Comparing Weighting Algorithms for Anthropometric Datasets to Enable the Generation of Representative Digital Human Models
Lecture notes in networks and systems(2023)
摘要
Digital human models (DHM) can support the virtual ergonomic design and evaluation of products and work systems, but in order to obtain meaningful results, the relevant anthropometric characteristics of the defined target population need to be considered. Hence, a representative and up-to-date detailed anthropometric dataset of the target population is required as a source of information for the appropriate scaling of the DHM. Weighting algorithms can be used to generate such data. The goal of this study was to compare and evaluate the one nearest neighbor (1NN) approach and the iterative proportional fitting (IPF) algorithm. Data from Germany were used for this study. In both weighting algorithms, the general idea is to weight a non-representative but detailed dataset with a representative reference dataset, using the few available anthropometric measures from the reference dataset as weighting parameters. To quantify the performance of 1NN and IPF several evaluation parameters were defined. Absolute percent errors were calculated from the 1st percentile (P1) to the 99th percentile (P99). Moreover, covariance matrices and multivariate computed total accommodation (TA) values were compared. Results show that neither 1NN nor IPF clearly outperformed the other algorithm across all evaluation parameters. IPF achieves better values for percentiles at the distribution edges (≤P5 & ≥P95). 1NN performs better for the multivariate evaluation parameters. However, the extent to which these results can be generalized needs to be investigated.
更多查看译文
关键词
anthropometric datasets,weighting algorithms,models,human
AI 理解论文
溯源树
样例
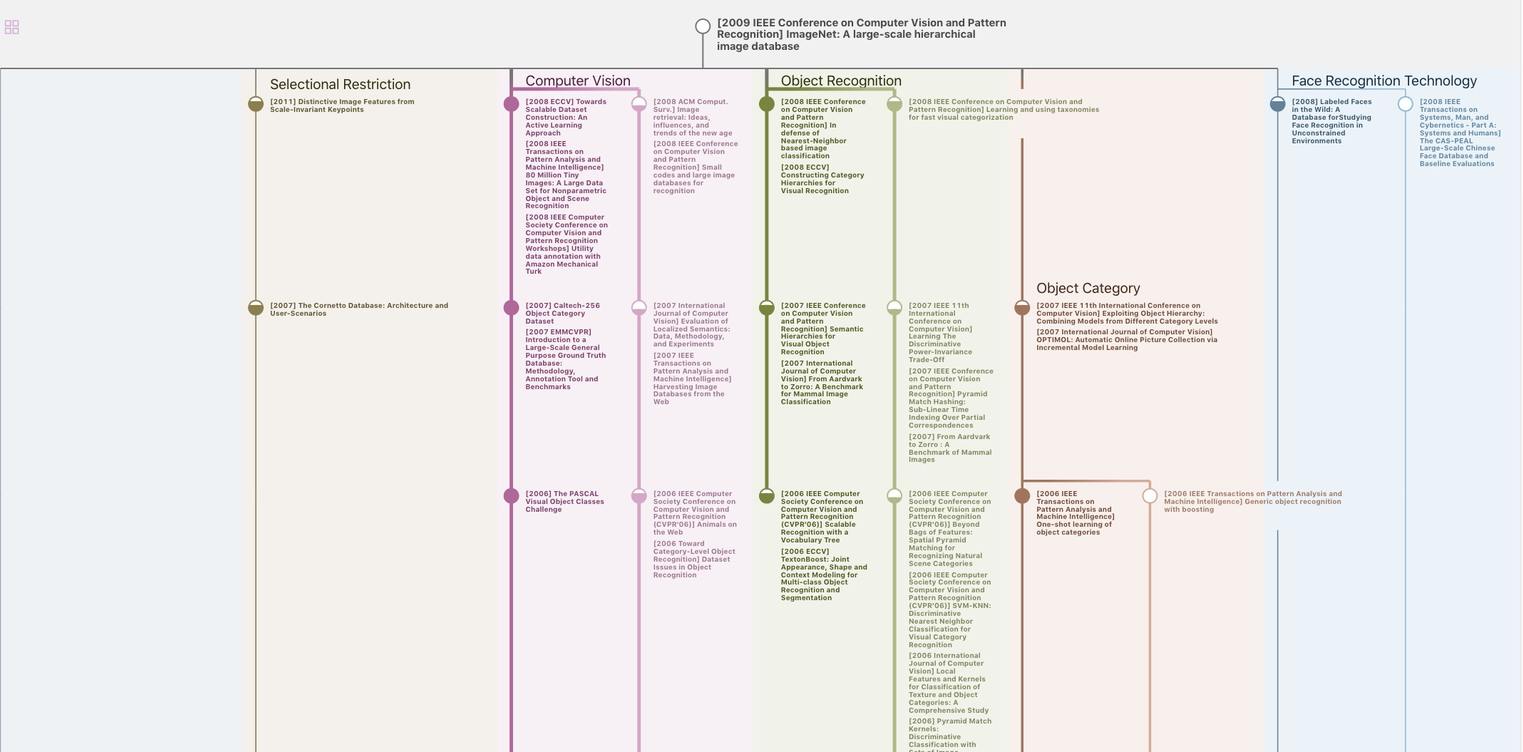
生成溯源树,研究论文发展脉络
Chat Paper
正在生成论文摘要