Restricting datasets to classifiable samples augments discovery of immune disease markers
Research Square (Research Square)(2023)
摘要
Immunological diseases are typically heterogeneous in clinical presentation, severity and response to therapy. Markers of immune diseases often reflect this variability, especially compared to their regulated behavior in health. This leads to a common, unarticulated problem that frustrates marker discovery and interpretation: Unequal variance of immune disease marker expression between patient classes necessarily limits a marker’s informative range. To solve this problem, we introduce dataset restriction, a procedure that splits datasets into classifiable and unclassifiable samples. Applied to synthetic flow cytometry data, restriction identified markers that were otherwise disregarded. In advanced melanoma, restriction found new markers of immune-related adverse event risk after immunotherapy and enabled multivariate models that accurately predicted immunotherapy-related hepatitis. Hence, dataset restriction augments discovery of immune disease markers, increases predictive certainty for classifiable samples and improves multivariate models incorporating markers with a limited informative range. This principle can be directly extended to any classification task.
更多查看译文
关键词
datasets,samples augments discovery
AI 理解论文
溯源树
样例
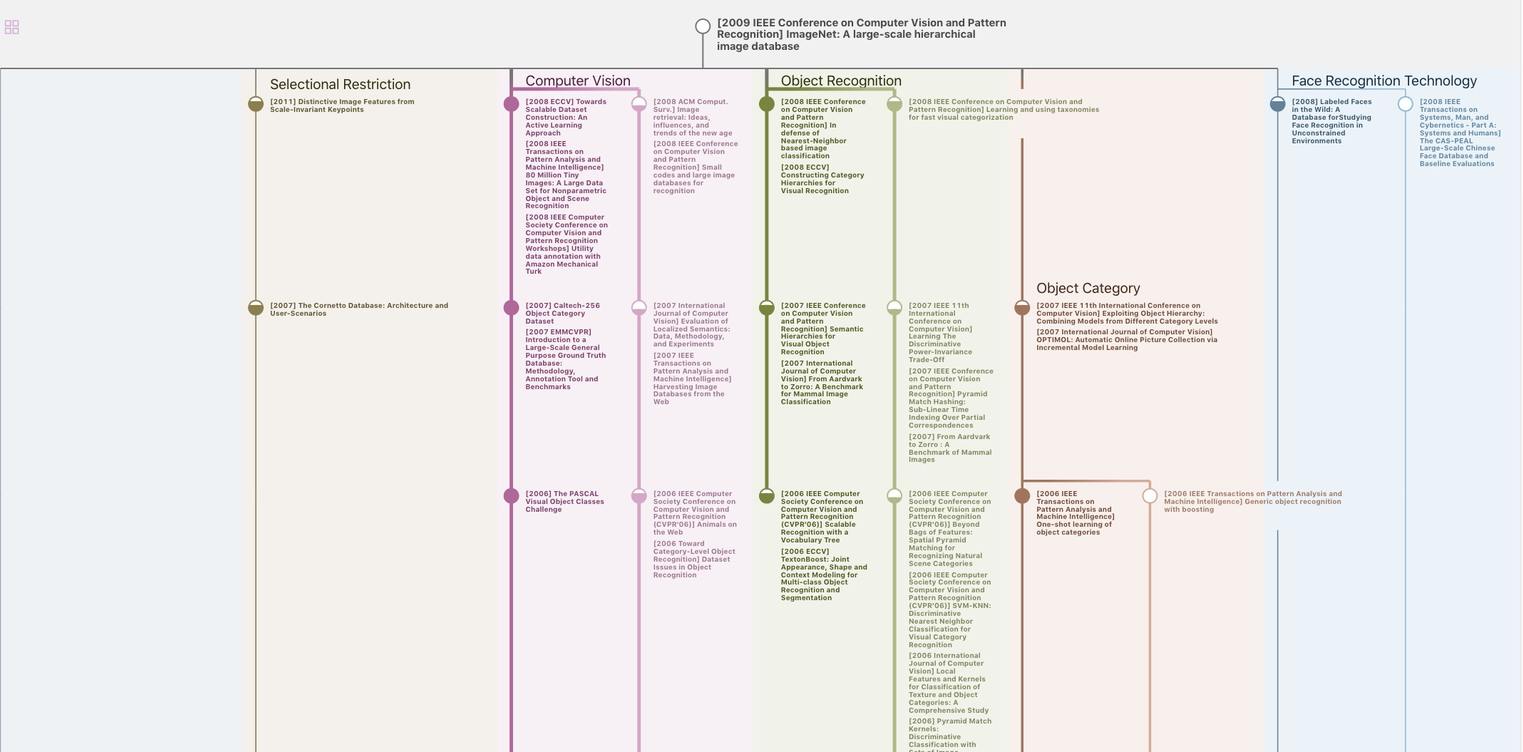
生成溯源树,研究论文发展脉络
Chat Paper
正在生成论文摘要