Comparative analysis of machine learning algorithms for classifying stunting among children under the age of five in Rwanda
Research Square (Research Square)(2023)
摘要
Abstract Purpose: In Rwanda, childhood stunting is a major public health problem. Earlier studies employed traditional statistical approaches to identify causal factors to stunting, and little is known about the uses and effectiveness of machine learning (ML) algorithms that may identify risk factors for a variety of health conditions based on complex data. Methods: This study examines the usefulness of machine learning algorithms in predicting stunting in children under the age of five using data from the 2020 Rwanda Demographic and Health Survey. Random Forest was utilized for feature selection, and supervised machine learning methods were applied. The confusion matrix and Receiver Operating Characteristics (ROC), which incorporated several metrics, were used to evaluate the performance of algorithms. Additionally, the outperformed model identifies variables that strongly predict stunting in Rwanda. Ultimately, multivariate logistic regression was used. Results: The XGBoost classifier predicts stunting with the lowest misclassification error among the selected ML algorithms, followed by a gradient boosting classifier, random forests, support vector machines, classification trees, and logistic regression with forward-stepwise selection. The 10 most important variables in predicting childhood stunting in Rwanda are breastfeeding start, mother’s height, provinces, possessing television, child size at birth, maternal education, maternal BMI, wealth index, preceding birth interval, and child age. Conclusion: This study contributes to the body of knowledge that confirmed the efficacy of ML for population health research and policy decision-making in a broad range of areas, including defining treatment effects in epidemiological studies and child undernutrition. This study shows that the XGBoost classifer is highly recommended because of the combination of flexibility, scalability,regularization, ensemble approaches, and feature importance that distinguishesXGBoost from gradient-based classifers.
更多查看译文
关键词
stunting,children,rwanda
AI 理解论文
溯源树
样例
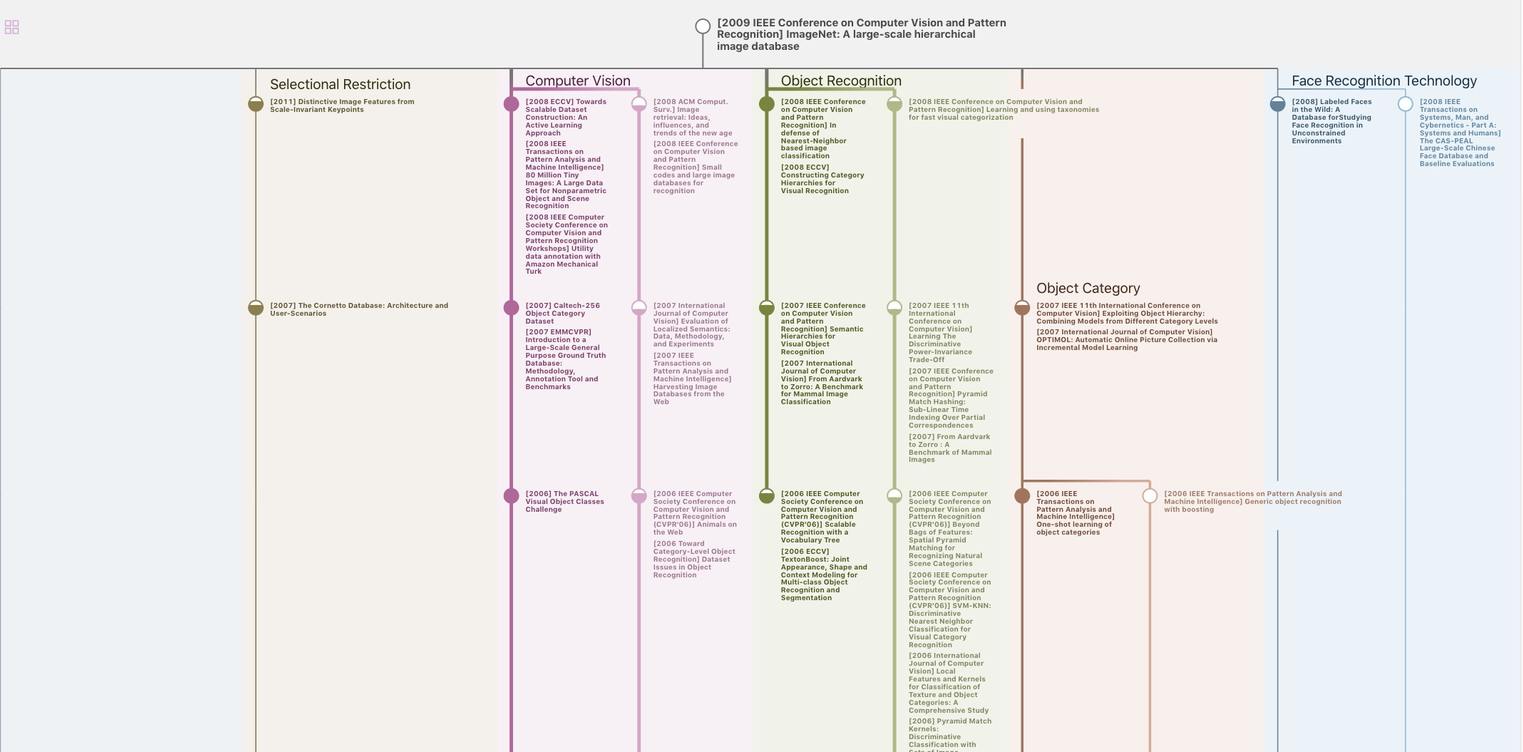
生成溯源树,研究论文发展脉络
Chat Paper
正在生成论文摘要