Calibration Methods in Imbalanced Binary Classification
Research Square (Research Square)(2023)
摘要
Abstract The calibration problem in machine learning classification tasks arises when a model's output score does not align with the ground truth observed probability. There exist several parametric and non-parametric post-processing methods that can help to calibrate an existing classifier. In this work, we focus on binary classification cases where the dataset is imbalanced, meaning that the negative class significantly outnumbers the positive one. We propose new parametric calibration methods specific to this case and a new calibration measure focusing on the primary objective in imbalanced problems: detecting infrequent positive cases. Experiments on several datasets show that, for unbalanced problems, our approaches outperform state-of-the-art methods in many cases.
更多查看译文
关键词
calibration methods,classification,binary
AI 理解论文
溯源树
样例
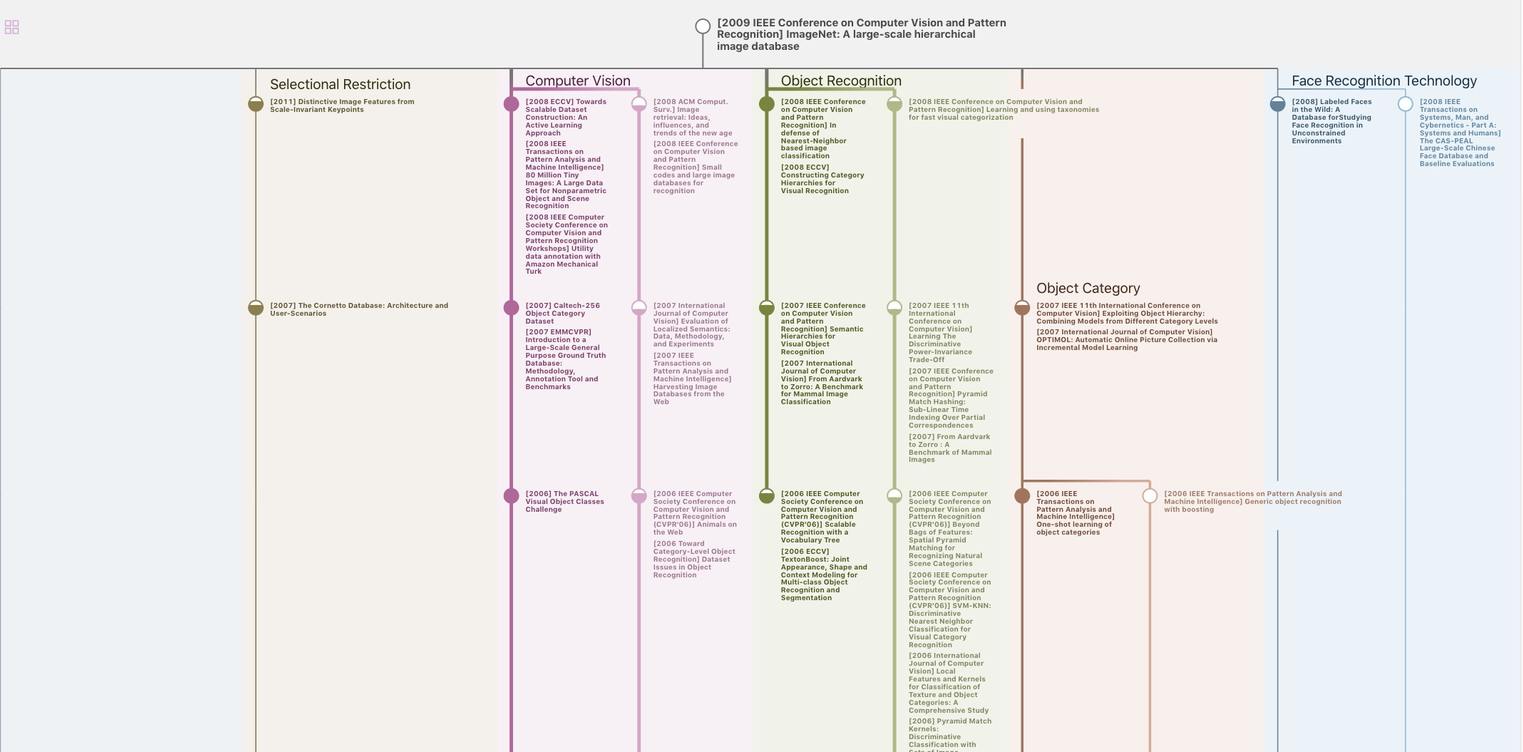
生成溯源树,研究论文发展脉络
Chat Paper
正在生成论文摘要