Bayesian and Discriminative Models for Visual Stability across Saccades
Journal of Vision(2023)
摘要
The brain interprets sensory inputs to guide behavior, but behavior itself disrupts sensory inputs. Perceiving a coherent world while acting in it constitutes active perception. For example, saccades constantly displace the retinal image and yet, we perceive a stable world. The visual system must compare the predicted sensory consequence of each saccade with the incoming sensory input to judge whether a mismatch occurred. This process is vulnerable to sensory uncertainty from two potential sources: external noise in the world (“image noise”) and internal uncertainty due to one’s own movements (“motor-driven noise”). Since Bayesian models have been influential in explaining how priors can compensate for sensory uncertainty, we tested whether they are used for visual stability across saccades. We found that non-human primates (2 rhesus macaques) used priors to compensate for internal, motor-driven noise in a Bayesian manner. For external, image noise, however, they were anti-Bayesian. Instead, they likely used a discriminative strategy, suggesting that vision across saccades is governed by both Bayesian and discriminative strategies. Next, we tested whether single neurons in the Frontal Eye Field (FEF), which receives both internal saccade-related and external visual information, support either the Bayesian or anti-Bayesian (discriminative) strategies. We found that FEF neurons predict the anti-Bayesian, but not Bayesian, behavior. Taken together, the results demonstrate that Bayesian and discriminative computations for visual stability are dissociable at the behavioral and neural levels and situate FEF along a pathway that selectively supports the discriminative contribution.
更多查看译文
关键词
visual stability,saccades,discriminative models
AI 理解论文
溯源树
样例
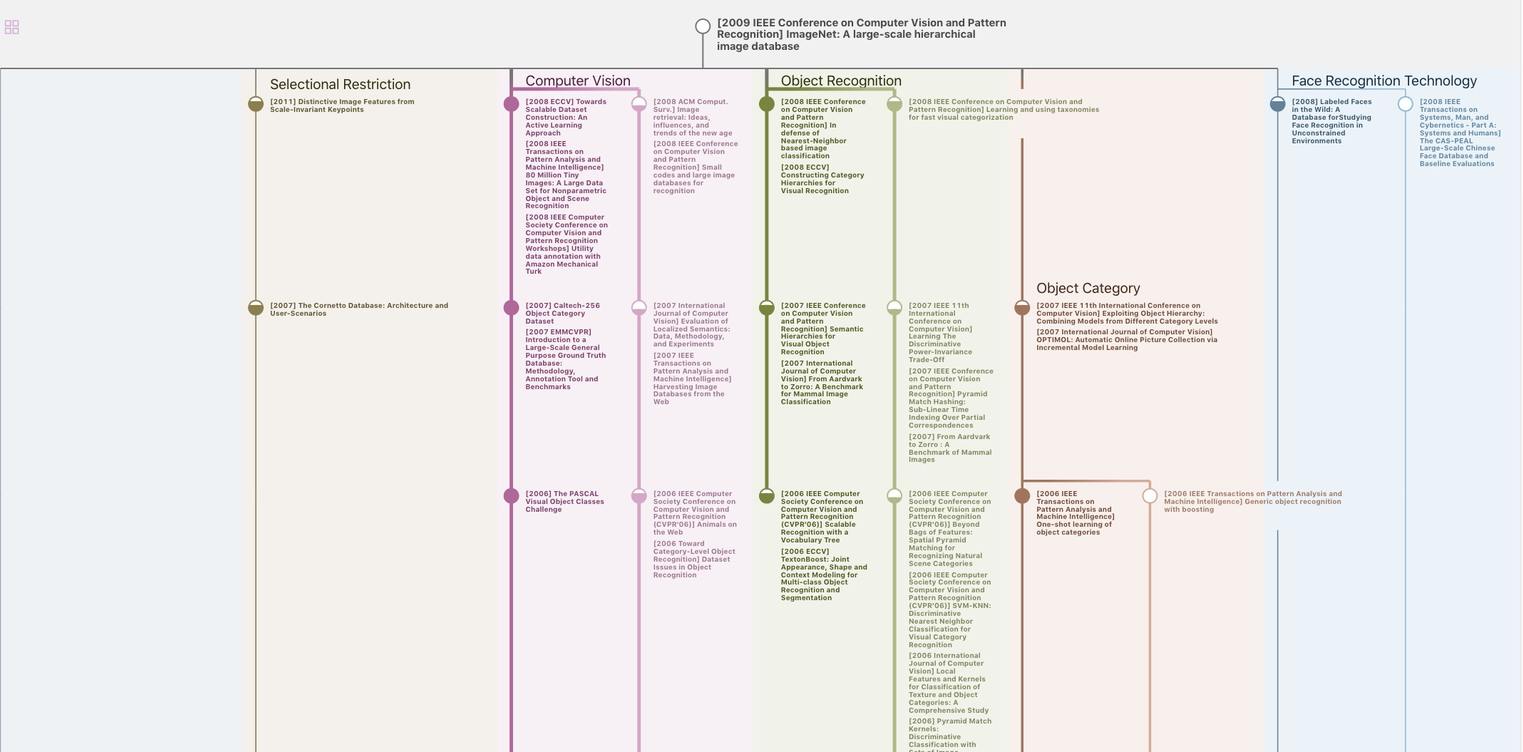
生成溯源树,研究论文发展脉络
Chat Paper
正在生成论文摘要