Tell Model Where to Attend: Improving Interpretability of Aspect-Based Sentiment Classification via Small Explanation Annotations
arXiv (Cornell University)(2023)
摘要
Gradient-based explanation methods play an important role in the field of interpreting complex deep neural networks for NLP models. However, the existing work has shown that the gradients of a model are unstable and easily manipulable, which impacts the model's reliability largely. According to our preliminary analyses, we also find the interpretability of gradient-based methods is limited for complex tasks, such as aspect-based sentiment classification (ABSC). In this paper, we propose an \textbf{I}nterpretation-\textbf{E}nhanced \textbf{G}radient-based framework for \textbf{A}BSC via a small number of explanation annotations, namely \texttt{{IEGA}}. Particularly, we first calculate the word-level saliency map based on gradients to measure the importance of the words in the sentence towards the given aspect. Then, we design a gradient correction module to enhance the model's attention on the correct parts (e.g., opinion words). Our model is model agnostic and task agnostic so that it can be integrated into the existing ABSC methods or other tasks. Comprehensive experimental results on four benchmark datasets show that our \texttt{IEGA} can improve not only the interpretability of the model but also the performance and robustness.
更多查看译文
关键词
sentiment classification,interpretability,aspect-based
AI 理解论文
溯源树
样例
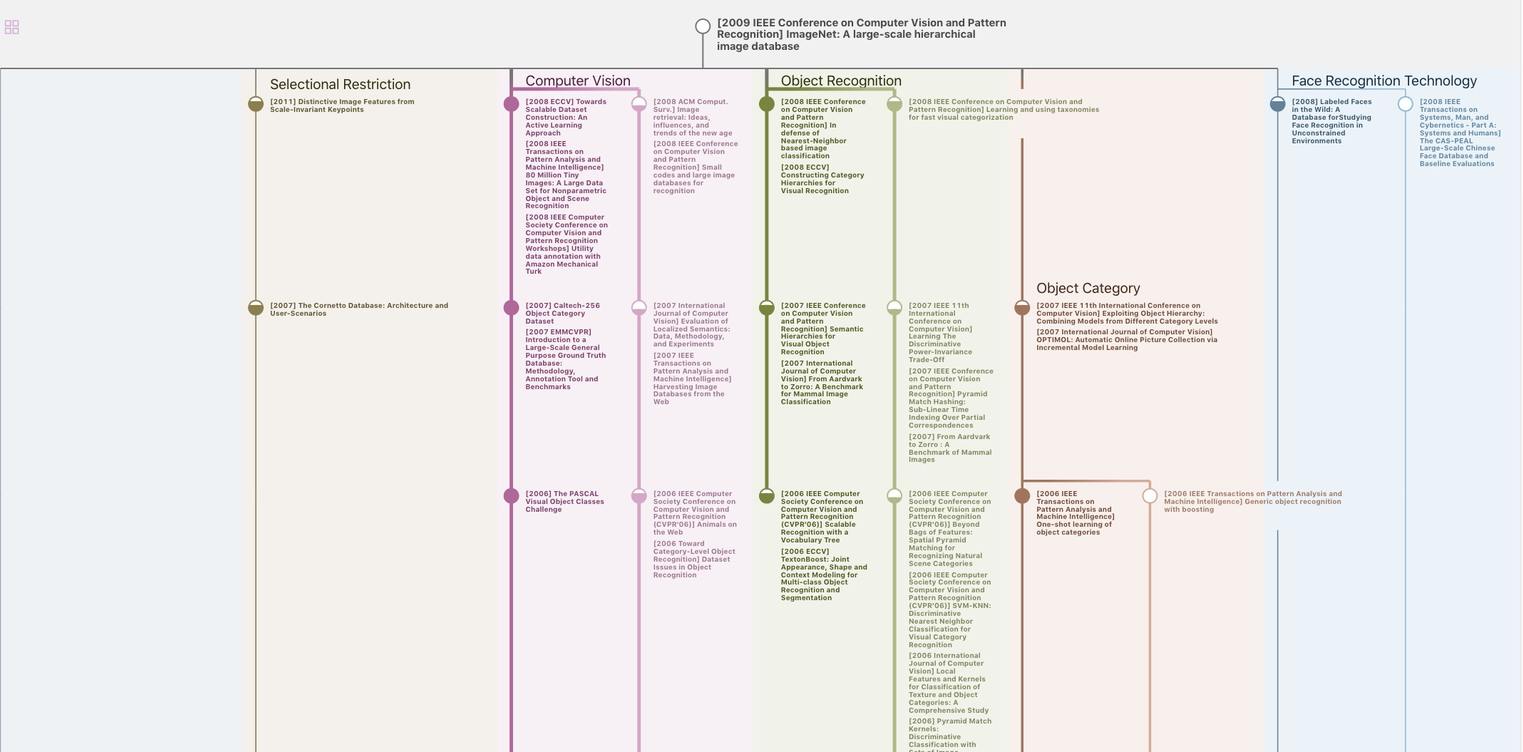
生成溯源树,研究论文发展脉络
Chat Paper
正在生成论文摘要