P1337: identification of clonal hematopoiesis driver mutations through in silico saturation mutagenesis
HemaSphere(2023)
摘要
Topic: 23. Hematopoiesis, stem cells and microenvironment Background: Clonal hematopoiesis (CH) is a common condition frequently associated with age in the human population caused by somatic mutations in hematopoietic stem cells. Mutations conferring a selective advantage lead to a clonal expansion that may eventually affect a substantial proportion of mature blood cells. CH is associated with an increased risk of hematological cancer, cardiovascular disease, and all-cause mortality. In recent years, the main CH driver genes have been characterized, but identifying which specific mutations in those genes are capable of driving CH is still an unsolved problem. Currently, the most common approach is to use manually curated rules based on experience, which are thus not standardized, hindering the detection of CH cases. Aims: Here, we aimed to identify CH driver mutations using machine learning-based models for in silico saturation mutagenesis that identify the combination of features that define CH driver mutations in a gene-specific manner. Methods: Considering that CH arises from a somatic evolutionary process similar to that of tumorigenesis, we repurposed a machine learning-based approach originally devised to learn the features of positively selected mutations in cancer. Making use of this repurposed method, so-called BoostDM-CH, we leveraged blood somatic mutations obtained from more than 36,000 individuals corresponding to three large cohorts to build gene-specific models that identify CH driver mutations. We evaluated our models in comparison with the currently used rule-based approaches using CH mutations from independent cohorts. Finally, we used BoostDM-CH to identify CH driver mutations in close to 470,000 individuals with whole-exome sequencing from the UK Biobank and validated our approach by studying the association of CH with several medical conditions. Results: We obtained reliable BoostDM-CH models for twelve genes, including the most common CH drivers DNMT3A, TET2, and ASXL1. These models provide a thorough picture of all potential driver mutations in each gene, defining the specific features that characterize them. Our models indicate that driver mutations in the different CH genes are characterized by heterogeneous feature combinations and complexity, providing a better understanding of the mechanisms leading to clonal expansion in the blood. The evaluation of our machine learning method in independent cohorts evidenced that BoostDM-CH has an accuracy comparable to currently used manually curated rules in identifying CH driver mutations. Using the large cohort from the UK Biobank, we showed that CH driver mutations identified by BoostDM-CH are highly correlated with age while non-drivers show no association (Figure 1A). Similarly, only BoostDM-CH drivers are associated with an increased risk of hematological cancer (especially myeloid neoplasms), cardiovascular diseases such as heart failure, and all-cause mortality (Figure 1B-C). These associations are particularly strong in patients with large CH clones (i.e. variant allele frequency above 10%). Our approach also allows for uncovering the effect of specific genes, or even specific groups of mutations, on the development of these diseases. Summary/Conclusion: We developed and validated gene-specific machine learning models for in silico saturation mutagenesis to identify CH driver mutations that show an accuracy comparable to state-of-the-art manually curated rules, with the advantage of being automatic and unbiased. These comprehensive blueprints may support the identification and clinical interpretation of CH mutations in newly sequenced individuals.Keywords: Clonal hematopoiesis of indeterminate potential, Age related clonal hematopoiesis, Machine learning
更多查看译文
关键词
clonal hematopoiesis driver,mutations
AI 理解论文
溯源树
样例
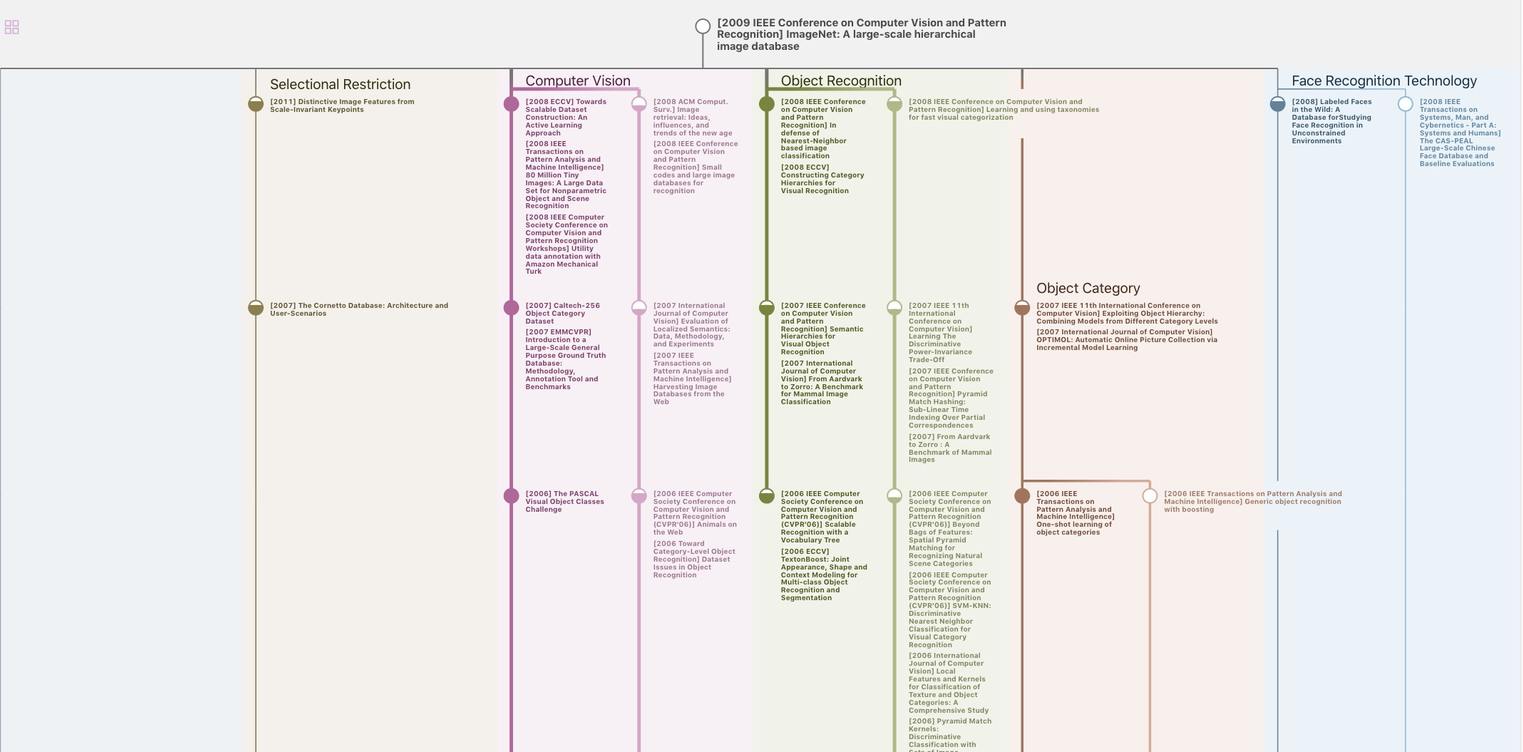
生成溯源树,研究论文发展脉络
Chat Paper
正在生成论文摘要