3D Brain Tumour Segmentation Using UNet with Quantitative Analysis of the Tumour Features
Journal of physics(2023)
摘要
Abstract Brain tumours are abnormal cells that grow within the brain and may cause severe harm to an individual. The diagnosis of tumours often requires a manual review of Magnetic Resonance (MR) images by a skilled radiologist, which is a laborious task. The study aims to use a deep learning-based approach to segment the brain tumour and quantitatively evaluate the performance of the model by comparing the statistical distribution of five textural and four non-textural feature differences between the ground truth and the prediction. Textural features were extracted from a Grey Level Co-occurrence Matrix (GLCM) and averaged over four different directions. A UNet model was constructed with MONAI using BraTS 2020 dataset. Due to hardware constraints, the model was trained over a short epoch range of 100 but managed to achieve a mean Dice similarity coefficient (DSC) of 0.86. The quantitative analysis showed that the ground truth and the prediction had low differences in most features, except for percentage variation in signal intensity, energy, correlation and Hausdorff distance, which could be attributed to false positive voxels that are far from the ground truth. The textural properties of homogeneity, entropy, and correlation had an average absolute percentage difference of less than 5% from the ground truth.
更多查看译文
AI 理解论文
溯源树
样例
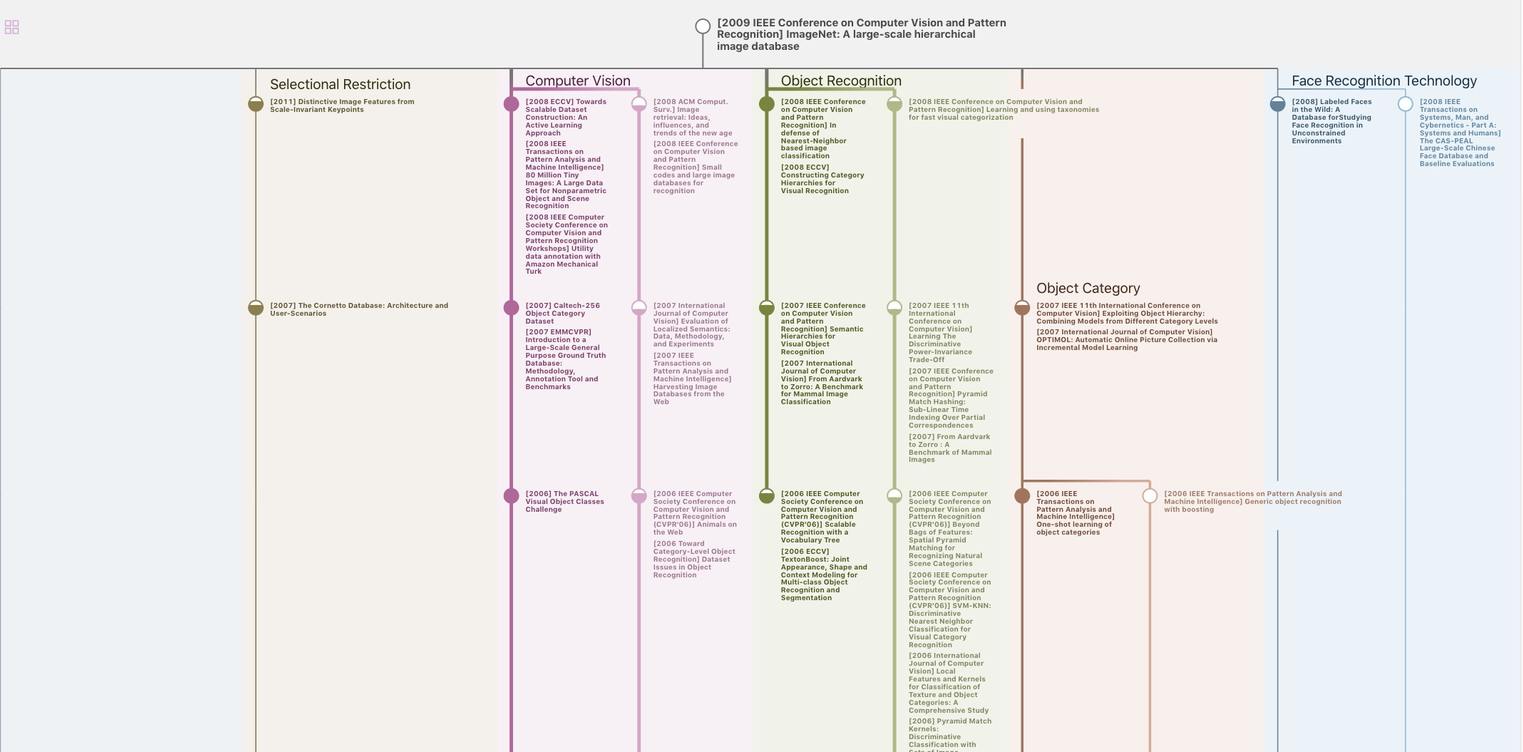
生成溯源树,研究论文发展脉络
Chat Paper
正在生成论文摘要