Identifying Signs and Symptoms of AL Amyloidosis in Electronic Health Records Using Natural Language Processing, Diagnosis Codes, and Manually Abstracted Registry Data
American journal of hematology(2023)
Abstract
AL amyloidosis, a plasma cell disorder caused by extracellular deposition of misfolded proteins, is a rare disease with an estimated incidence of 12 to 14 cases per million person-years.1 Patients with early-stage disease have a relatively high survival rate, with nearly 80% of patients alive 5 years after diagnosis.2 However, delayed diagnosis reduces the survival rate substantially; patients diagnosed at stage IIIB have 5-year overall survival of about 10%.3 Early diagnosis of AL amyloidosis is often delayed due to the non-specificity of early symptoms and rarity of the condition.4 In a retrospective study of ~1500 patients with newly diagnosed AL amyloidosis, the median time from sign or symptom onset to diagnosis was 2.7 years.5 Electronic health records (EHRs) present an avenue for identifying patients with suspected AL amyloidosis based on their symptoms, which could enable earlier diagnosis and treatment. EHRs are especially useful for investigating rare diseases because of difficulties in recruiting sufficient patients for prospective clinical trials. EHRs contain a wealth of clinical insight across the structured tables (e.g., diagnosis codes, medications, and laboratory results) and unstructured free text (e.g., admission/discharge summaries, physician notes, and descriptions of conditions), which can be used collectively to identify early indications. The goal of this study was to compare three different methods for identifying 15 signs and symptoms of AL amyloidosis from EHRs in a study population of 1223 patients with biopsy-confirmed systemic AL amyloidosis diagnosis. Patients were diagnosed between January 1, 2010, and August 31, 2019, according to a research registry from Mayo Clinic Rochester, and had research authorization available. If patients had no data in the Mayo EHR within 90 days of their diagnosis date, they were excluded from the analysis. Demographics and clinical characteristics of the study population are summarized in Table S1. We considered 15 signs and symptoms: ascites, atrial fibrillation or flutter, autonomic neuropathy, carpal tunnel, congestive heart failure, dyspnea, edema, fatigue, lightheadedness, proteinuria, orthostatic hypotension, paresthesia, pericardial effusion, peripheral neuropathy, and pleural effusion. These were selected because they are characteristic of AL amyloidosis, present in the registry, and relatively common in patients (>3% prevalence). We considered signs and symptoms around the time of diagnosis since longitudinal data were not available for many patients, and we did not consider post-diagnosis signs and symptoms since they could be secondary to treatment. Three data sources were used for identifying signs and symptoms: (1) a manually curated registry, (2) structured diagnosis codes, and (3) unstructured clinical notes curated with a natural language processing (NLP) algorithm. The registry was made by the abstraction of signs and symptoms from the EHR's unstructured notes. Conditions not attributed to AL amyloidosis were not entered into the registry, and only signs and symptoms recorded in the registry prior to initiation of treatment were considered in this analysis. International Classification of Disease (ICD)-9-CM and ICD-10-CM diagnosis codes from a structured table in the EHR provided another data source. Lists of codes were generated and reviewed for clinical relevance by the hematologist (A. Dispenzieri) who trained the data abstractors who created the registry (Table S2). The notes, which we automatically curated with a neural network-based NLP algorithm, were the third data source.6 The algorithm classifies a sign/symptom synonym and its surrounding text fragment with one of the following labels: “Yes”-confirmed, “No”-ruled-out, “Maybe”-suspected, or “Other”-alternate context (e.g., family history of sign or symptom; Figure S1).6 This data source is referred to as “augmented curation”. Lists of synonyms for each sign and symptom (Table S3) were curated with input from the hematologist (A. Dispenzieri) to ensure alignment with categories in the registry. Synonyms classified with a “Yes” sentiment were counted as a record, while other classifications were not. ICD codes and notes timestamped 1 year before to 90 days after AL amyloidosis diagnosis and prior to initiation of treatment were considered. For a patient to be counted as having a sign/symptom according to a given data source, the patient needed at least one record of the sign/symptom in that data source. The number of cases identified from each data source and the overlap across data sources are reflected in Euler diagrams (Figure 1). Congestive heart failure (38.5%) and pleural effusion (32.3%) had the highest levels of concordance across all data sources (Figure 1 and Table S4). Lightheadedness (3.8%), atrial fibrillation/flutter (5.3%), and paresthesia (8.6%) had the lowest concordance across the data sources. There was relatively high concordance between augmented curation and the registry for the most prevalent signs and symptoms: edema (520/876 patients; 59.4%), dyspnea (375/770 patients; 48.7%), fatigue (307/786 patients; 39.1%), and proteinuria (230/712 patients; 32.3%). Relatively few of these cases were also captured by ICD codes (Table S4). We evaluated the accuracy of each data source for proteinuria by deriving a “gold standard” patient set based on laboratory data. For this, we considered all patients with at least one laboratory measurement for urine protein occurring 1 year before to 90 days after the AL amyloidosis diagnosis date and prior to initiation of treatment, followed by a clinical note within 0 to 15 days, which was 974 patients (of 1223 in the study population). Individuals who had at least one measurement ≥0.5 grams of urine protein/24 hours during the study period were counted as positive for laboratory test-derived proteinuria, which was 423 patients. Using this “gold standard” patient set, we computed specificity, sensitivity, positive predictive value (PPV), and negative predictive value (NPV) metrics for patient sets identified by each data source. Augmented curation and registry yielded similar results in terms of specificity (67.2% and 66.4%, respectively), sensitivity (73.2% and 75.1%, respectively), PPV (76.5% and 76.5%, respectively), and NPV (63.2% and 64.6%, respectively; Table S5). ICD codes had higher specificity (91.9%) and PPV (85.3%), but substantially lower sensitivity (32.2%) and NPV (48.1%), affirming that ICD codes miss many true positive cases. For each of the signs and symptoms, we further investigated a random sample of 10 cases (150 cases in total) identified by augmented curation alone. We manually reviewed all notes containing a mention of the sign/symptom in the observation window, and then assigned one of the following labels for each patient-symptom pair: “Present, attributed to AL amyloidosis”, “Present, attributed to another condition/treatment”, “Present, no attribution”, or “Not present”. Of the 150 cases reviewed, the symptom was confirmed to be present in 141, while 9 were false positives (Figure S2). Of the 141 cases, the symptom was not attributed to any condition in 90, attributed to another condition or treatment in 25, and attributed to AL amyloidosis in 26. Symptoms most commonly attributed to conditions other than AL amyloidosis included peripheral neuropathy (5), proteinuria (4), congestive heart failure (3), and dyspnea (3). Peripheral neuropathy was attributed to diabetes, trauma/overuse from long-distance running, and vincristine. Proteinuria was attributed to chronic kidney disease, glomerulonephritis, and diuretics. Congestive heart failure was attributed to heart attack, mild hypertension, hyperlipidemia, and as an adverse event of pomalidomide for treating multiple myeloma. Dyspnea was attributed to cerebrovascular disease, depression, fatigue, and promethazine and fentanyl for treating abdominal pain. Overall, augmented curation was highly accurate in identifying conditions experienced by patients, but these conditions were often not explicitly linked to AL amyloidosis in the clinical notes. Alternatively, the registry dataset was manually curated to include signs and symptoms attributed to AL amyloidosis exclusively. To develop screening algorithms that facilitate earlier diagnosis, symptoms identified via augmented curation may be more useful, since they capture symptoms noted at their earliest manifestation and do not rely upon a suspected diagnosis of AL amyloidosis from the clinician. These findings demonstrate that an NLP-based approach is valuable for the comprehensive capture of signs and symptoms of AL amyloidosis from EHRs. The NLP-based method matches the quality of manual curation, but it is significantly more time-efficient and cost-effective. This analysis had several limitations. First, the lists of synonyms and ICD codes may not fully capture all terms and codes used to record the signs and symptoms. Second, this analysis only considered EHR data from a single healthcare system, and further validation studies are needed to determine if these NLP algorithms can be directly used in other healthcare systems. Going forward, an NLP method for identifying signs and symptoms from clinical notes could be integrated as part of an AL amyloidosis screening / early identification tool. These tools could reduce the time between the initial presentation of AL amyloidosis to treatment of the disease. Medical writing and editorial support were provided by Lisa Shannon, PharmD, of Lumanity Communications Inc., and were funded by Janssen Global Services, LLC. This analysis was sponsored by Janssen Research & Development, LLC. ES, CP, and VS are employees of nference and have financial interests in the company. LH, BK, SK, NT, and NK are employees of Janssen R&D, LLC. ER was an employee of nference at the time of the study. FB has nothing to disclose. EM received honorarium from Janssen and consultation fees from Protego (fee paid to institution). MG reports personal fees from Ionis/Akcea, Prothena, Sanofi, Janssen, Aptitude Healthgrants, Ashfield, Juno, Physicians Education Resource, AbbVie (for Data Safety Monitoring board), Johnson & Johnson, Celgene, Research to Practice, and Sorrento; and development of educational materials for i3Health. AD served on an advisory board and independent review committee for Janssen, served on a data monitoring safety committee for Oncopeptides and Sorrento and received research funding from Alnylam, Pfizer, Takeda, and Bristol Myers Squibb. The data sharing policy of Janssen Pharmaceutical Companies of Johnson & Johnson is available at https://www.janssen.com/clinical-trials/transparency. These data were made available by Mayo Clinic for the current study and are not publicly available due to the inclusion of protected health information (PHI). To request data from this study, researchers should contact the corresponding author and follow Mayo Clinic's standard IRB process for such requests. Table S1. Patient demographics and clinical characteristics. Table S2. ICD-9 and ICD-10 diagnosis codes used to identify signs and symptoms of AL amyloidosis. Table S3. Synonyms used for identifying signs and symptoms of AL amyloidosis in the clinical notes. Table S4. Prevalence counts and proportions of AL amyloidosis signs and symptoms across the registry, ICD codes, and augmented curation of clinical notes data sources, along with intersections. Table S5. Performance metrics of proteinuria diagnoses from augmented curation, manual abstraction of the EHR registry, and ICD codes compared to laboratory test-derived “gold standard.” For each data source, we define TP as the number of patients with proteinuria based on both laboratory tests and the data source, TN as the number of patients without proteinuria based on both laboratory tests and the data source, FN as the number of patients with proteinuria based on laboratory tests but not based on the data source, and FP as the number of patients with proteinuria based on the data source but not based on laboratory tests. Figure S1. Overview of the study design. (A) Illustration of the inclusion criteria for the study population. (B) Description of the three different sign and symptom extraction methods, along with the time windows considered for each extraction method. (C) Summary of the comparison and evaluation of the three data extraction methods. Figure S2. Stacked horizontal bar chart depicting results of a manual review of signs and symptoms identified exclusively by augmented curation of clinical notes. For each sign or symptom, we show the manual review results from 10 randomly selected patients who had the sign/symptom determined by the augmented curation method but not by ICD codes or registry data sources. Please note: The publisher is not responsible for the content or functionality of any supporting information supplied by the authors. Any queries (other than missing content) should be directed to the corresponding author for the article.
MoreTranslated text
AI Read Science
Must-Reading Tree
Example
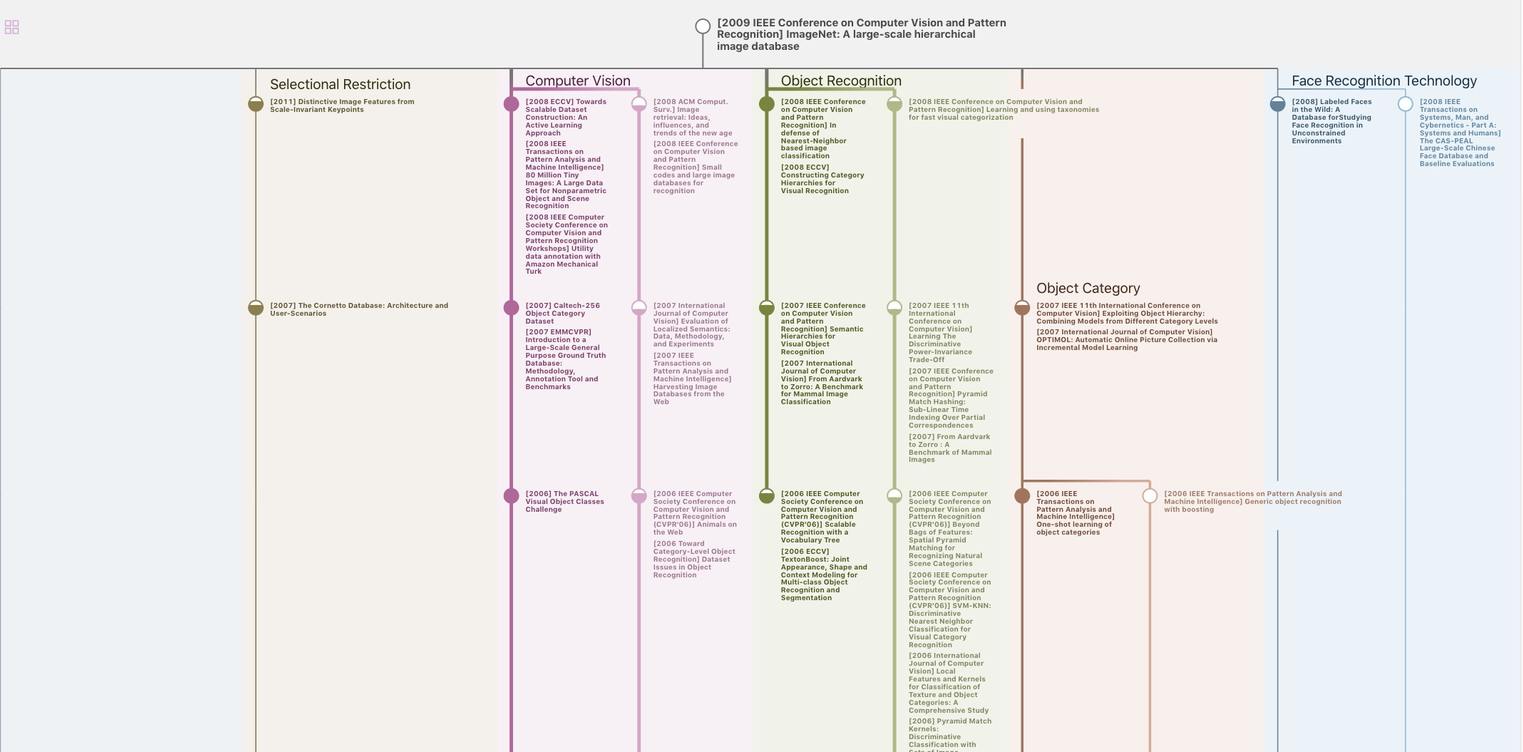
Generate MRT to find the research sequence of this paper
Chat Paper
Summary is being generated by the instructions you defined