Analysis of the Factors Influencing the Predictive Learning Performance Using Federated Learning
Research Square (Research Square)(2023)
摘要
Abstract Numerous educational institutions utilize data mining techniques to manage student records, particularly those related to academic achievements , which are essential in improving learning experiences and overall 1 Springer Nature 2021 L A T E X template outcomes. Educational Data Mining (EDM) is a thriving research field that employs data mining and machine learning methods to extract valuable insights from educational databases, primarily focused on predicting students’ academic performance. This study proposes a novel Federated Learning (FL) standard that ensures the confidentiality of the dataset and allows predicting student grades, categorized into four levels: Low, Good, Average, and Drop. To enhance model precision, optimized features are incorporated into the training process. This study evaluates the optimized dataset using five machine learning (ML) algorithms , namely Support Vector Machine (SVM), Decision Tree, Nã A¯ve Bayes, K-Nearest Neighbours, and the proposed Federated Learning Model. The models’ performance is assessed regarding accuracy, precision , recall, and F1 score, followed by a comprehensive comparative analysis. The results reveal that FL and SVM outperform the alternative models, demonstrating superior predictive performance for student grade classification. This study showcases the potential of Federated Learning in effectively utilizing educational data from various institutes while maintaining data privacy, contributing to educational data mining and machine learning advancements for student performance prediction.
更多查看译文
关键词
federated learning,predictive learning performance
AI 理解论文
溯源树
样例
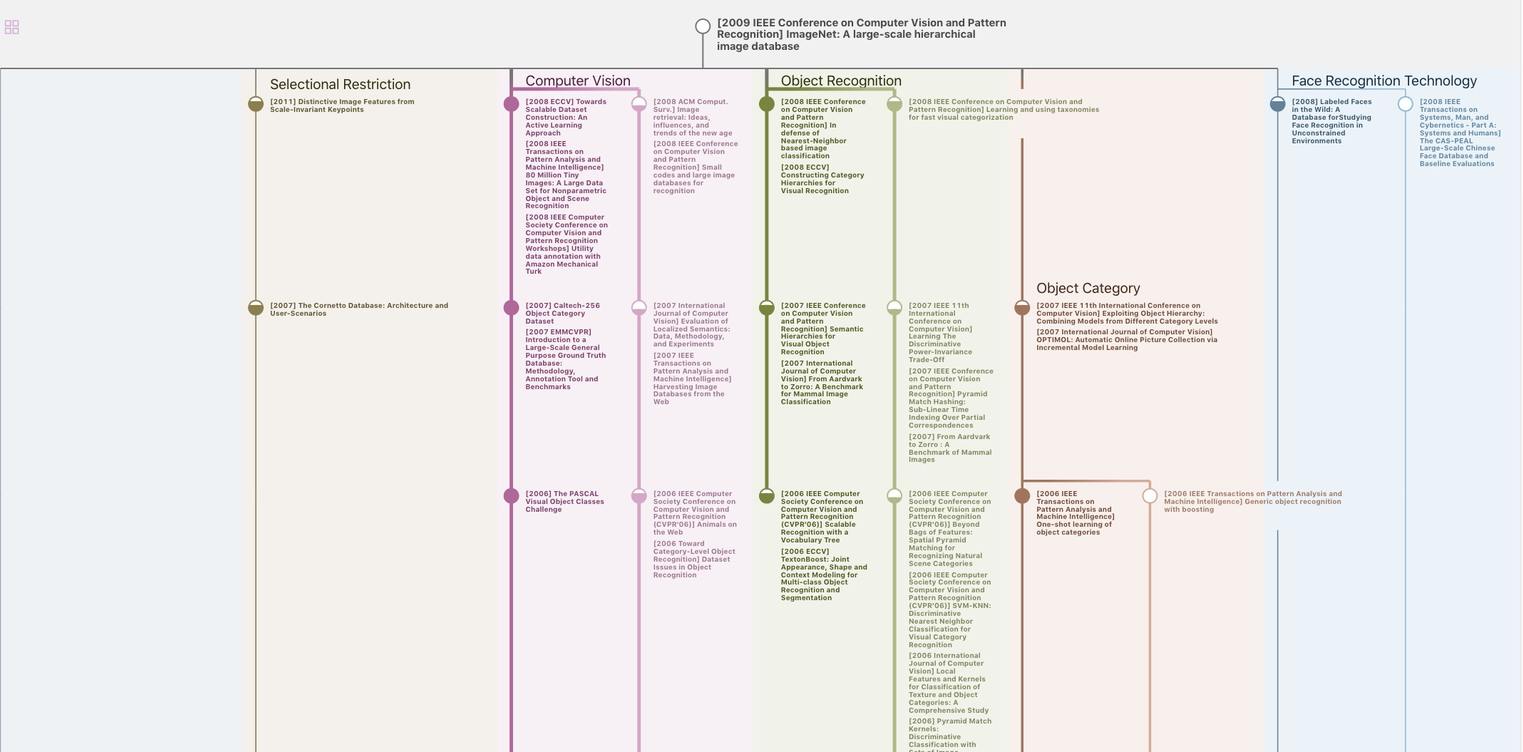
生成溯源树,研究论文发展脉络
Chat Paper
正在生成论文摘要