Predicting Students Final Academic Performance Using Deep Learning Techniques
Studies in computational intelligence(2023)
摘要
Students classification according to their potential performance in the early stages is very useful process for managing the institution resources, mitigate failure and promote the achievement for increasing the results of students. This paper proposed Student Final Academic Performance Prediction (SFAPP) System, supported by deep learning techniques that uses emotional, academic and socio-demographic information of students to predict the final performance of students. In SFAPP, end to end RNN-LSTM (Recurrent Neural Network-Long Short Term Memory) prediction model is proposed to extract the student learning time series patterns and classified the students into pass or fail according to predicted scores. The performance of proposed SFAPP system is evaluated using generated academic dataset. The superiority of proposed system is verified by comparing it with traditional deep learning and machine learning models. The prediction results illustrate that proposed SFAPP system predict the final performance of students more accurately than the traditional models by achieving 89.05% precision, 92.67% recall, 90.82% F-measure, 91.00% accuracy and 96.03% AUC rate respectively. The impact of emotional and socio-demographic information on prediction result is also measured. The results revealed that, emotional and socio-demographic information plays an important role in increasing the accuracy of student final performance prediction.
更多查看译文
关键词
students final academic performance,deep learning techniques,deep learning
AI 理解论文
溯源树
样例
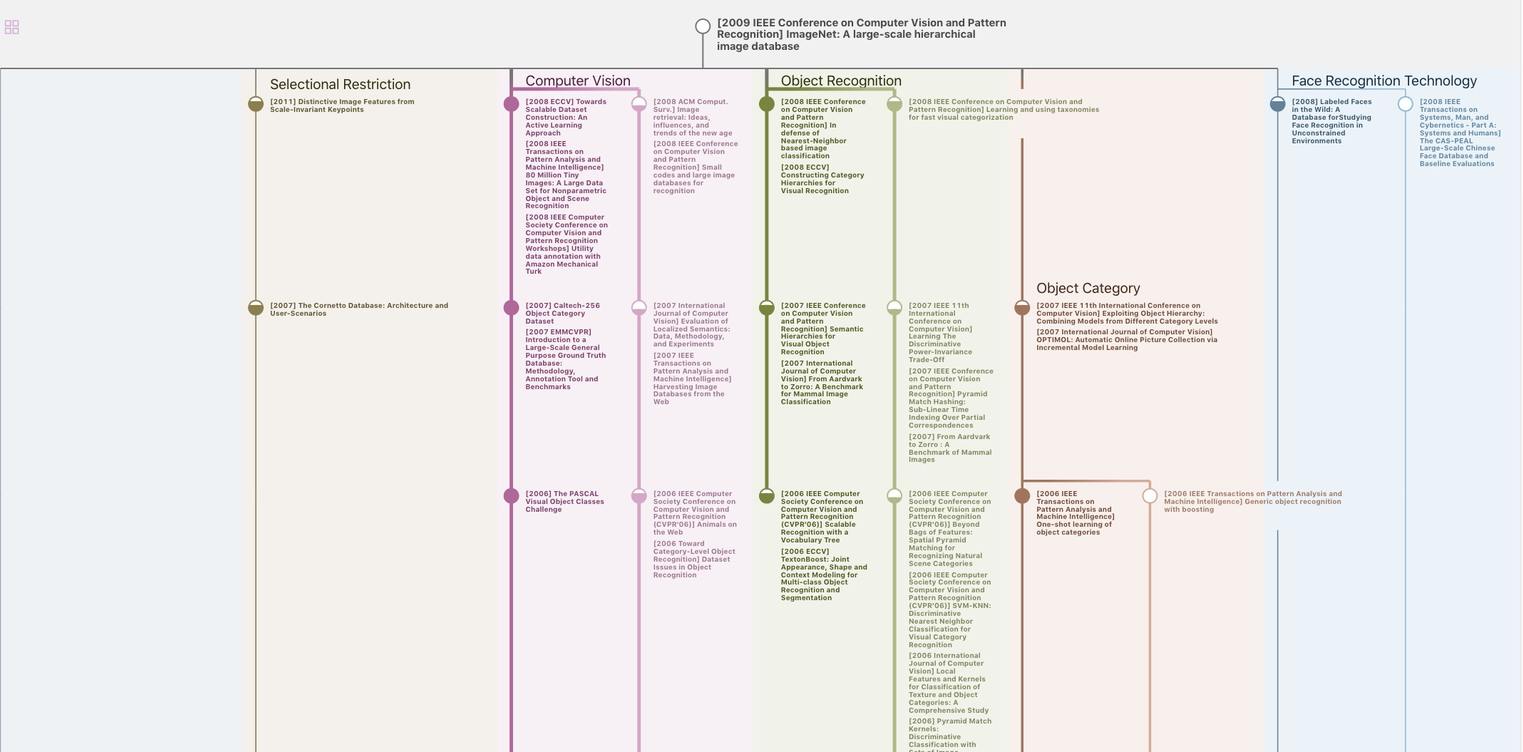
生成溯源树,研究论文发展脉络
Chat Paper
正在生成论文摘要