Distributions and Bootstrap for Data-based Stochastic Programming
Research Square (Research Square)(2023)
摘要
Abstract In the context of optimization under uncertainty, we consider various combinations of distribution estimation and resampling (bootstrap and bagging) for obtaining samples used to estimate a confidence interval for an optimality gap. This paper makes three experimental contributions to on-going research in data driven stochastic programming: a) most of the combinations of distribution estimation and resampling result in algorithms that have not been published before, b) within the algorithms, we describe innovations that improve performance, and c) we provide open-source software implementations of the algorithms. Among others, three important conclusions can be drawn: using a smoothed point estimate for the optimality gap for the center of the confidence interval is preferable to a purely empirical estimate, bagging generally performs better than bootstrap, and smoothed bagging sometimes performs better than bagging based directly on the data.
更多查看译文
关键词
stochastic programming,bootstrap,distributions,data-based
AI 理解论文
溯源树
样例
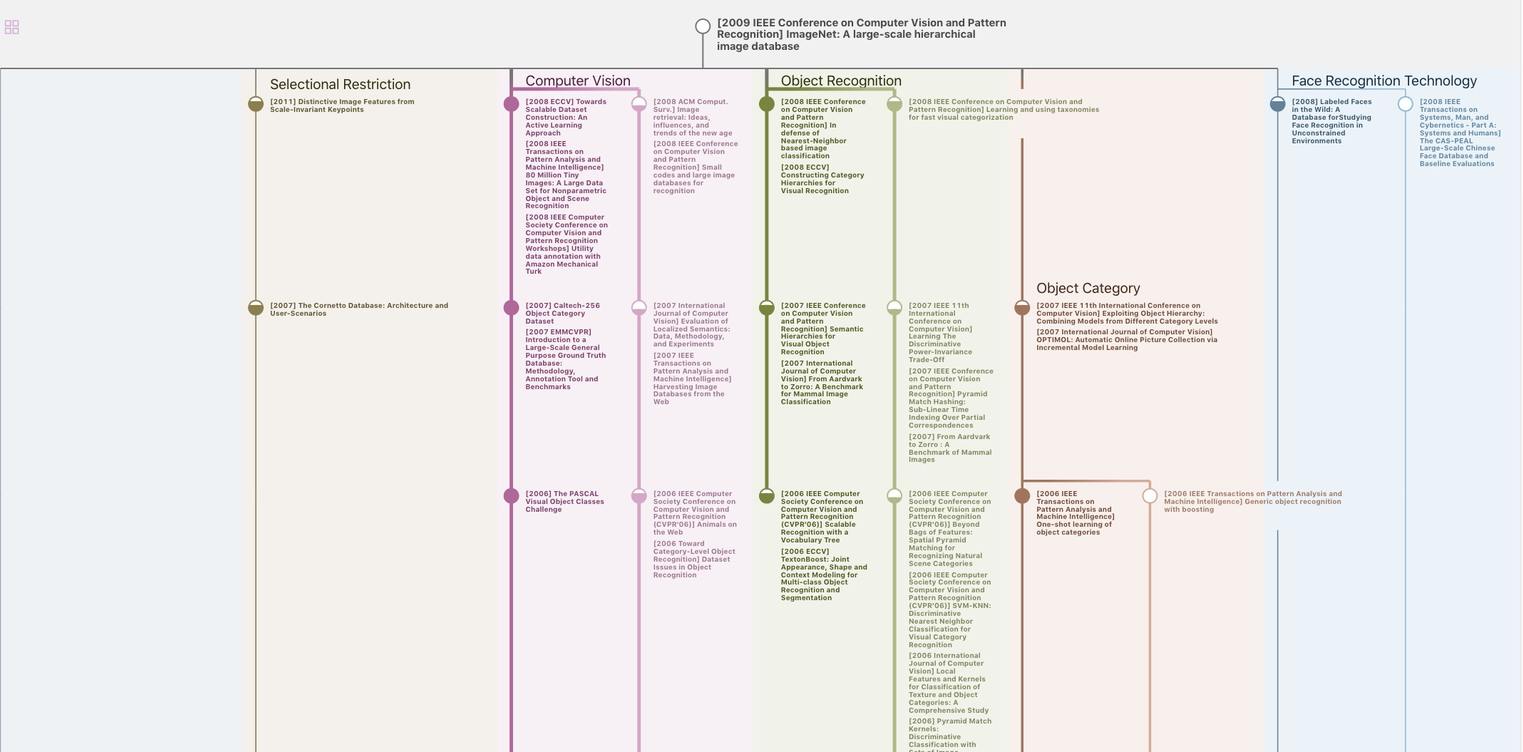
生成溯源树,研究论文发展脉络
Chat Paper
正在生成论文摘要