A Reinforcement Learning-Based Incentive Scheme for Multi-Hop Communications in Vehicular Networks.
IEEE Trans. Cogn. Commun. Netw.(2024)
摘要
Vehicular network applications heavily rely on multi-hop communication technologies to ensure efficient and reliable data transmissions because of the limited wireless transmission range of vehicles, road side units, and some other mobile devices in the network. There is a common assumption in most of the applications that all network nodes are cooperative during the entire network procedure. However, this is not practical in reality, because rational nodes are reluctant to assist others with consuming own precious resources. Hence, incentive mechanisms are inevitably required to encourage the intermediate nodes to forward data packets for multi-hop communications. Nevertheless, the high mobility of nodes and the intermittent connectivity among them make the estimation of the environment extremely challenging in vehicular networks. Moreover, in some traffic scenarios where there is a lack of fixed infrastructures (rural area, disaster areas, or during maintenance), the incentive schemes should be self-organized in a distributed manner. In this paper, we propose an incentive scheme for multi-hop communications in vehicular networks. The proposed scheme estimates the complicated dynamic environment based on a fuzzy logic approach, then further stimulates intermediate nodes to maximize the expected utility from a long-term perspective based on a reinforcement learning method in a fully distributed manner. To evaluate the performance of our proposed scheme, we conducted extensive computer simulations based on realistic traffic networks in Xi’an city of China. The results of the simulations demonstrate that the proposed scheme has the superiority of incentive capabilities over other baseline approaches in terms of packet delivery ratio, end-to-end delay within vehicular networks.
更多查看译文
关键词
Incentive schemes,vehicular networks,selfish nodes,reinforcement learning,fuzzy logic
AI 理解论文
溯源树
样例
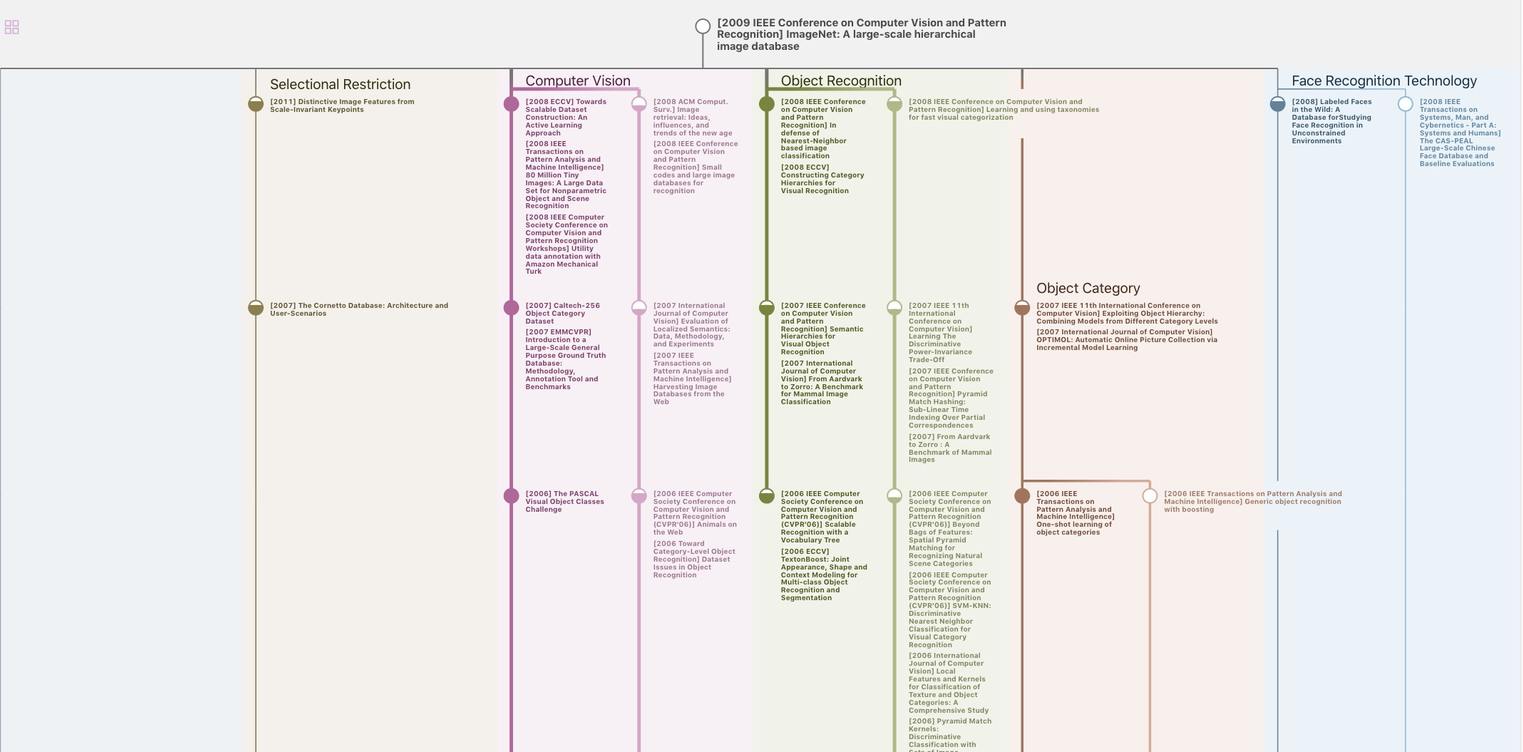
生成溯源树,研究论文发展脉络
Chat Paper
正在生成论文摘要