Ace-Sniper: Cloud-Edge Collaborative Scheduling Framework With DNN Inference Latency Modeling on Heterogeneous Devices
IEEE TRANSACTIONS ON COMPUTER-AIDED DESIGN OF INTEGRATED CIRCUITS AND SYSTEMS(2024)
摘要
The cloud-edge collaborative inference requires efficient scheduling of artificial intelligence (AI) tasks to the appropriate edge intelligence devices. Gls DNN inference latency has become a vital basis for improving scheduling efficiency. However, edge devices exhibit highly heterogeneous due to the differences in hardware architectures, computing power, etc. Meanwhile, the diverse deep neural networks (DNNs) are continuing to iterate over time. The diversity of devices and DNNs introduces high computational costs for measurement methods, while invasive prediction methods face significant development efforts and application limitations. In this article, we propose and develop Ace-Sniper, a scheduling framework with DNN inference latency modeling on heterogeneous devices. First, to address the device heterogeneity, a unified hardware resource modeling (HRM) is designed by considering the platforms as black-box functions that output feature vectors. Second, neural network similarity (NNS) is introduced for feature extraction of diverse and frequently iterated DNNs. Finally, with the results of HRM and NNS as input, the performance characterization network is designed to predict the latencies of the given unseen DNNs on heterogeneous devices, which can be combined into most time-based scheduling algorithms. Experimental results show that the average relative error of DNN inference latency prediction is 11.11%, and the prediction accuracy reaches 93.2%. Compared with the nontime-aware scheduling methods, the average waiting time for tasks is reduced by 82.95%, and the platform throughput is improved by 63% on average.
更多查看译文
关键词
Cloud-edge collaborative,hardware resource modeling (HRM),heterogeneous platform,inference latency modeling
AI 理解论文
溯源树
样例
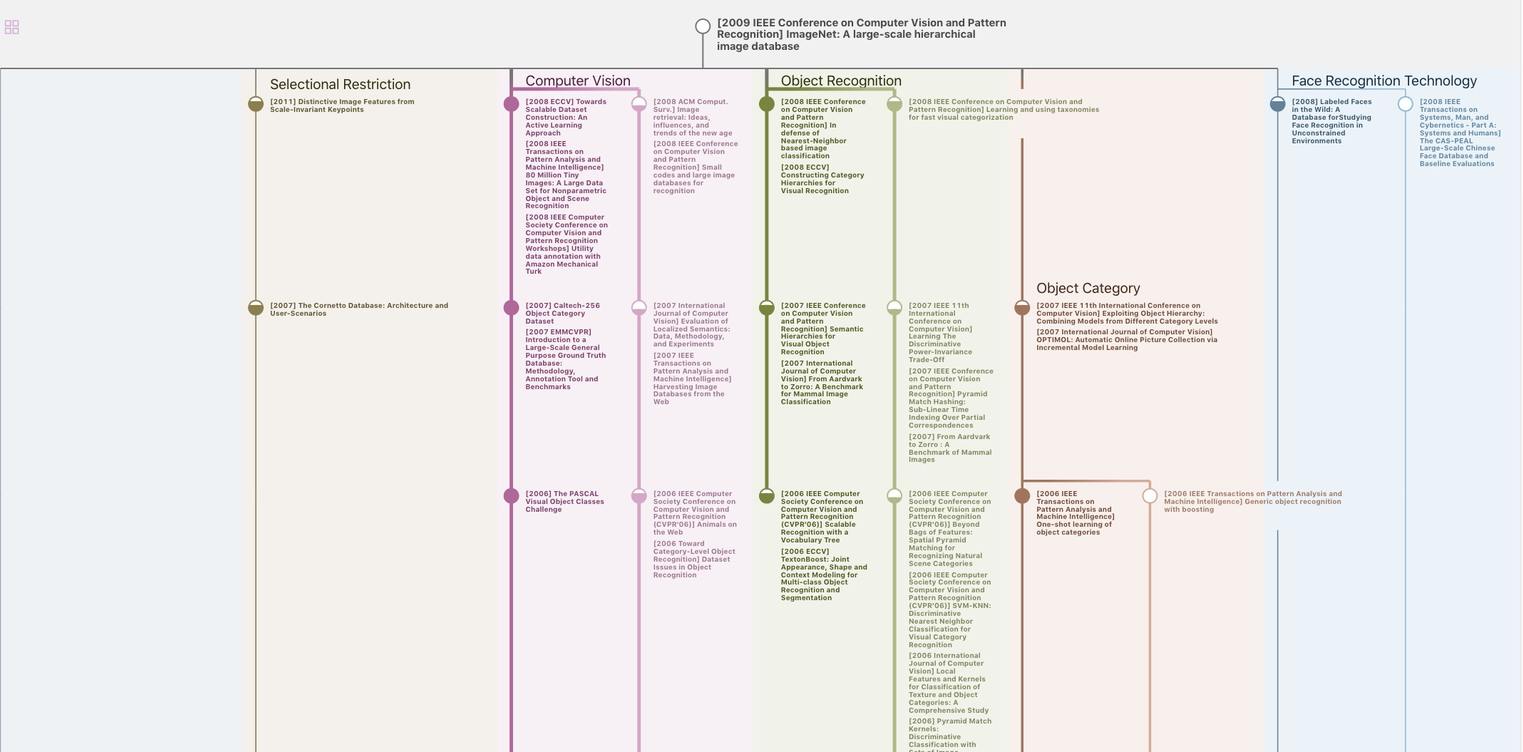
生成溯源树,研究论文发展脉络
Chat Paper
正在生成论文摘要