Deterministic Approximation of a Stochastic Imitation Dynamics with Memory
arXiv (Cornell University)(2023)
摘要
We provide results of a deterministic approximation for non-Markovian stochastic processes modeling finite populations of individuals who recurrently play symmetric finite games and imitate each other according to payoffs. We show that a system of delay differential equations can be obtained as the deterministic approximation of such a non-Markovian process. We also show that if the initial states of stochastic process and the corresponding deterministic model are close enough, then the trajectory of stochastic process stays close to that of the deterministic model up to any given finite time horizon with a probability exponentially approaching one as the population size increases. We use this result to obtain that the lower bound of the population size on the absorption time of the non-Markovian process is exponentially increasing. Additionally, we obtain the replicator equations with distributed and discrete delay terms as examples and analyze how the memory of individuals can affect the evolution of cooperation in a two-player symmetric Snow-drift game. We investigate the stability of the evolutionary stable state of the game when agents have the memory of past population states, and implications of these results are given for the stochastic model.
更多查看译文
关键词
stochastic imitation dynamics,deterministic approximation,memory
AI 理解论文
溯源树
样例
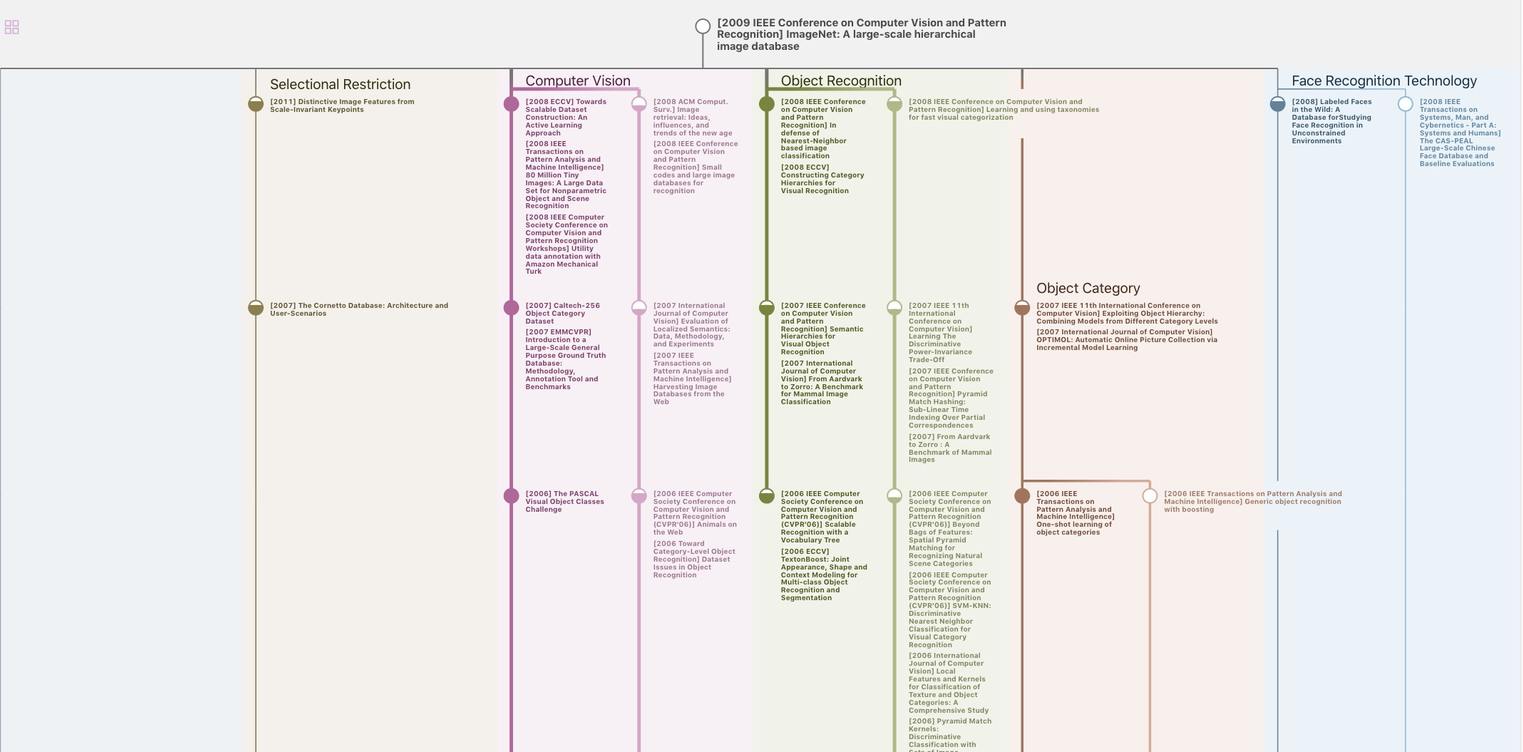
生成溯源树,研究论文发展脉络
Chat Paper
正在生成论文摘要