A multi-layer feature fusion fault diagnosis method for train bearings under noise and variable load working conditions
MEASUREMENT SCIENCE AND TECHNOLOGY(2024)
摘要
The working characteristics of noise and variable load conditions make it challenging to extract the feature of train bearing vibration signal. Therefore, a multi-layer feature fusion inverted residual network (MFIRN) is proposed. Firstly, a joint shrinkage denoising module (JSDM) is proposed, and an inverted residual denoising module (IRDM) is designed by combining the JSDM with the inverted residual network. The IRDM is used as the basic unit to improve the anti-noise performance of MFIRN. Then, a global interactive awareness module (GIAM) is designed to perceive the information of different layers, which can effectively extract the fault characteristics of bearing signals under variable load conditions. Finally, the fault diagnosis capabilities of the MFIRN under noisy and variable load conditions are tested using two cases, and the effectiveness of the JSDM, GIAM and IRDM is verified by ablation experiments. The experimental results demonstrate that, compared with seven recent models, the MFIRN can filter out the interference information more effectively under different noise conditions. Furthermore, MFIRN can effectively obtain the correlation characteristics between different load signals with better diagnostic accuracy and domain adaptability, showing potential for practical application.
更多查看译文
关键词
train bearing,fault diagnosis,inverted residual network,multi-layer feature fusion,denoising,variable loads
AI 理解论文
溯源树
样例
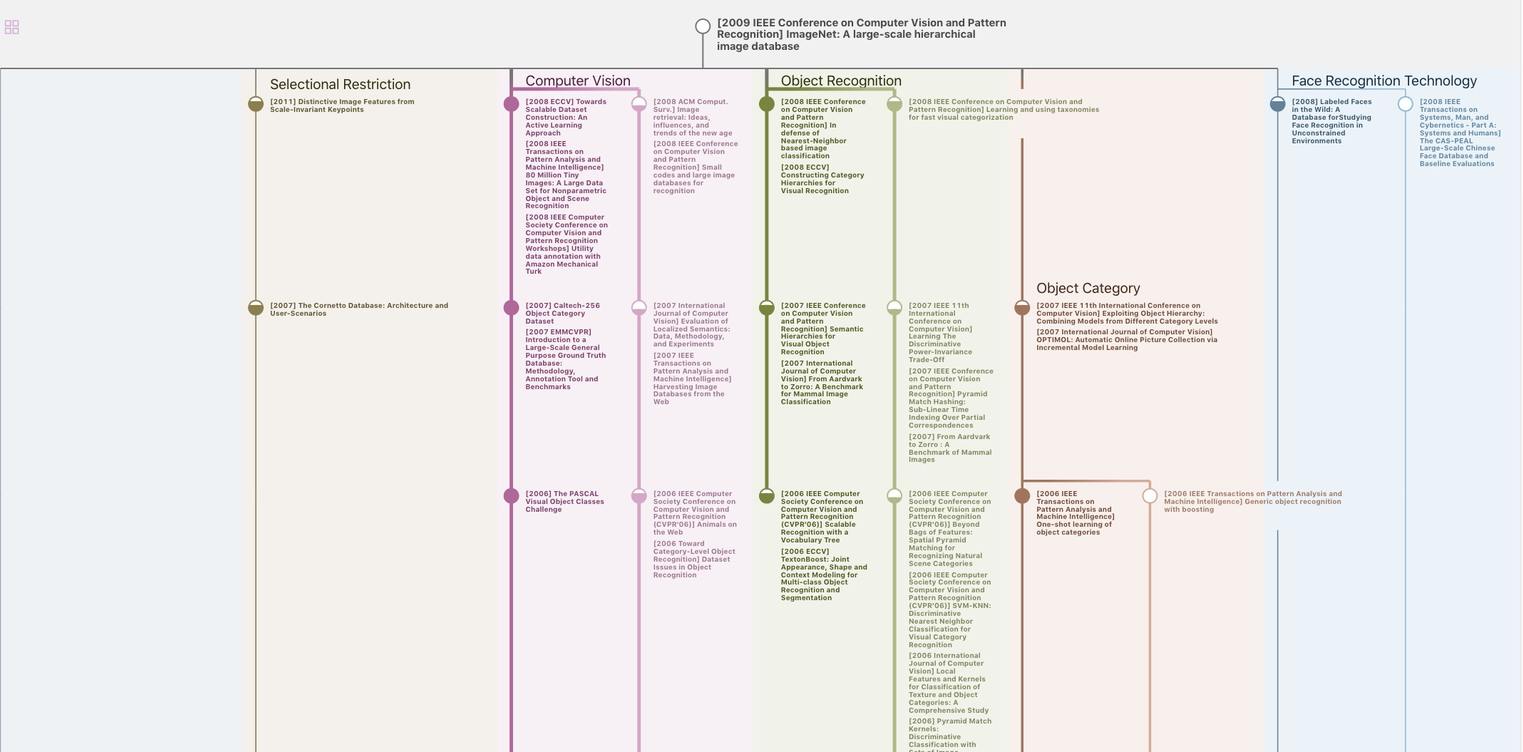
生成溯源树,研究论文发展脉络
Chat Paper
正在生成论文摘要