Abstract PO-031: Integrated bioinformatics and functional spatial analysis platform for the discovery of predictive biomarkers in oral cancer
Clinical Cancer Research(2023)
摘要
Abstract Background: Oral cavity squamous cell carcinoma (OCSCC) has an unpredictable response to immunotherapy, and standard biomarkers evaluating the tumor immune microenvironment (TIME) lack widespread translational applicability. Defining the TIME is critical to understanding mechanisms and allows the development of reproducible and accurate prognostic and predictive biomarkers based on immune profiles; however, to date, this remains elusive for clinical use. To further study and deconstruct the TIME within OCSCC, we integrated our previously developed computational spatial analysis (CSA) with in-silico deconvolution analyses to comprehensively study the TIME in OCSCC. Methods: We leveraged access to two independent OCSCC cohorts (UM and TCGA) with paired bulk RNASeq, WES, and CSA-immune score data. TMB, neoantigen load, and deconvolution analysis (CIBERSORTx) were performed to generate in silico immune profiles. A previously described CSA evaluates the density and distribution of tumor-infiltrating lymphocytes (TILs) relative to cancer cells to generate immune scores (GCross function [GFx]). GFx scores were then correlated with WES and RNASeq-derived cell-type deconvolution results, immune cell activation expression signatures, and gene expression-based radiosensitivity index scores. Pearson's correlation coefficient and associated p-value were calculated for each comparison. Cox proportional hazards multivariate regression was performed using the entire cohort for survival and recurrence analysis. Results: Here, we better characterize the immunologic tumor environment by objectively measuring the TIL density in spatial relationships with cancer cells. We found that immunoreactive tumors with high GFx scores are highly prognostic for overall survival, with the lowest tiers associated with poor survival. Combining the spatial relationship with immunogenomics (WES and RNASeq immune profiles) increased the prognostic capability of the integrated bioinformatics analysis model. In addition, we further revealed that lymphocyte-infiltrated regions, specifically CD8+ T-cells, are associated with TMB, neoantigen load, and improved overall survival. Conclusion: This study provides an integrated dataset from which to generate future insights and possible immunomodulatory therapies to counteract the poor immunogenicity of OCSCC. We show that integrating computational spatial analysis and in-silico deconvolution analysis is generalizable to multiple patient populations with different demographic compositions and pathology images collected from distinctive digitization methods. Our machine-learning approaches provide clinically actionable predictions that confirm the relevance of these biomarkers with immune cell infiltration and suggest the role of digital pathology profiling in predicting response to immunotherapy which could inform treatments for oral squamous carcinoma patients. Citation Format: Michael M. Allevato, Lisa Chionis, Joshua Smith, Santhoshi Krishnan, Leanne Henry, Tingting Qin, Janice Farlow, Laura Rozek, Jonathan Mchugh, Arvind Rao, Maureen Sartor, Steven B. Chinn. Integrated bioinformatics and functional spatial analysis platform for the discovery of predictive biomarkers in oral cancer [abstract]. In: Proceedings of the AACR-AHNS Head and Neck Cancer Conference: Innovating through Basic, Clinical, and Translational Research; 2023 Jul 7-8; Montreal, QC, Canada. Philadelphia (PA): AACR; Clin Cancer Res 2023;29(18_Suppl):Abstract nr PO-031.
更多查看译文
关键词
integrated bioinformatics,predictive biomarkers,functional spatial analysis platform
AI 理解论文
溯源树
样例
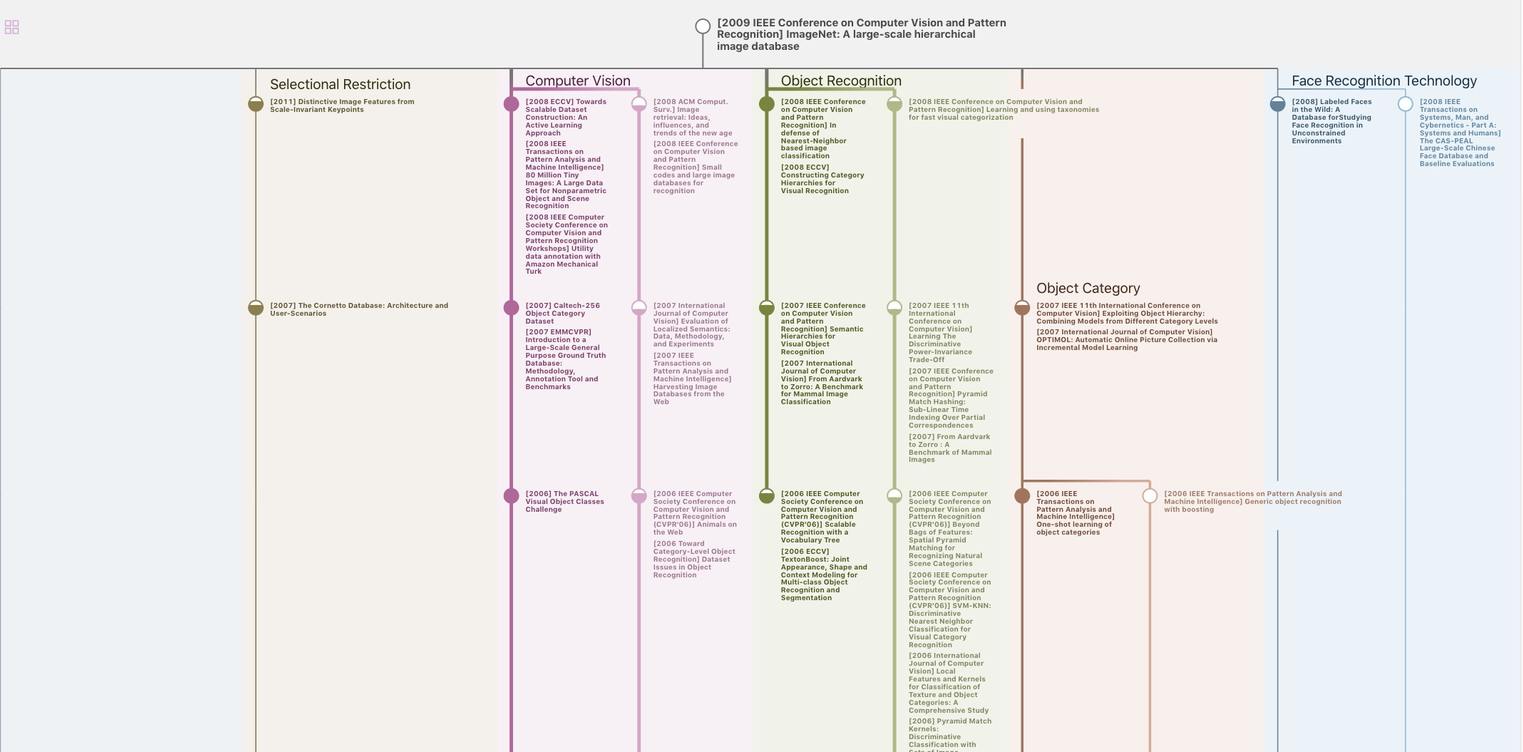
生成溯源树,研究论文发展脉络
Chat Paper
正在生成论文摘要