P533: a fully automated supervised ai-based cell classifier to accurately flag pathological signature cells in 28,285 real-world blood smears
HemaSphere(2023)
摘要
Background: Cytomorphological analysis of peripheral blood (PB) is used for pre-assessment and guidance of downstream diagnostics in a hematological routine laboratory. Artificial intelligence (AI)-based tools have shown to be effective support of human investigators in single cell classification. Aims: To expand our established blood cell classifier model to especially evaluate its capability to correctly call different pathological cell types. Methods: From 01/2021 to 07/2022, 28,285 routine diagnostic blood smears including all kinds of reactive and neoplastic conditions have been scanned and differentiated by two independent human experts (100 cells/sample). In parallel, about 500 single cells per smear were captured with a fully automated Metafer Slide Scanning System SFX80 (MetaSystems, Altlussheim; Axio Imager.Z2 microscope, Zeiss, Jena) at 40x magnification with oil, and processed by our cell classification model based on the Swin V2 transformer. Here, myeloblasts (MYBL), atypical promyelocytes (aPML) of acute promyelocytic leukemias (APL), hairy cells (HC) of hairy cell leukemias (HCL), and atypical lymphocytes (potentially neoplastic, nLYM) of lymphomas were investigated as four different classes of pathological signature cells. Results: In total 2,828,500 cells were manually differentiated, and in parallel 14,142,500 cells were processed by AI. The dataset included 2,003 cases with significant evidence of peripheral blasts (> 1% MYBL in manually differentiated PB). It furthermore included 23 APL cases, 211 HCL, and 4,882 lymphomas, all assessed at first diagnosis or in state of refractory disease. Cases with only 1% assumed MYBL in the manually differentiated PB (666 cases) were excluded from the whole analysis. Cases of HCL and lymphoma were confirmed by immunophenotyping as gold standard, APL cases by PML::RARA PCR. The 20,500 remaining cases were used as negative controls. As sensitivity is the decisive parameter for detection of rare leukemia cells, special attention was paid to it during AI training (model weights designed to emphasize recall with a factor of 5). The AI classifier detected MYBL in 1828/2003 cases (sensitivity 91%, accuracy 79%, specificity 78%), aPMBL in 21/23 cases (91%/70%/70%), HC in 111/211 cases (53%/78%/78%), and nLYM in 4312/4882 cases (88%/34%/19%). It is worth emphasizing that even experienced human investigators often cannot distinguish between reactive and neoplastic atypical lymphocytes relying on cytomorphology alone, and that in our routine workflow they were supported by results of immunophenotyping for their final cell classifications in HCL and lymphoma cases. In contrast, AI did or did not detect pathological cells without additional metadata, which explains remarkably lower accuracy in lymphomas and lower sensitivity in HCL. To further improve total prediction accuracy of the AI classifier, we focussed on cell classifications with a prediction probability of at least 0.8 (7,858,903 cells = 56% meeting the criterion). Based on that, we achieved a prediction accuracy of 92%, 95%, 96%, and 71% for MYBL, aPML, HC and for nLYM, respectively. Summary/Conclusion: An AI-based cell classifier is able to detect even rare pathological signature cells in blood smears in a very large real-world cohort and consequently can flag them for prioritized review by human experts. Through this reproducible approach, the system in many cases enables reliable diagnostic predictions while reducing human error. This tool for rapid and accurate prediction of malignant cells will be further tested and continuously developed. Keywords: Leukemia, Lymphoma, Diagnosis, Artificial intelligence
更多查看译文
关键词
cell classifier,p533,ai-based,real-world
AI 理解论文
溯源树
样例
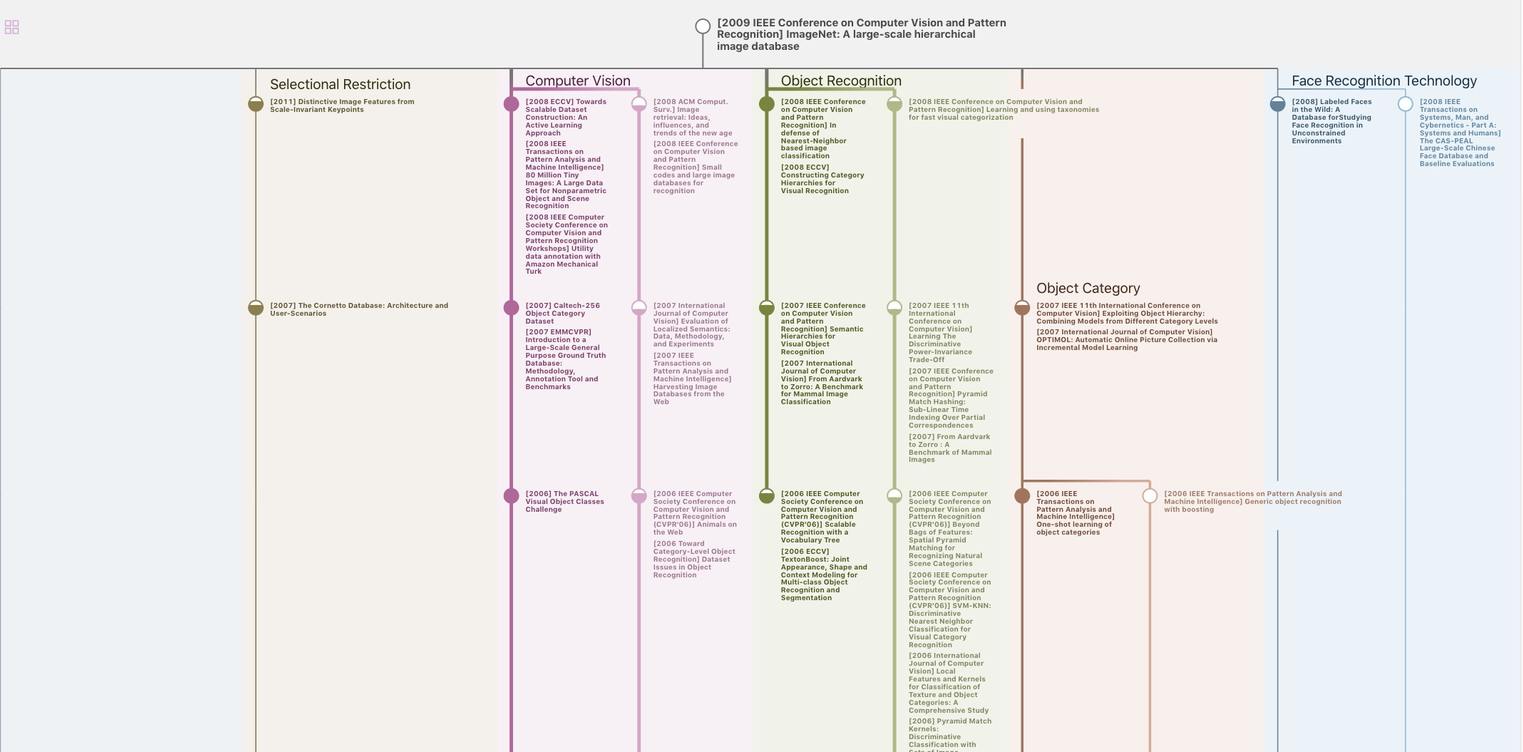
生成溯源树,研究论文发展脉络
Chat Paper
正在生成论文摘要