Iterative Decorrelation Analysis, Unit of Measure Preserving Transformations and Latent Biomarker Discovery
Research Square (Research Square)(2023)
Abstract
Abstract Background Numerous biomarker discovery studies and exploratory clinical studies extract a large set of measurable variables, which often have varying degrees of correlation among them. This data collinearity can impact statistical model interpretation and hinder the discovery of potential associations between measured variables and the observed outcome. Exploratory Factor Analysis (EFA), Principal Component Analysis (PCA), and Machine-Learning (ML) can be used to discover latent variables associated with disease progression or outcome by computing transformation matrices, but the interpretation of unsupervised/supervised latent variables in high-dimensional datasets can be challenging. Results This study describe and reports the performance of the iterative decorrelation analysis algorithm (IDeA). The algorithm iteratively analyzes the correlation matrix of the data, updating the transformation coefficients until it reaches the desired correlation goal. The output of IDeA is a basis-transformation matrix that preserves the data dimensionality and unit of measure of the original observed variables. The main advantages of the IDeA basis transformations are sparsity and interpretability. The transformation does not alter uncorrelated features, thus statistical modeling and biomarker discovery in the new transformed basis can be a combination of novel latent variables and a sizable subset of unaltered variables. The algorithm was tested on five multidimensional/hyperdimensional and multimodal sets, demonstrating the effect of decorrelation parameters, interpretability, and latent biomarker discovery. Conclusions The iterative decorrelation approach provides a simple to use tool for researchers to explore the association between correlated features in hyperdimensional/multimodal settings and to decorrelate significant associations via latent variables that preserve the unit of measurement. An efficient computer implementation of IDeA is available in the FRESA.CAD R package (https://cran.r-project.org/web/packages/FRESA.CAD/index.html).
MoreTranslated text
Key words
biomarker,measure preserving transformations
AI Read Science
Must-Reading Tree
Example
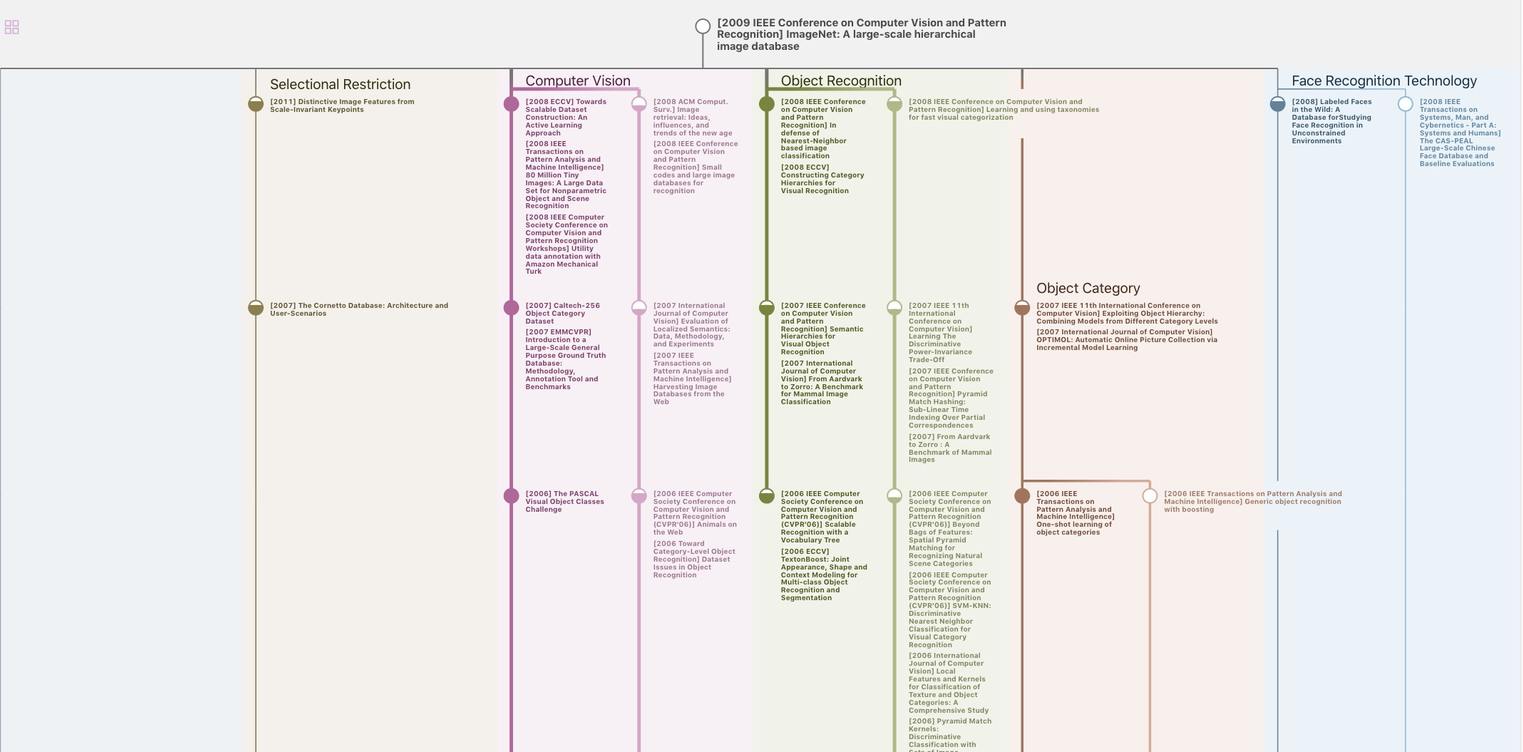
Generate MRT to find the research sequence of this paper
Chat Paper
Summary is being generated by the instructions you defined