Po-01-204 an artificial intelligence (ai)-assisted end-to-end computational platform for prediction of extra-pulmonary vein (extra-pvi) ablation targets in atrial fibrillation (af) patients with fibrosis remodeling
Heart Rhythm(2023)
摘要
Existing strategies targeting the extra-PVI AF substrate have failed to improve outcomes, as shown by clinical trials. There is an urgent need to establish new approaches that overcome existing limitations. We have shown previously, in a feasibility study, that bi-atrial personalized computational modeling can guide ablation in patients with fibrosis detected on late gadolinium enhancement MRI (LGE-MRI). However, the approach had laborious aspects, such as segmentation of both atria and fibrosis distribution, and determining substrate locations capable of sustaining rotors. These prevented the approach from being directly implementable in clinical workflows. To develop an AI-assisted computational platform for personalized extra-PVI substrate ablation target prediction in patients with atrial fibrosis detected by LGE-MRI, as part of an FDA-approved randomized clinical trial. We designed an AI-assisted platform enabling end-to-end modeling workflow for predicting extra-PVI ablation targets. The platform consists of 3 main components (Figure): i) personalized bi-atrial model construction from LGE-MRI, ii) personalized simulation of substrate AF inducibility, and iii) personalized extra-PVI ablation strategy implementable in the clinical workflow. For the first component, we developed, validated, and tested a deep learning approach to bi-atrial geometry and fibrosis segmentation, which consisted of a cascade of 4 neural networks (NNs). Extra-PVI substrate rotor inducibility was probed by pacing from numerous sites; locations capable of sustaining persistent drivers were determined using a novel NN approach and ablated. Each ablation was followed by the inducibility test, repeated until full substrate non-inducibility from any pacing site was achieved. The platform was successfully tested on 17 AF patients by multiple operators of differing expertise, with consistent results. Atrial geometry/fibrosis segmentation was achieved in 15 min, as compared to 5 hours pre-AI implementation. Using the NN approach for targeted substrate ablation ensured robustness and reproducibility of the predicted targets. Finally, a seamless import of atrial geometry and the optimal set of targets was achieved in a number of EAM systems. We developed a robust AI-assisted computational platform for prediction of extra-PVI atrial substrate ablation targets in AF patients with fibrosis, enabling our FDA-approved randomized clinical trial.
更多查看译文
关键词
atrial fibrillation,fibrosis remodeling,ablation targets,artificial intelligence,end-to-end,extra-pulmonary,extra-pvi
AI 理解论文
溯源树
样例
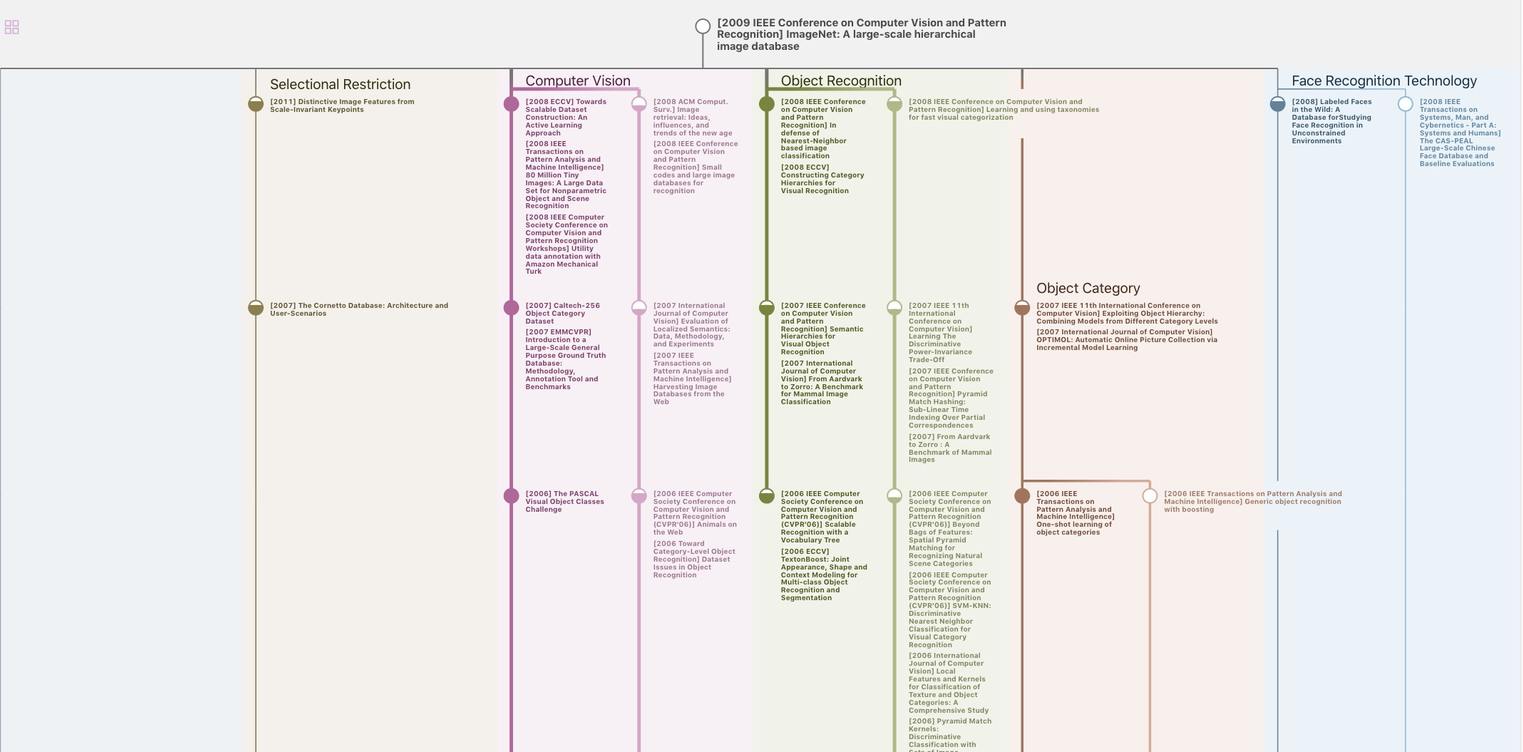
生成溯源树,研究论文发展脉络
Chat Paper
正在生成论文摘要