Unsupervised Object-Centric Voxelization for Dynamic Scene Understanding
arXiv (Cornell University)(2023)
摘要
Understanding the compositional dynamics of multiple objects in unsupervised visual environments is challenging, and existing object-centric representation learning methods often ignore 3D consistency in scene decomposition. We propose DynaVol, an inverse graphics approach that learns object-centric volumetric representations in a neural rendering framework. DynaVol maintains time-varying 3D voxel grids that explicitly represent the probability of each spatial location belonging to different objects, and decouple temporal dynamics and spatial information by learning a canonical-space deformation field. To optimize the volumetric features, we embed them into a fully differentiable neural network, binding them to object-centric global features and then driving a compositional NeRF for scene reconstruction. DynaVol outperforms existing methods in novel view synthesis and unsupervised scene decomposition and allows for the editing of dynamic scenes, such as adding, deleting, replacing objects, and modifying their trajectories.
更多查看译文
关键词
scene,understanding,object-centric
AI 理解论文
溯源树
样例
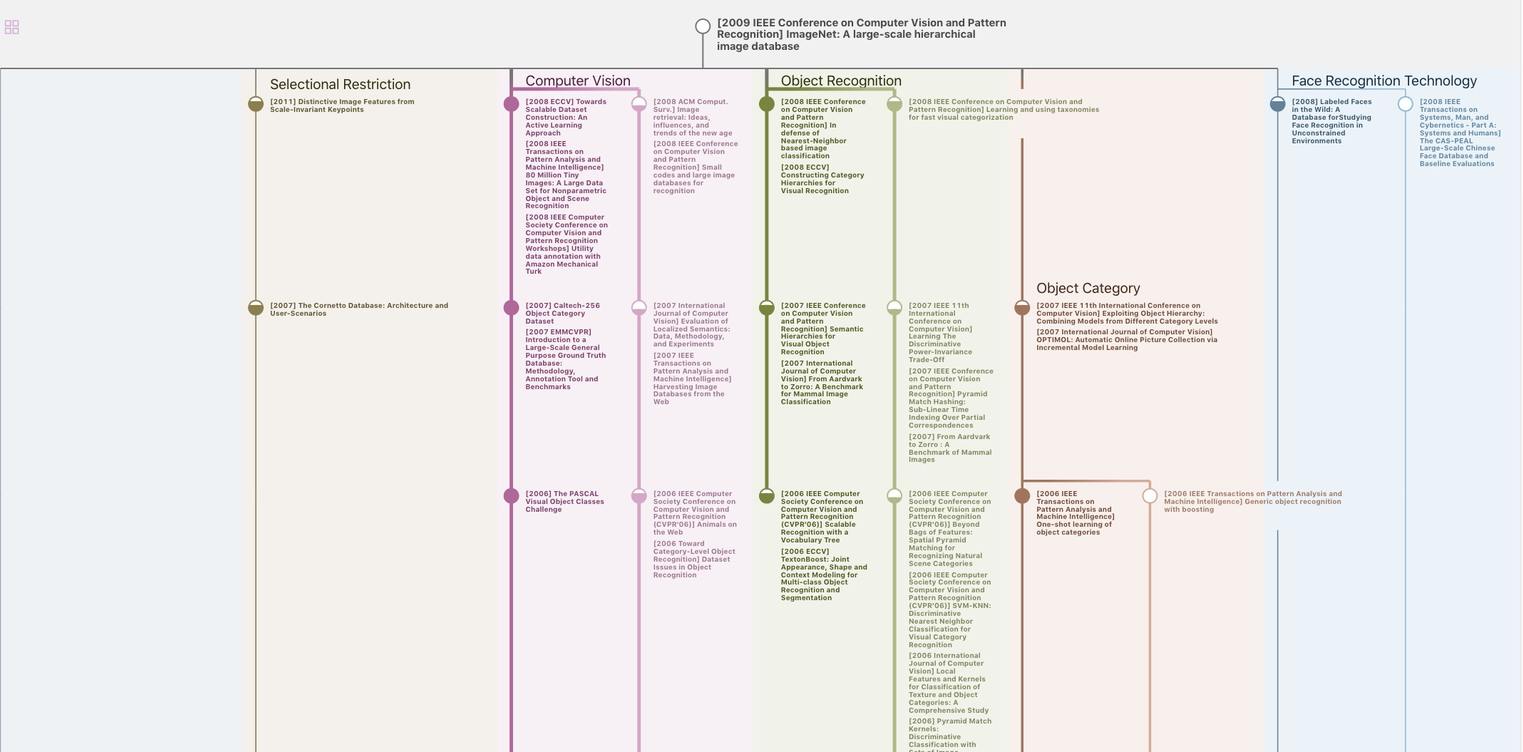
生成溯源树,研究论文发展脉络
Chat Paper
正在生成论文摘要