From Naturalistic Traffic Data to Learning-Based Driving Policy: A Sim-to-Real Study
IEEE TRANSACTIONS ON VEHICULAR TECHNOLOGY(2024)
摘要
Reinforcement learning (RL) is a promising way to achieve human-like autonomous driving (HAD) in complex and dynamic traffic, but faces challenges such as low sample efficiency, partial observability, and sim2real transfer. In light of this, a comprehensive solution for RL-driven HAD is established. First, an efficient training scheme called Deep Recurrent Q-learning from demonstration algorithm (DRQfD) is proposed for lane-changing decision-making to address the low sample efficiency in RL and the poor generalization capability in Imitation Learning (IL). The inherent LSTM structure potentially learns to predict future states of surrounding vehicles, helping to address the partially observable problem in autonomous driving (AD). Second, to reduce the sim2real gap, a twin high-fidelity simulator is built based on ROS-Gazebo for simulating LiDAR sensing, model training, and evaluations. Domain randomization is used to improve the robustness and generalization ability, making it easier for the model to be transferred to real-world scenarios. In addition, for the multi-objective optimization and imbalanced data issues in this scenario, a hierarchical decision-making framework is proposed to decompose the complex decision-making problem into several subtasks, making the driving policies easier to converge. To avoid the excessive dependence of the decision-making module on the output of perception module in modular systems, we train each modularized skill in an end-to-end manner. Moreover, we compare our method with a vanilla RL method to show improvement in learning efficiency. Comparisons between RL-based model and IL baseline in terms of safety, travel efficiency, and human-likeness are also given. To further validate the generalization ability of our model, we test the model on real traffic dataset. Finally, we implement the RL model on physical cars to demonstrate the performance of sim2real transfer.
更多查看译文
关键词
Human-like driving behavior modeling,reinforcement learning,Imitation learning,sim2real
AI 理解论文
溯源树
样例
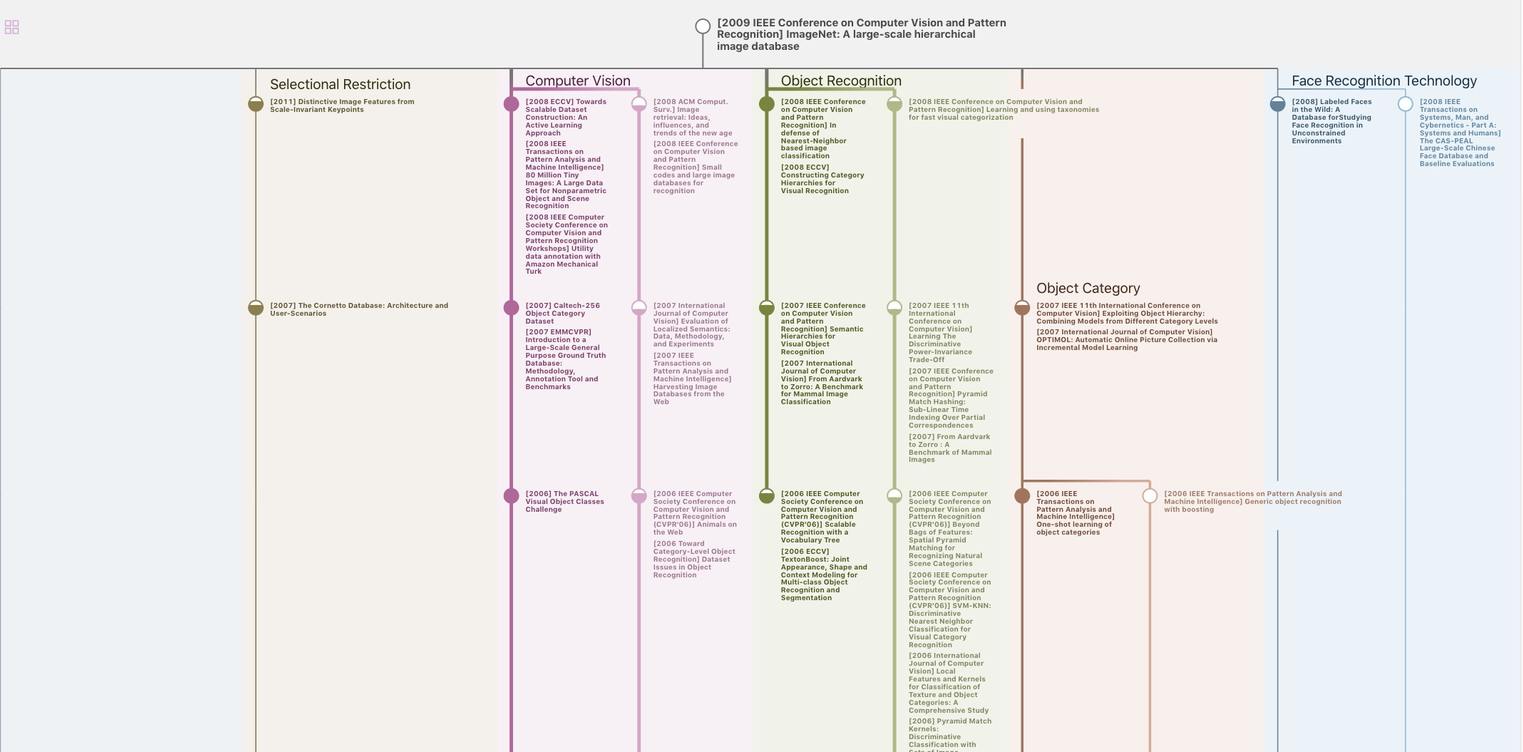
生成溯源树,研究论文发展脉络
Chat Paper
正在生成论文摘要