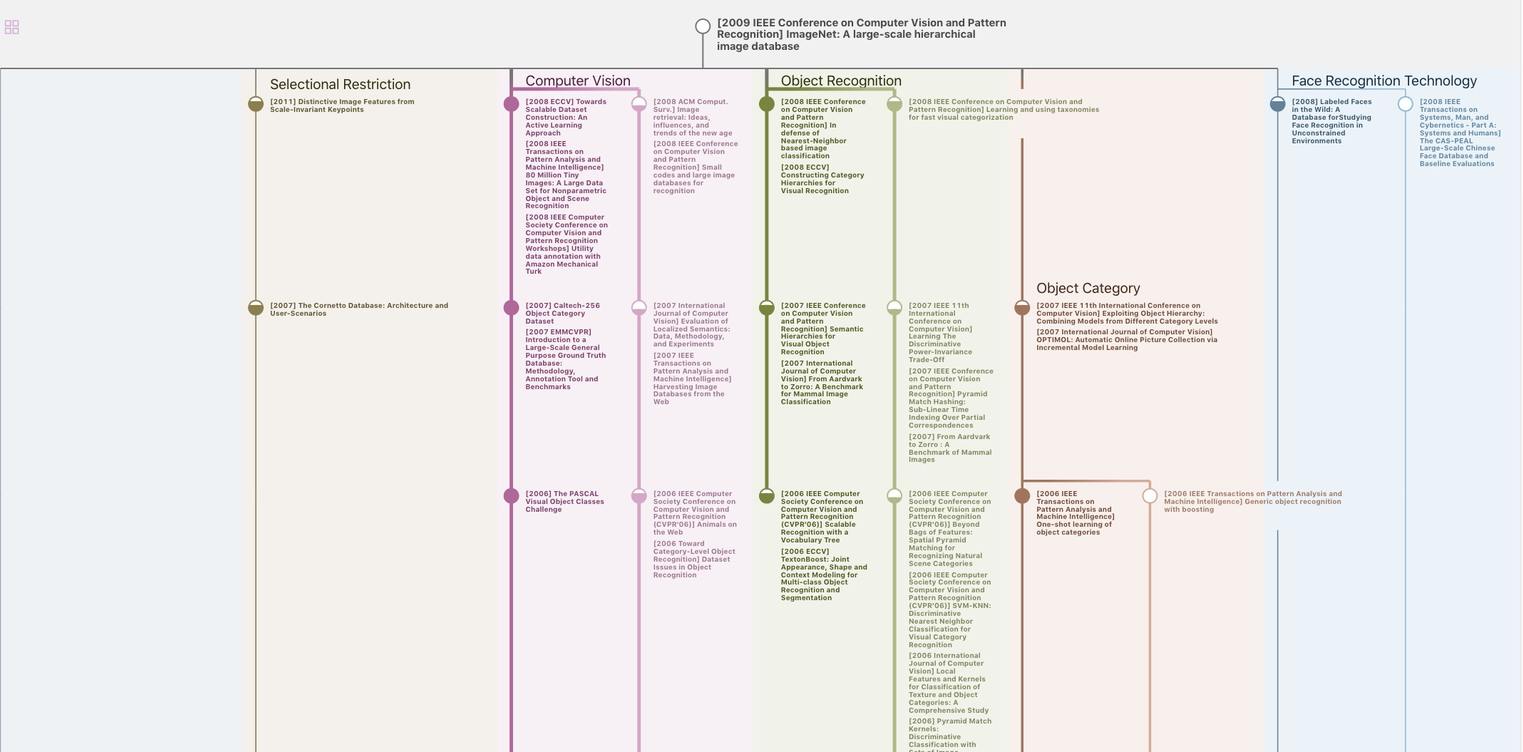
生成溯源树,研究论文发展脉络
Chat Paper
正在生成论文摘要