W 2 GAN: Importance Weight and Wavelet feature guided Image-to-Image translation under limited data
Computers & Graphics(2023)
摘要
Image-to-Image (I2I) translation methods based on generative adversarial networks (GANs) require large amounts of training data, without which they will suffer from over-fitting and train divergence, and trained models are sub-optimal. In addition, it would be very difficult for the model to synthesize high-frequency signals, deteriorating the synthesis quality. To address these, this paper proposes W2GAN, which mainly introduces the ideas of the Importance Weight and Wavelet transformation to achieve the I2I translation trained on limited-data. Concretely, this paper first alleviates the over-fitting and train divergence by the adversarial loss with importance weight, which aims to improve the influence of the high-quality generated images during the training generator, thus enhancing the generator to deceive the discriminator. Then, the high-frequency features of the wavelet transformation are applied to the decoder, and wavelet -AdaIN normalization is proposed to prevent deficiency of high-frequency information, which adaptively integrates high-frequency statistical characteristics from generated features and real image high-frequency information. Qualitative and quantitative results on the AFHQ and CelebA-HQ datasets demonstrate the merits of the W2GAN. Noticeably, this paper achieves state-of-the-art FID and KID on AFHQ and CelebA-HQ datasets.
更多查看译文
关键词
wavelet feature,<mmlmath xmlnsmml=http//wwww3org/1998/math/mathml,altimg=si6svg><mmlmsup><mmlmrow><mmlmtext>w</mmlmtext></mmlmrow><mmlmrow><mmlmn>2</mmlmn></mmlmrow></mmlmsup></mmlmath>gan,image-to-image
AI 理解论文
溯源树
样例
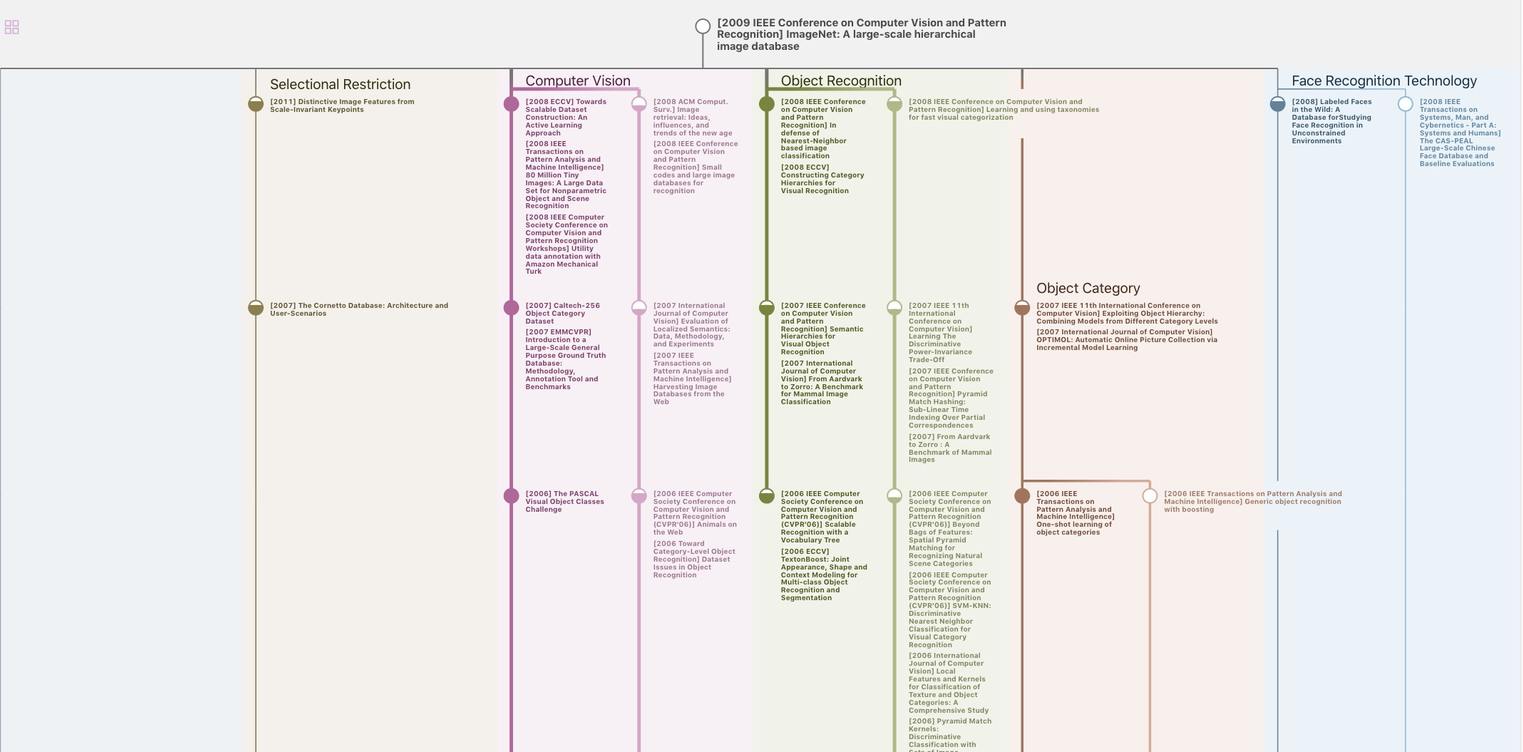
生成溯源树,研究论文发展脉络
Chat Paper
正在生成论文摘要