Anomaly Detection with Memory-augmented Adversarial Autoencoder Networks for Industry 5.0
IEEE Transactions on Consumer Electronics(2023)
摘要
CNN-based adversarial machine learning models are proposed to drive the innovation of anomaly detection techniques under Industry 5.0. However, the generalization inherent in the model is inadequate to separate anomalies from normal data with insufficient representations. It suffers from the spatial locality of CNN, resulting in inferior reconstructions due to low-quality feature representations. The intermediate features have not been well utilized, which impairs industrial anomaly detection. In this paper, we propose Memory-augmented Adversarial Autoencoder with CNN-Transformer (CTMAANet), aiming to improve the quality and utilization of features, which is conducive to the innovation of detection technology under Industry 5.0. CNN-Trans block is proposed as the backbone to inherit the advantage of both CNN and Transformer for feature extraction. Memory-augmented Adversarial Autoencoder is exploited for industrial detection by making full use of intermediate features. Specifically, a memory module with multiple measurements is designed to suppress the generator generalization by cascading multivariate features. A dual-view discriminator is presented to discriminate anomalies from both image and latent spaces. Further, a G-D latent loss enhances adversarial training by minimizing the encoding and discriminative processes. Extensive experiments conducted on numerous datasets show that the proposed model achieves effective detection under Industry 5.0.
更多查看译文
关键词
Adversarial Machine Learning,Anomaly Detection,Industry 5.0,Dual-View Discriminator,G-D Latent Loss
AI 理解论文
溯源树
样例
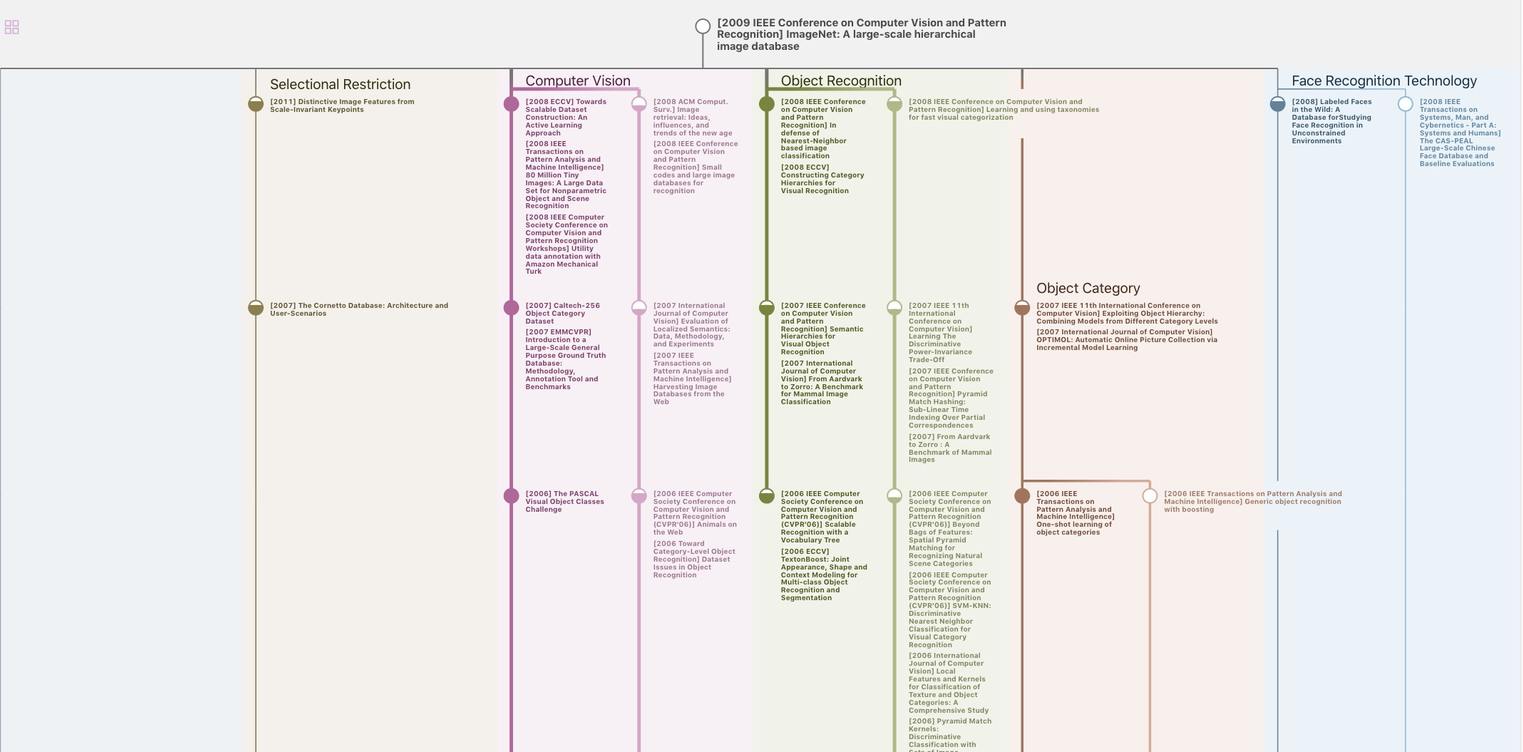
生成溯源树,研究论文发展脉络
Chat Paper
正在生成论文摘要