Deep learning tree and forest biomass from sub-meter resolution images
Research Square (Research Square)(2023)
摘要
Abstract Computational visual intelligence has been shown to be able to comprehend the content of images, which has been widely used to foster a digitized society, but is often underutilized in applications related to the green transition and climate change mitigation. Here, we evaluate the capacity of convolutional neural networks (CNN) to interpret spatial semantic patterns in optical RGB images to directly estimate forest biomass, an essential climate parameter previously assessed from structural measures of trees. Trained with forest inventory plots, the CNN model demonstrates its learning via interpreting the composition of biomass at tree level, differing from traditional approaches reliant on conversions of aggregated parameters without explanatory rationale. The CNN approach yields consistently low bias across wide biomass ranges, whereas traditional models show insufficiency without information on tree height. Visually interpretable models link advanced computational tools with the power of data, facilitating the sustainable management of resources for a carbon-neutral society.
更多查看译文
关键词
forest biomass,deep learning,tree,sub-meter
AI 理解论文
溯源树
样例
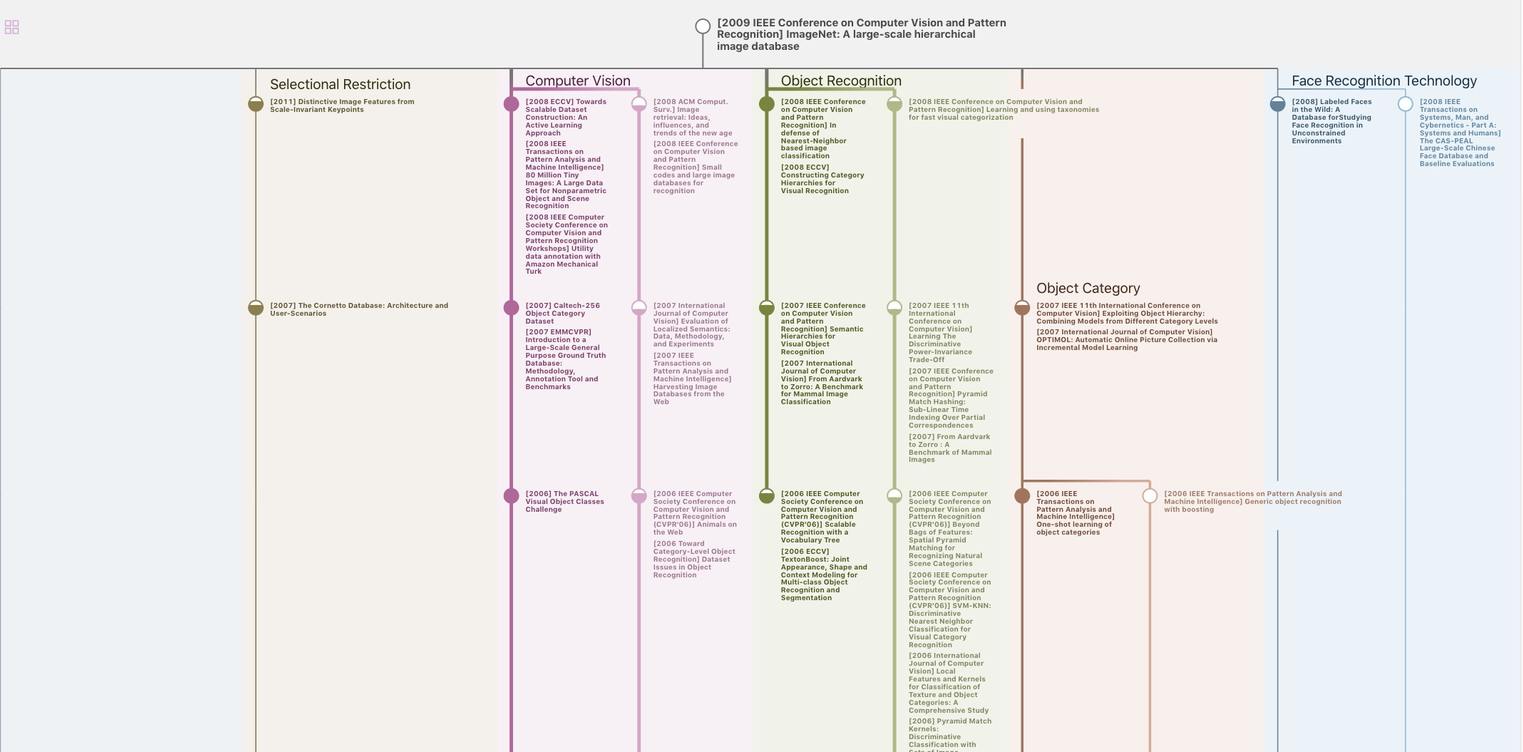
生成溯源树,研究论文发展脉络
Chat Paper
正在生成论文摘要