Contrastive Correlation Preserving Replay for Online Continual Learning
IEEE TRANSACTIONS ON CIRCUITS AND SYSTEMS FOR VIDEO TECHNOLOGY(2024)
摘要
Online Continual Learning (OCL), as a core step towards achieving human-level intelligence, aims to incrementally learn and accumulate novel concepts from streaming data that can be seen only once, while alleviating catastrophic forgetting on previously acquired knowledge. Under this mode, the model needs to learn new classes or tasks in an online manner, and the data distribution may change over time. Moreover, task boundaries and identities are not available during training and evaluation. To balance the stability and plasticity of networks, in this work, we propose a replay-based framework for OCL, named Contrastive Correlation Preserving Replay (CCPR), which focuses on not only instances but also correlations between multiple instances. Specifically, besides the previous raw samples, the corresponding representations are stored in the memory and used to construct correlations for the past and the current model. To better capture correlation and higher-order dependencies, we maximize the low bound of mutual information between the past correlation and the current correlation by leveraging contrastive objectives. Furthermore, to improve the performance, we propose a new memory update strategy, which simultaneously encourages the balance and diversity of samples within the memory. With limited memory slots, it allows less redundant and more representative samples for later replay. We conduct extensive evaluations on several popular CL datasets, and experiments show that our method consistently outperforms the state-of-the-art methods and can effectively consolidate knowledge to alleviate forgetting.
更多查看译文
关键词
Task analysis,Correlation,Knowledge transfer,Training,Memory management,Data models,Mutual information,Continual learning,catastrophic forgetting,class-incremental learning,experience replay
AI 理解论文
溯源树
样例
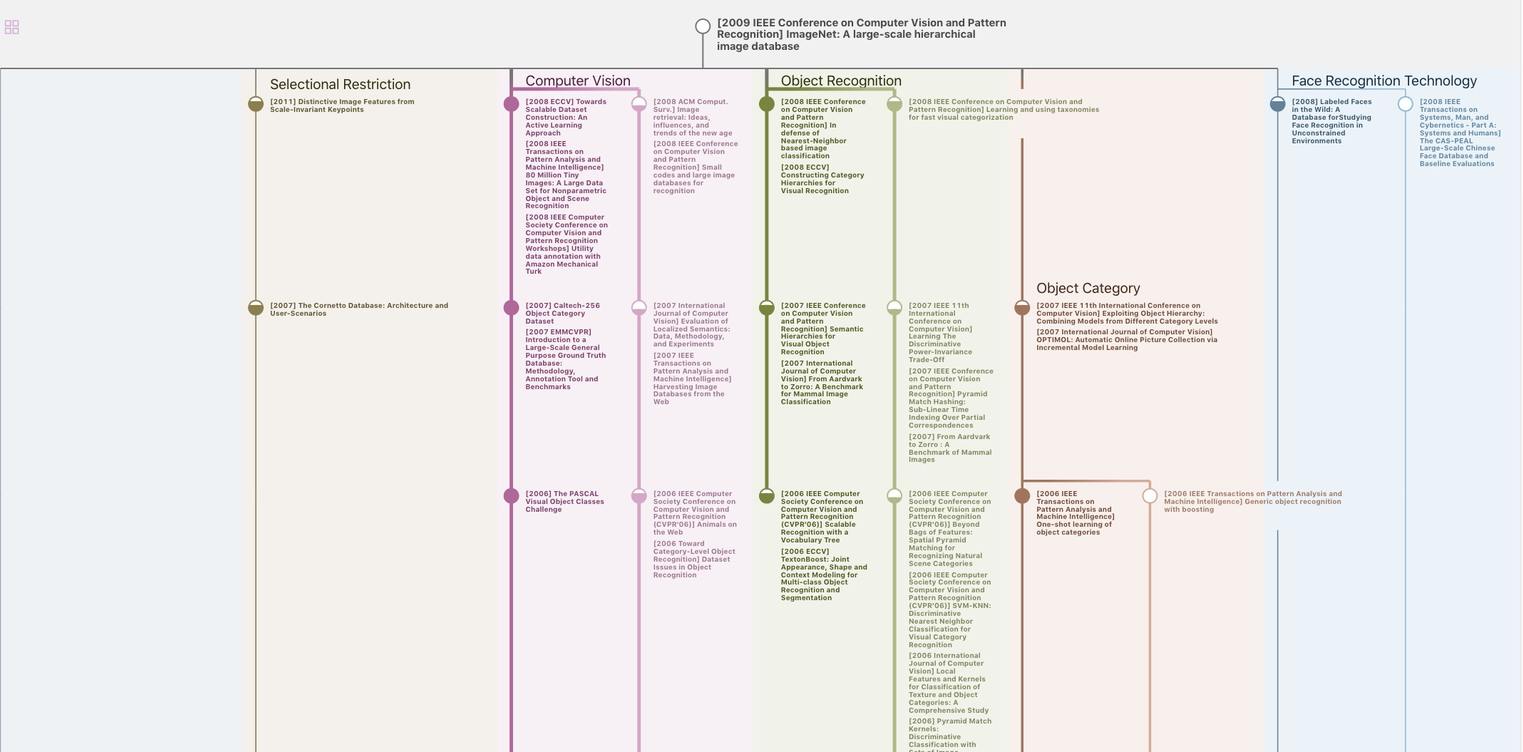
生成溯源树,研究论文发展脉络
Chat Paper
正在生成论文摘要