CGMega: explainable graph neural network framework with attention mechanisms for cancer gene module dissection.
Nature communications(2024)
摘要
Cancer is rarely the straightforward consequence of an abnormality in a single gene, but rather reflects a complex interplay of many genes, represented as gene modules. Here, we leverage the recent advances of model-agnostic interpretation approach and develop CGMega, an explainable and graph attention-based deep learning framework to perform cancer gene module dissection. CGMega outperforms current approaches in cancer gene prediction, and it provides a promising approach to integrate multi-omics information. We apply CGMega to breast cancer cell line and acute myeloid leukemia (AML) patients, and we uncover the high-order gene module formed by ErbB family and tumor factors NRG1, PPM1A and DLG2. We identify 396 candidate AML genes, and observe the enrichment of either known AML genes or candidate AML genes in a single gene module. We also identify patient-specific AML genes and associated gene modules. Together, these results indicate that CGMega can be used to dissect cancer gene modules, and provide high-order mechanistic insights into cancer development and heterogeneity.
更多查看译文
关键词
cancer gene module dissection,graph,neural
AI 理解论文
溯源树
样例
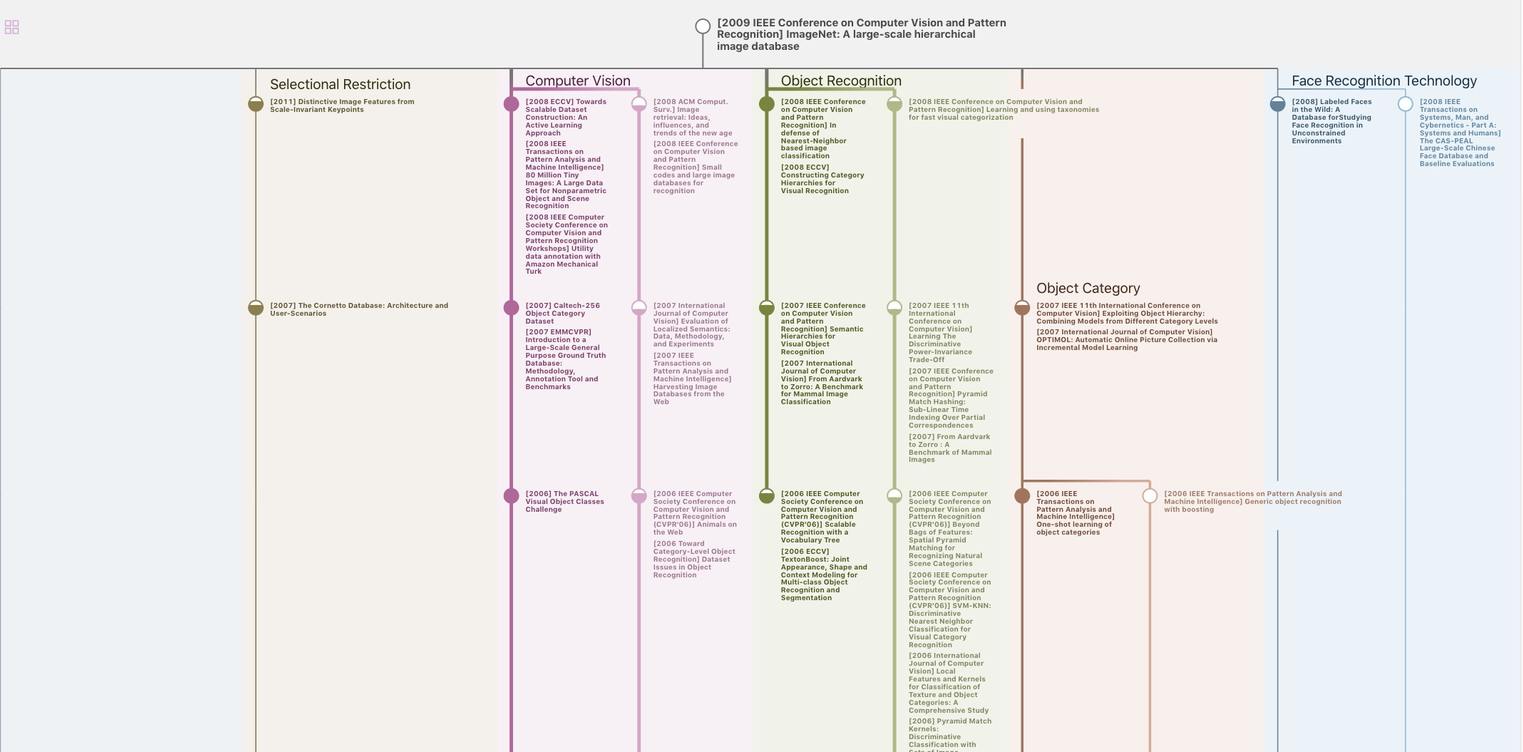
生成溯源树,研究论文发展脉络
Chat Paper
正在生成论文摘要