Feature Weighted Multi-View Graph Clustering.
IEEE Trans. Consumer Electron.(2024)
摘要
Graph-based multi-view clustering aims to learn an affinity graph by exploiting consistent and complementary information from multiple views. However, most existing methods suffer the following problems: (1) they treat all features equally for each view during learning, but the redundant and corrupted features should not be treated equally without discrimination; (2) they sometimes employ low-rank constraint to capture the latent global manifold structure of views, this inevitably introduces a parameter that is difficult to tune, and aggravates the computational complexity; and (3) they usually fed the raw multi-view data into graph learning model directly, but the unclean data impair the quality of affinity graph greatly. In this work, we propose a unified Feature Weighted Multi-view Graph Clustering (FWMGC) method to tackle the above problems. For each view, a latent low-rank weighting matrix with mandatory row-sparsity property is efficiently learned to assign weight values to different feature relations, so as to select important features and ensure the robustness to noise. Furthermore, the complementary and consistent information of the clean weighted multi-view data are integrated to learn a consensus affinity graph. Comprehensive experiments on some widely used benchmark datasets demonstrate that the proposed FWMGC yields significant improvements of clustering performance and running time.
更多查看译文
关键词
Affinity graph learning,feature weighting,graph clustering,multi-view clustering,noise removal
AI 理解论文
溯源树
样例
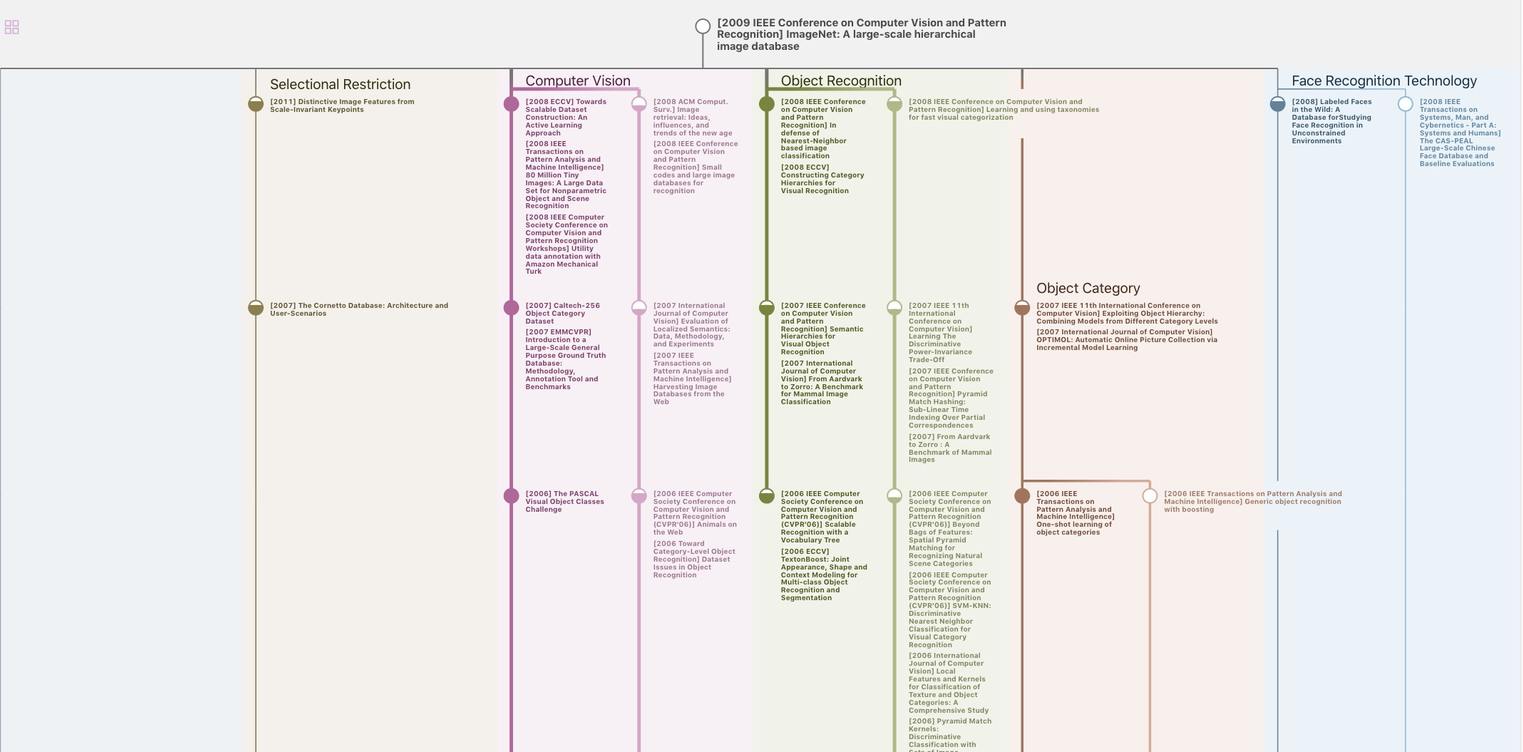
生成溯源树,研究论文发展脉络
Chat Paper
正在生成论文摘要