ATM: Action Temporality Modeling for Video Question Answering
arXiv (Cornell University)(2023)
摘要
Despite significant progress in video question answering (VideoQA), existing methods fall short of questions that require causal/temporal reasoning across frames. This can be attributed to imprecise motion representations. We introduce Action Temporality Modeling (ATM) for temporality reasoning via three-fold uniqueness: (1) rethinking the optical flow and realizing that optical flow is effective in capturing the long horizon temporality reasoning; (2) training the visual-text embedding by contrastive learning in an action-centric manner, leading to better action representations in both vision and text modalities; and (3) preventing the model from answering the question given the shuffled video in the fine-tuning stage, to avoid spurious correlation between appearance and motion and hence ensure faithful temporality reasoning. In the experiments, we show that ATM outperforms previous approaches in terms of the accuracy on multiple VideoQAs and exhibits better true temporality reasoning ability.
更多查看译文
关键词
action temporality modeling,video
AI 理解论文
溯源树
样例
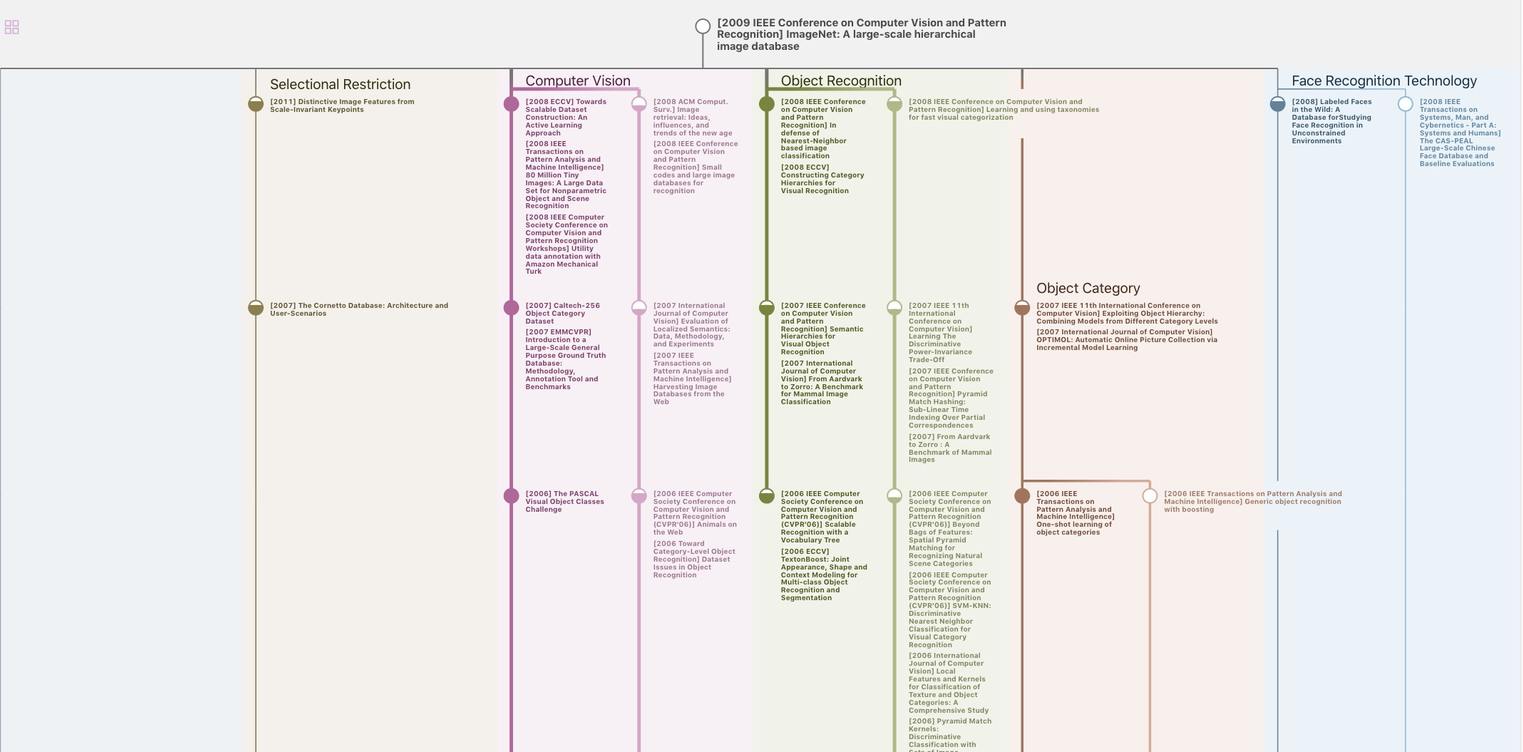
生成溯源树,研究论文发展脉络
Chat Paper
正在生成论文摘要