Knowledge-Infused Self Attention Transformers
arXiv (Cornell University)(2023)
摘要
Transformer-based language models have achieved impressive success in various natural language processing tasks due to their ability to capture complex dependencies and contextual information using self-attention mechanisms. However, they are not without limitations. These limitations include hallucinations, where they produce incorrect outputs with high confidence, and alignment issues, where they generate unhelpful and unsafe outputs for human users. These limitations stem from the absence of implicit and missing context in the data alone. To address this, researchers have explored augmenting these models with external knowledge from knowledge graphs to provide the necessary additional context. However, the ad-hoc nature of existing methods makes it difficult to properly analyze the effects of knowledge infusion on the many moving parts or components of a transformer. This paper introduces a systematic method for infusing knowledge into different components of a transformer-based model. A modular framework is proposed to identify specific components within the transformer architecture, such as the self-attention mechanism, encoder layers, or the input embedding layer, where knowledge infusion can be applied. Additionally, extensive experiments are conducted on the General Language Understanding Evaluation (GLUE) benchmark tasks, and the findings are reported. This systematic approach aims to facilitate more principled approaches to incorporating knowledge into language model architectures.
更多查看译文
关键词
attention,self,knowledge-infused
AI 理解论文
溯源树
样例
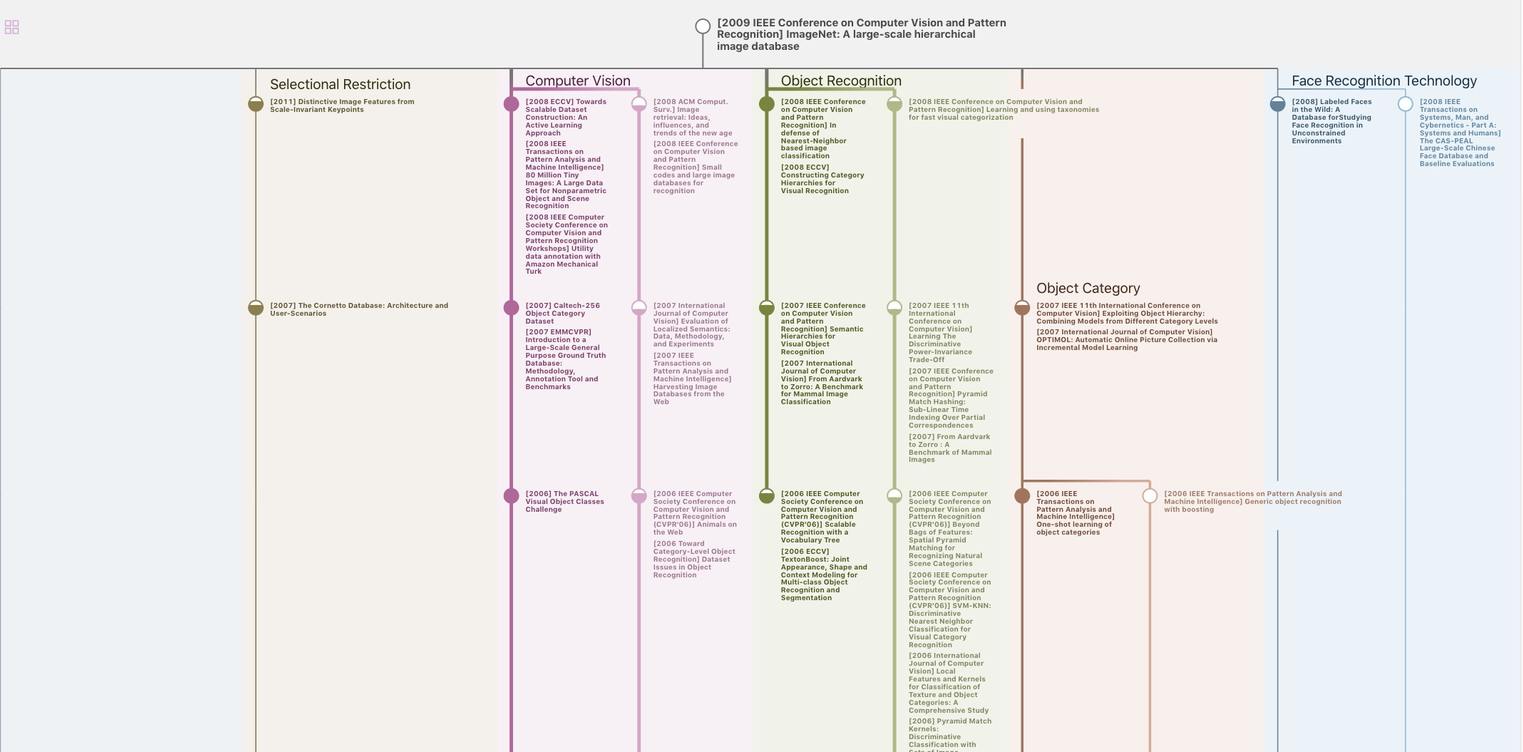
生成溯源树,研究论文发展脉络
Chat Paper
正在生成论文摘要